Decentralized Anomaly Detection via Deep Multi-Agent Reinforcement Learning
2022 58th Annual Allerton Conference on Communication, Control, and Computing (Allerton)(2022)
摘要
We consider a decentralized anomaly detection problem, where multiple agents collaborate to localize a single anomalous process among a finite number
$M$
of processes. At each time, a subset of the processes can be observed by each agent, and the observations from each chosen process follow two different distributions, depending on whether the process is normal or abnormal. The communication channel between agents is rate-limited. The objective is a sequential search strategy that minimizes the Bayes risk, consisting of the sampling cost, and the joint terminal cost among the agents. This problem generalizes previous studies that considered anomaly detection by a single detector. We develop a novel algorithm based on deep multi-agent reinforcement learning optimization to minimize the Bayes risk. Numerical experiments demonstrate the ability of the algorithm to learn good policies in this challenging problem, and improve the single-agent performance by applying the proposed multi-agent collaborative learning method.
更多查看译文
关键词
deep multiagent reinforcement learning,decentralized anomaly detection,communication channel,sequential search strategy,Bayes risk minimization,sampling cost,joint terminal cost,single-agent performance,multiagent collaborative learning
AI 理解论文
溯源树
样例
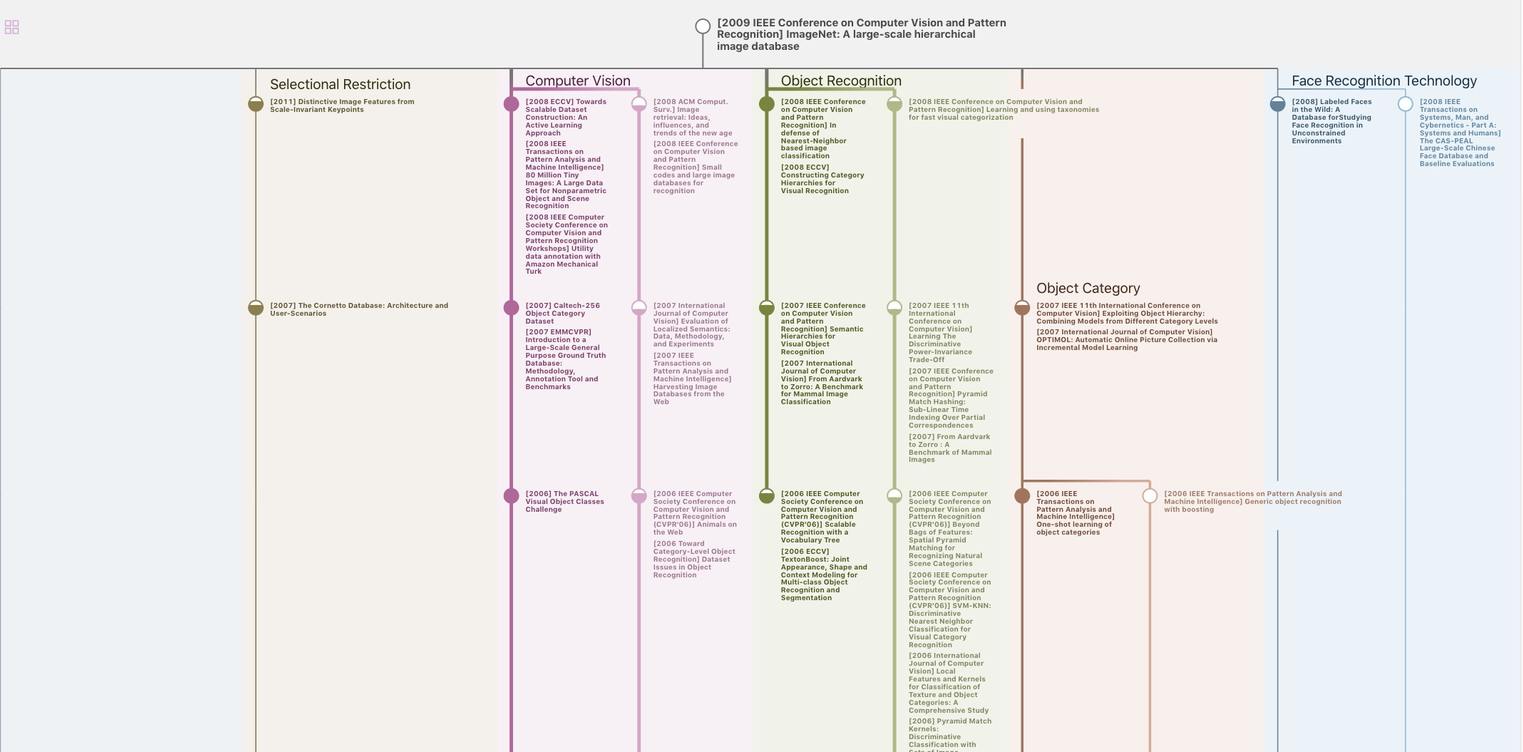
生成溯源树,研究论文发展脉络
Chat Paper
正在生成论文摘要