Learning to school in dense configurations with multi-agent deep reinforcement learning.
Bioinspiration & biomimetics(2022)
摘要
Fish are observed to school in different configurations. However, how and why fish maintain a stable schooling formation still remains unclear. This work presents a numerical study of the dense schooling of two free swimmers by a hybrid method of the multi-agent deep reinforcement learning and the immersed boundary-lattice Boltzmann method. Active control policies are developed by synchronously training the leader to swim at a given speed and orientation and the follower to hold close proximity to the leader. After training, the swimmers could resist the strong hydrodynamic force to remain in stable formations and meantime swim in desired path, only by their tail-beat flapping. The tail movement of the swimmers in the stable formations are irregular and asymmetrical, indicating the swimmers are carefully adjusting their body-kinematics to balance the hydrodynamic force. In addition, a significant decrease in the mean amplitude and the cost of transport is found for the followers, indicating these swimmers could maintain the swimming speed with less efforts. The results also show that the side-by-side formation is hydrodynamically more stable but energetically less efficient than other configurations, while the full-body staggered formation is energetically more efficient as a whole.
更多查看译文
关键词
collective motion,fish schooling,immersed boundary-lattice Boltzmann method,multi-agent deep reinforcement learning,side-by-side swimming,staggered swimming
AI 理解论文
溯源树
样例
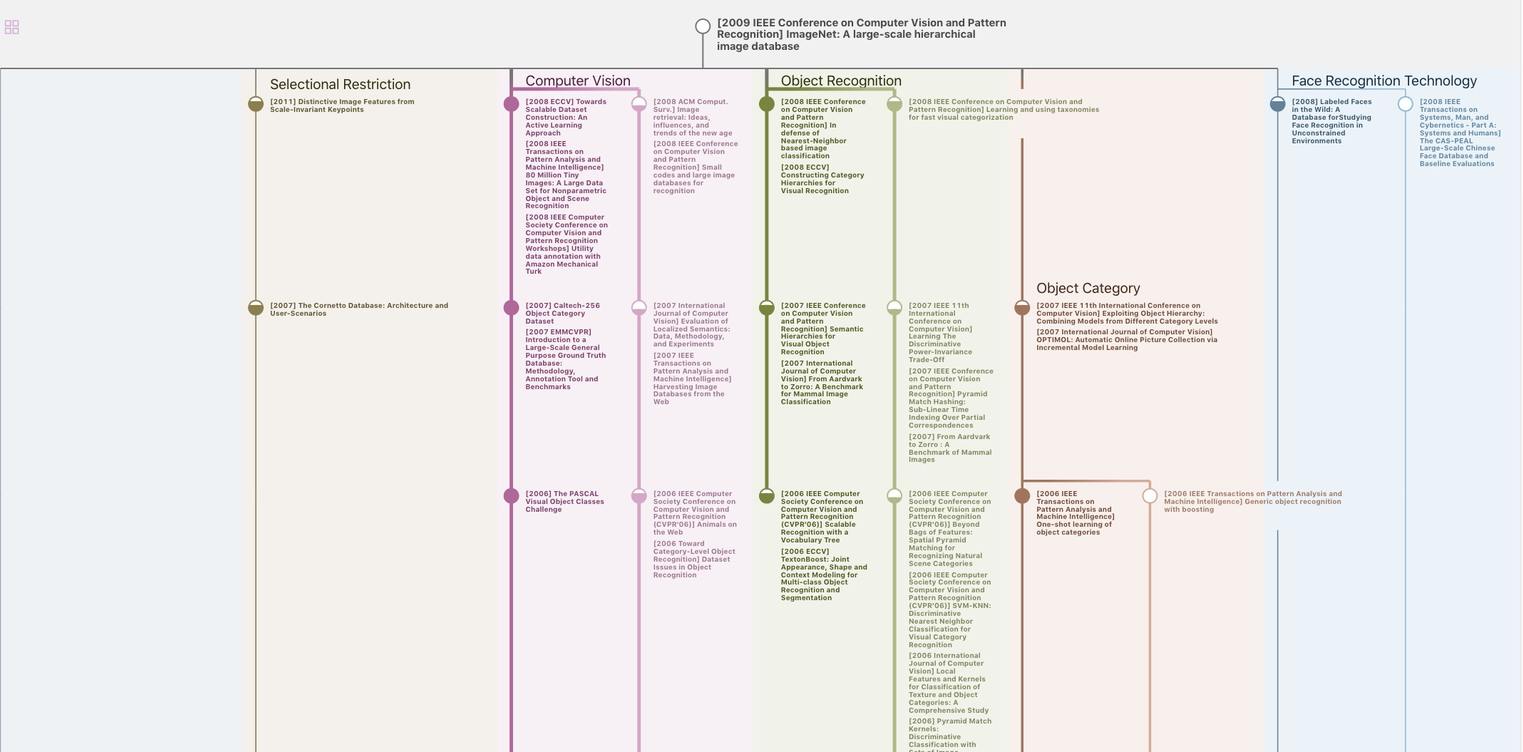
生成溯源树,研究论文发展脉络
Chat Paper
正在生成论文摘要