Establishment of early diagnosis models for cervical precancerous lesions using large-scale cervical cancer screening datasets
VIROLOGY JOURNAL(2022)
摘要
Background Human papilloma virus (HPV) DNA test was applied in cervical cancer screening as an effective cancer prevention strategy. The viral load of HPV generated by different assays attracted increasing attention on its potential value in disease diagnosis and progression discovery. Methods In this study, three HPV testing datasets were assessed and compared, including Hybrid Capture 2 (n = 31,954), Aptima HPV E6E7 (n = 3269) and HPV Cobas 4800 (n = 13,342). Logistic regression models for diagnosing early cervical lesions of the three datasets were established and compared. The best variable factor combination (VL + BV) and dataset (HC2) were used for the establishment of six machine learning models. Models were evaluated and compared, and the best-performed model was validated. Results Our results show that viral load value was significantly correlated with cervical lesion stages in all three data sets. Viral Load and Bacterial Vaginosis were the best variable factor combination for logistic regression model establishment, and models based on the HC2 dataset performed best compared with the other two datasets. Machine learning method Xgboost generated the highest AUC value of models, which were 0.915, 0.9529, 0.9557, 0.9614 for diagnosing ASCUS higher, ASC-H higher, LSIL higher, and HSIL higher staged cervical lesions, indicating the acceptable accuracy of the selected diagnostic model. Conclusions Our study demonstrates that HPV viral load and BV status were significantly associated with the early stages of cervical lesions. The best-performed models can serve as a useful tool to help diagnose cervical lesions early.
更多查看译文
关键词
Human papillomavirus,Cervical cancer,Viral load,Logistic regression,Machine learning,Diagnostic model
AI 理解论文
溯源树
样例
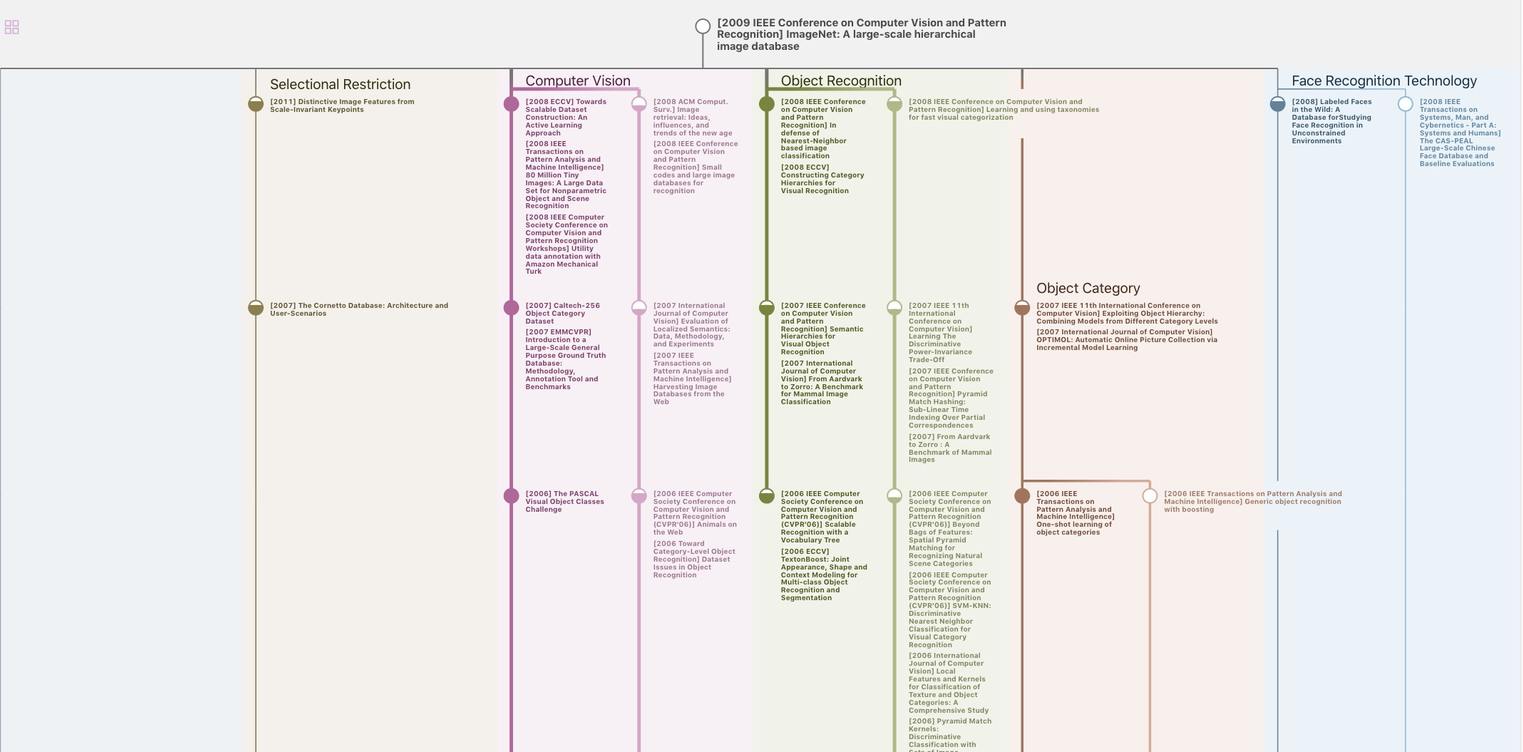
生成溯源树,研究论文发展脉络
Chat Paper
正在生成论文摘要