Machine learning-driven design of promising perovskites for photovoltaic applications: A review
Surfaces and Interfaces(2022)
Abstract
Metal halide perovskite solar cells (PSCs) have become popular photovoltaic devices due to their high power conversion efficiencies (PCEs), low-cost raw materials and simple production processes. However, metal halide perovskites decompose rapidly in the presence of humid air, light illumination and heat, which is unfavorable for the commercial applications of PSCs. Recently, machine learning (ML) has emerged as a powerful approach to screen novel perovskite materials with high stability and excellent optoelectronic properties for photovoltaic cells. Herein, we introduce the latest progress of ML methods for assisting the discovery of promising three-dimensional (3D) perovskites and two-dimensional (2D) layered perovskites as light-absorbing materials. The ML models to predict high-efficient PSCs are also reviewed. In the end, we discuss the advantages and challenges of ML methods as well as their possible future prospects. We expect that this review can provide some guidance for the future ML-driven design of promising perovskite materials for photovoltaics.
MoreTranslated text
Key words
Perovskite solar cell,Machine learning,Bandgap,Power conversion efficiency,Stability
AI Read Science
Must-Reading Tree
Example
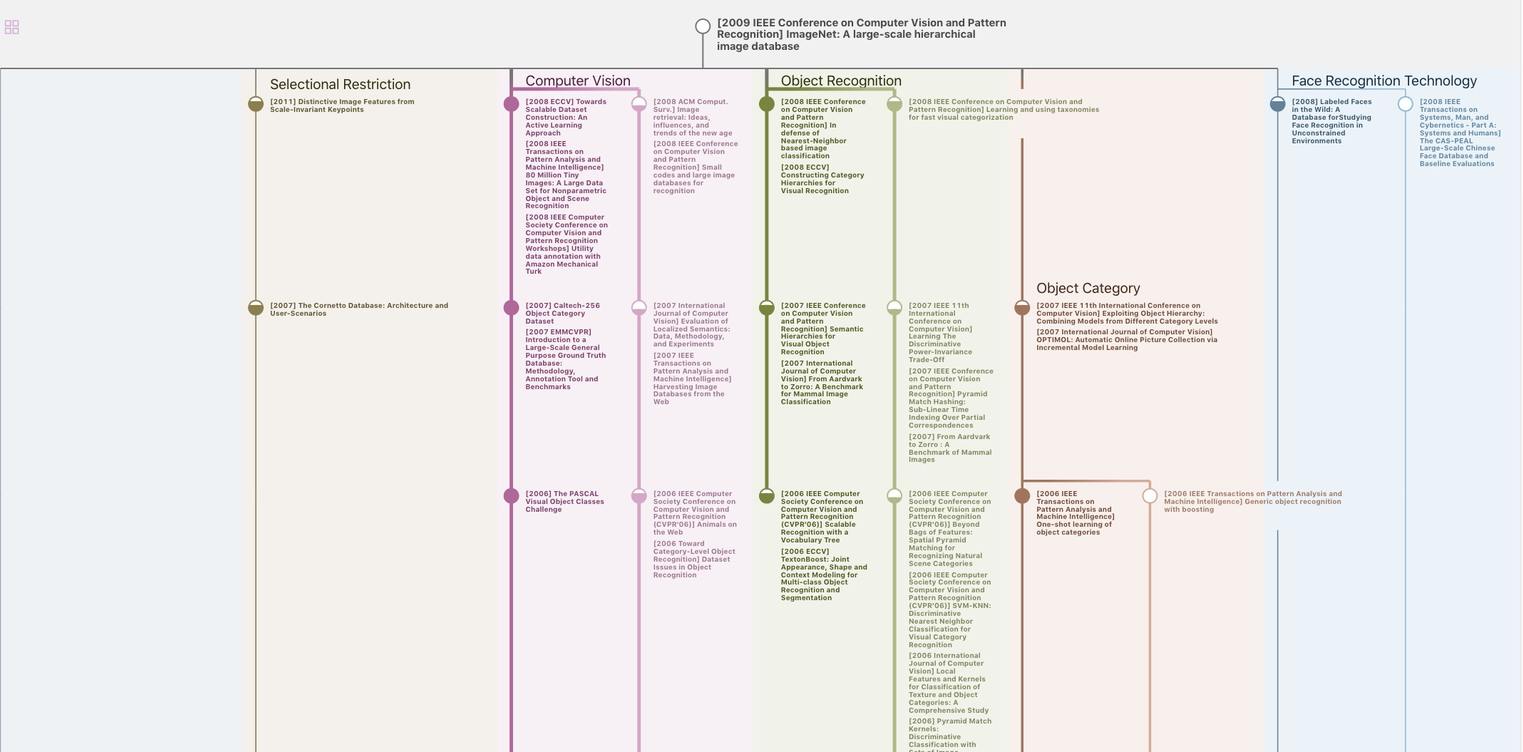
Generate MRT to find the research sequence of this paper
Chat Paper
Summary is being generated by the instructions you defined