Improving Deep Learning Based Second-Order Side-Channel Analysis With Bilinear CNN.
IEEE Trans. Inf. Forensics Secur.(2022)
摘要
In recent years, deep learning techniques have received significant attention in the side-channel community due to their state-of-the-art performance in profiled attacks against embedded devices. Compared with template attacks, deep learning-based attacks can deal with the high dimensionality of trace and misalignment without pre-processing. However, the performance of attacks is very sensitive to the network architecture, especially when considering masking countermeasures. Although previous works have shown the potential of neural networks to break the first-order masking, the inner-working of how the network combines the leakage of mask and masked value is still unclear and could be suboptimal. To reduce this gap, we propose to embed product combination, which has been proved to be the best combination function in noisy situations, into the design of neural networks. To this end, we introduce a bilinear convolutional neural network (in short, B-CNN) for efficient profiled attacks against the widely used masking countermeasure. In order to interpret the inner-working and decision-making of B-CNN, we propose a new visualization tool called layer-wise correlation that can reveal the points of interest and help to understand the combination of leakages. We evaluate our networks on several public datasets, e.g., ASCAD and CHES CTF 2018. The results indicate that B-CNN converges significantly faster than classic CNN models, even using a very limited number of profiling traces (e.g., 8000 profiling traces for the ASCAD dataset). Moreover, our networks perform even systematically better (w.r.t. the number of attack traces) than the current state-of-the-art results on all the investigated datasets.
更多查看译文
关键词
Neural networks,Convolutional neural networks,Deep learning,Visualization,Task analysis,Feature extraction,Decision making,Side-channel attacks,profiled attacks,deep learning,masking countermeasures
AI 理解论文
溯源树
样例
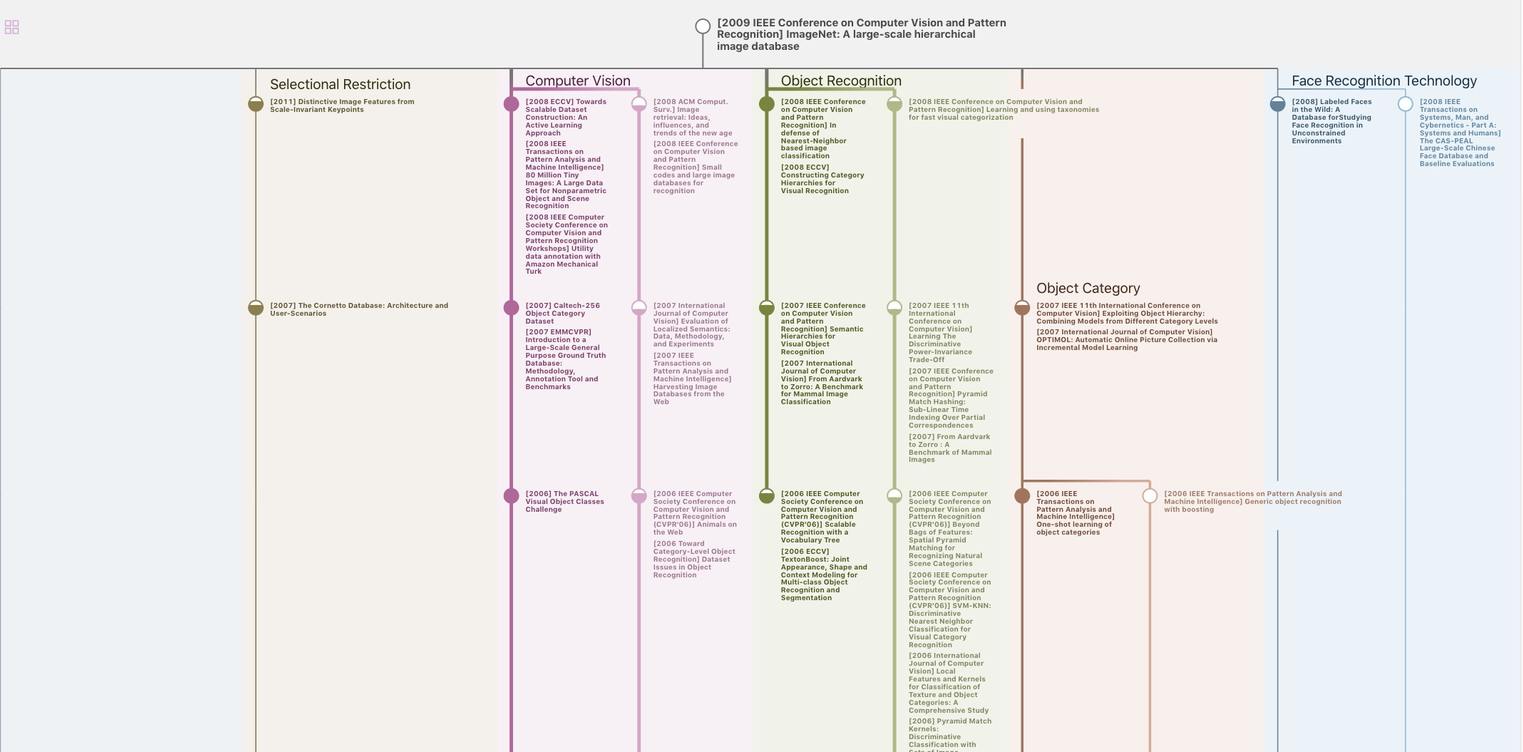
生成溯源树,研究论文发展脉络
Chat Paper
正在生成论文摘要