Forgetful Active Learning with Switch Events: Efficient Sampling for Out-of-Distribution Data
2022 IEEE INTERNATIONAL CONFERENCE ON IMAGE PROCESSING, ICIP(2022)
摘要
This paper considers deep out-of-distribution active learning. In practice, fully trained neural networks interact randomly with out-of-distribution (OOD) inputs and map aberrant samples randomly within the model representation space. Since data representations are direct manifestations of the training distribution, the data selection process plays a crucial role in outlier robustness. For paradigms such as active learning, this is especially challenging since protocols must not only improve performance on the training distribution most effectively but further render a robust representation space. However, existing strategies directly base the data selection on the data representation of the unlabeled data which is random for OOD samples by definition. For this purpose, we introduce forgetful active learning with switch events (FALSE) - a novel active learning protocol for out-of-distribution active learning. Instead of defining sample importance on the data representation directly, we formulate "informativeness" with learning difficulty during training. Specifically, we approximate how often the network "forgets" unlabeled samples and query the most "forgotten" samples for annotation. We report up to 4.5\% accuracy improvements in over 270 experiments, including four commonly used protocols, two OOD benchmarks, one in-distribution benchmark, and three different architectures.
更多查看译文
关键词
Active Learning, Forgetting Events, Out-of-Distribution
AI 理解论文
溯源树
样例
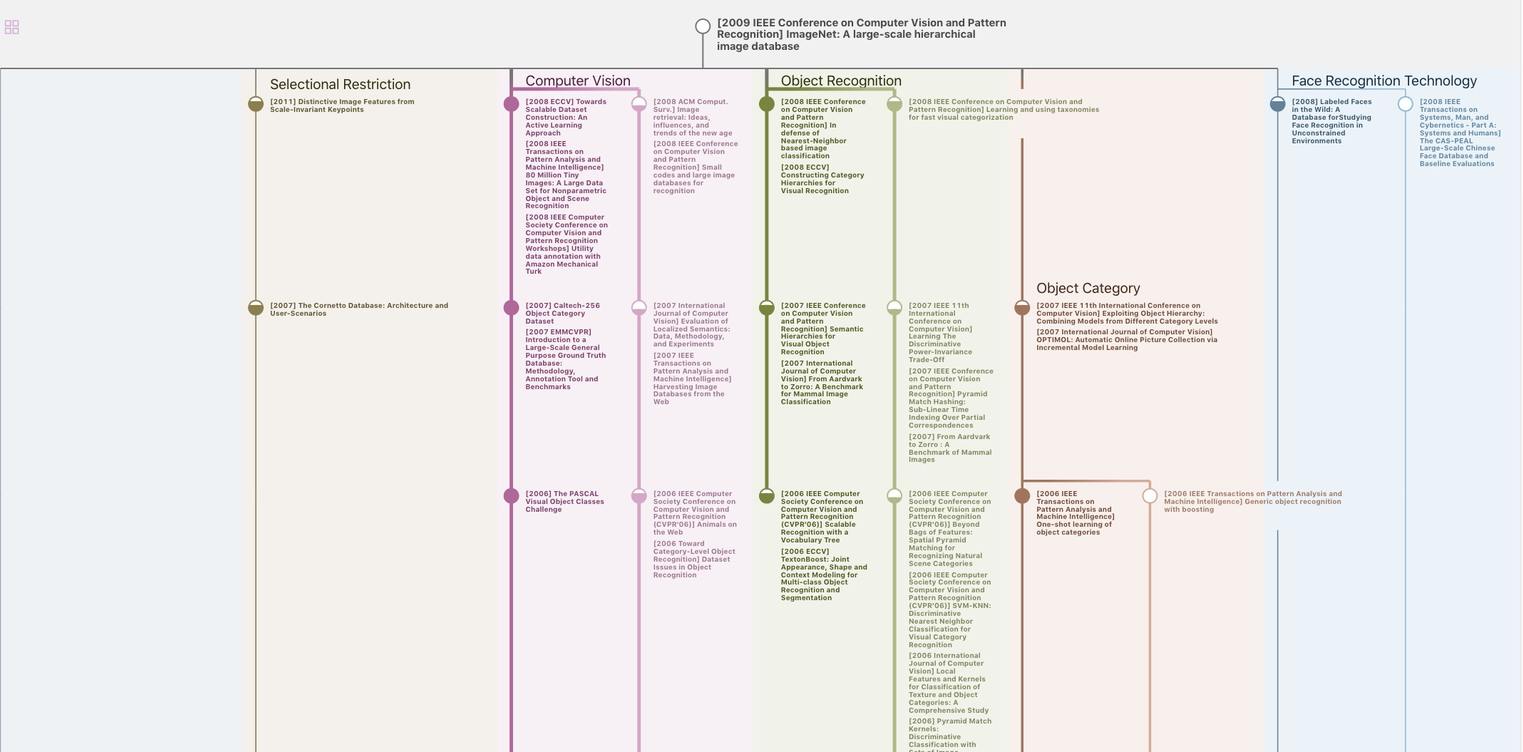
生成溯源树,研究论文发展脉络
Chat Paper
正在生成论文摘要