Multiagent Reinforcement Learning With Heterogeneous Graph Attention Network
IEEE TRANSACTIONS ON NEURAL NETWORKS AND LEARNING SYSTEMS(2023)
摘要
Most recent research on multiagent reinforcement learning (MARL) has explored how to deploy cooperative policies for homogeneous agents. However, realistic multiagent environments may contain heterogeneous agents that have different attributes or tasks. The heterogeneity of the agents and the diversity of relationships cause the learning of policy excessively tough. To tackle this difficulty, we present a novel method that employs a heterogeneous graph attention network to model the relationships between heterogeneous agents. The proposed method can generate an integrated feature representation for each agent by hierarchically aggregating latent feature information of neighbor agents, with the importance of the agent level and the relationship level being entirely considered. The method is agnostic to specific MARL methods and can be flexibly integrated with diverse value decomposition methods. We conduct experiments in predator–prey and StarCraft Multiagent Challenge (SMAC) environments, and the empirical results demonstrate that the performance of our method is superior to existing methods in several heterogeneous scenarios.
更多查看译文
关键词
Reinforcement learning, Multi-agent systems, Aggregates, Task analysis, Scalability, Marine vehicles, Learning systems, Graph attention network, heterogeneous agents, multiagent reinforcement learning (MARL), relationship-level attention
AI 理解论文
溯源树
样例
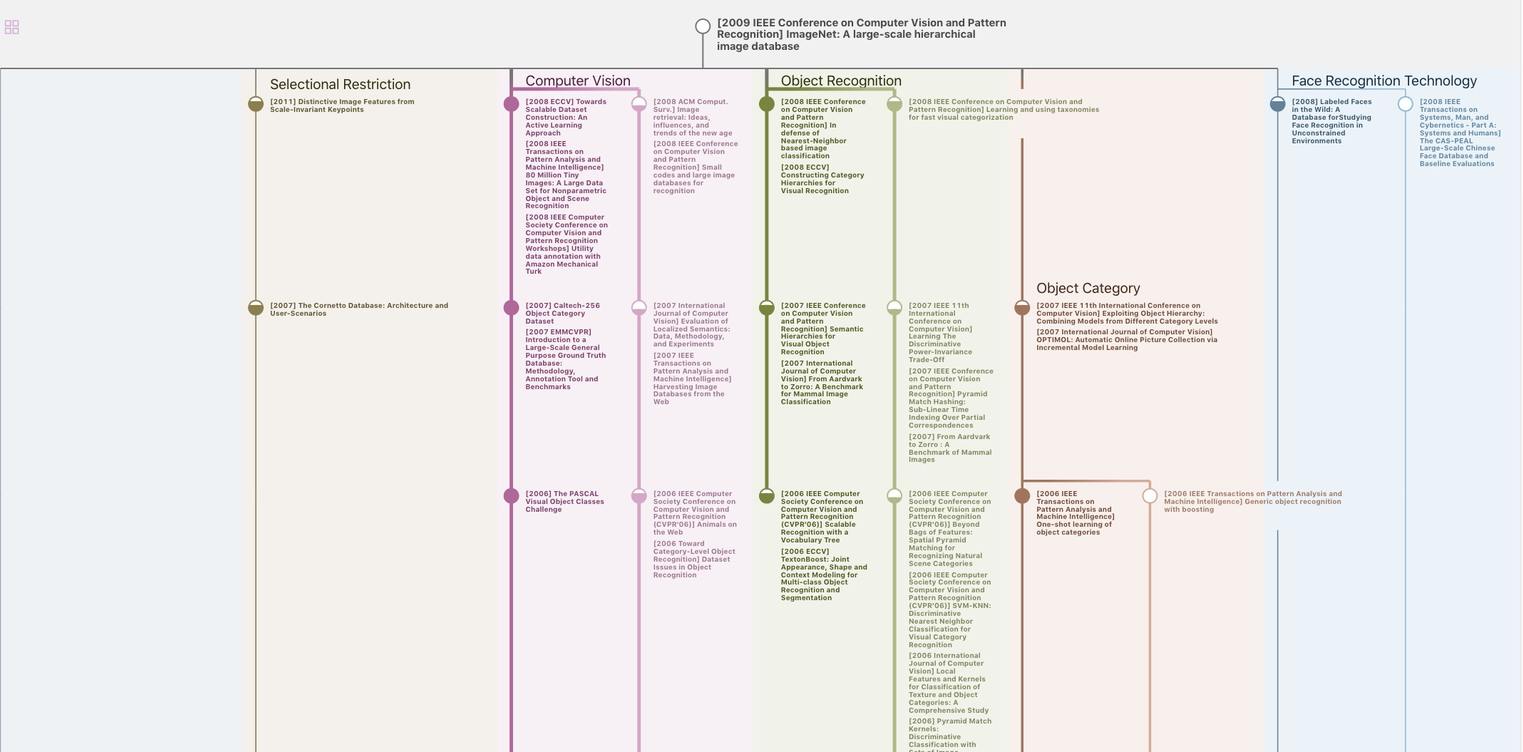
生成溯源树,研究论文发展脉络
Chat Paper
正在生成论文摘要