Adaptive DBSCAN LiDAR Point Cloud Clustering For Autonomous Driving Applications
2022 IEEE International Conference on Electro Information Technology (eIT)(2022)
摘要
LiDAR point cloud clustering is an essential part of a wide range of applications such as object detection, object recognition, and localization. In this paper, we focus on Density Based Spatial Clustering of Applications with Noise (DBSCAN) as a very promising and efficient algorithm to cluster LiDAR point cloud. However, it requires two tuning parameters that are manually specified based on prior knowledge of the domain. Furthermore, the selected tuning parameters cannot be modified once the clustering process begins. Environments in domains like robotics and autonomous driving are very dynamic, and hence, automated and adaptive selection of these parameters is preferred. In this work, we propose to estimate DBSCAN tuning parameters automatically based on a field of view division scheme, and points’ distances and densities within the division scheme. Furthermore, we adaptively estimate the tuning parameters for every point cloud frame separately. We evaluated the proposed method using the KITTI dataset, and the preliminary results show that the proposed method can achieve comparable results to the original DBSCAN without the need for manually selecting the parameters.
更多查看译文
关键词
LiDAR,Density-Based Clustering,DBSCAN,Object detection
AI 理解论文
溯源树
样例
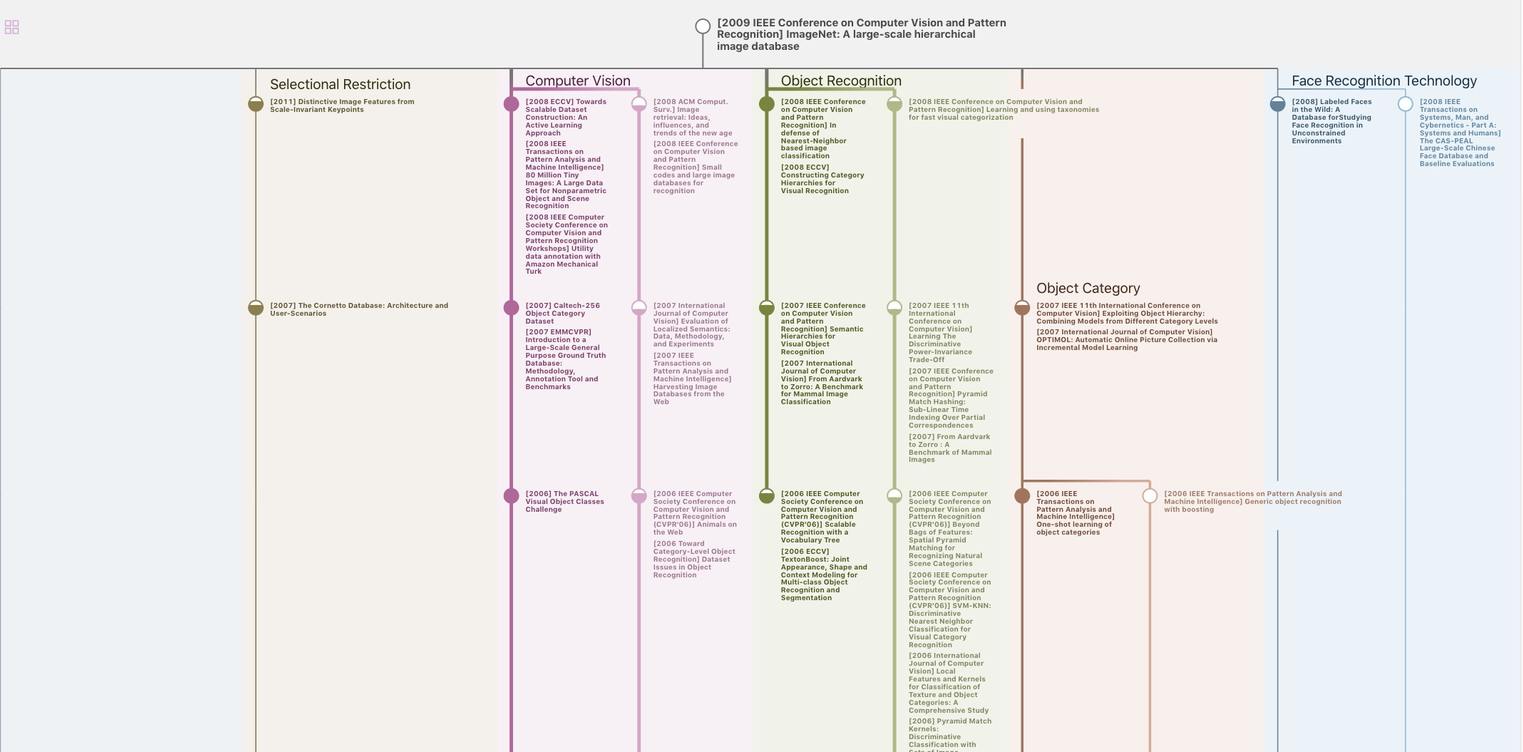
生成溯源树,研究论文发展脉络
Chat Paper
正在生成论文摘要