3D Multi-Object Tracking Based on Dual-Tracker and D-S Evidence Theory
IEEE Transactions on Intelligent Vehicles(2023)
摘要
Most of the current self-driving cars are equipped with a variety of sensors (such as lidar, camera), and it has become a trend to integrate multi-sensor information to achieve 3D multi-object tracking (MOT). In addition, unlike the perfect experimental conditions on public datasets, cars on real roads may experience a 3D sensor failure due to weather, mutual interference, etc. In this paper, we propose a dual-tracker-based 3D MOT system, which fuses 2D and 3D information to track objects and can still ensure good tracking accuracy when the 3D sensor fails in a single frame or multiple consecutive frames. Among them, two internal trackers complete the data association of 3D and 2D information, respectively. However, the outputs of the two internal trackers may conflict. First, we calculate the degree of association of potential matched pairs from the features extracted by each sensor, and then normalize it into the mass function of D-S evidence theory. Finally, we combine the evidence to obtain the final matched pairs of detection instances and track estimates. We do extensive experiments on the KITTI MOT dataset and simulate sensor failure scenarios by ignoring the output of the 3D detector. Using the latest evaluation measure for comparison, the results show that our method outperforms other advanced open-source 3D MOTs in a variety of scenarios.
更多查看译文
关键词
3D multi-object tracking,D-S evidence theory,multi-sensor fusion
AI 理解论文
溯源树
样例
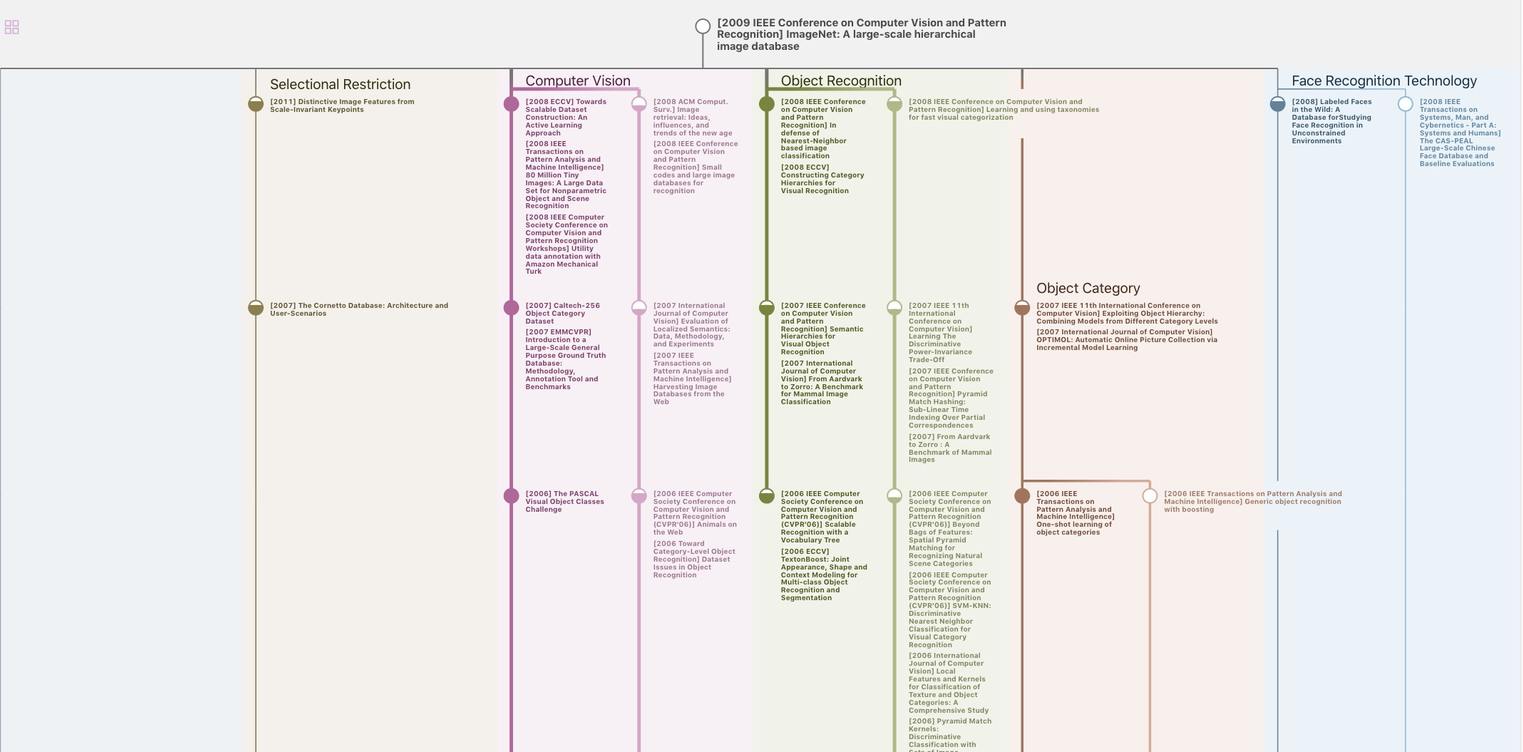
生成溯源树,研究论文发展脉络
Chat Paper
正在生成论文摘要