Spectrum Sensing in Cognitive Radio Using CNN-RNN and Transfer Learning
IEEE ACCESS(2022)
摘要
Cognitive radio has been proposed to improve spectrum utilization in wireless communication. Spectrum sensing is an essential component of cognitive radio. The traditional methods of spectrum sensing are based on feature extraction of a received signal at a given point. The development in artificial intelligence and deep learning have given an opportunity to improve the accuracy of spectrum sensing by using cooperative spectrum sensing and analyzing the radio scene. This research proposed a hybrid model of convolution and recurrent neural network for spectrum sensing. The research further enhances the accuracy of sensing for low SNR signals through transfer learning. The results of modelling show improvement in spectrum sensing using CNN-RNN compared to other models studied in this field. The complexity of an algorithm is analyzed to show an improvement in the performance of the algorithm.
更多查看译文
关键词
Sensors,Convolutional neural networks,Transfer learning,Deep learning,Cognitive radio,Signal to noise ratio,Mathematical models,Neural networks,Recurrent neural networks,Spectrum sensing,cognitive radio,deep neural network,convolutional neural network,recurrent neural network
AI 理解论文
溯源树
样例
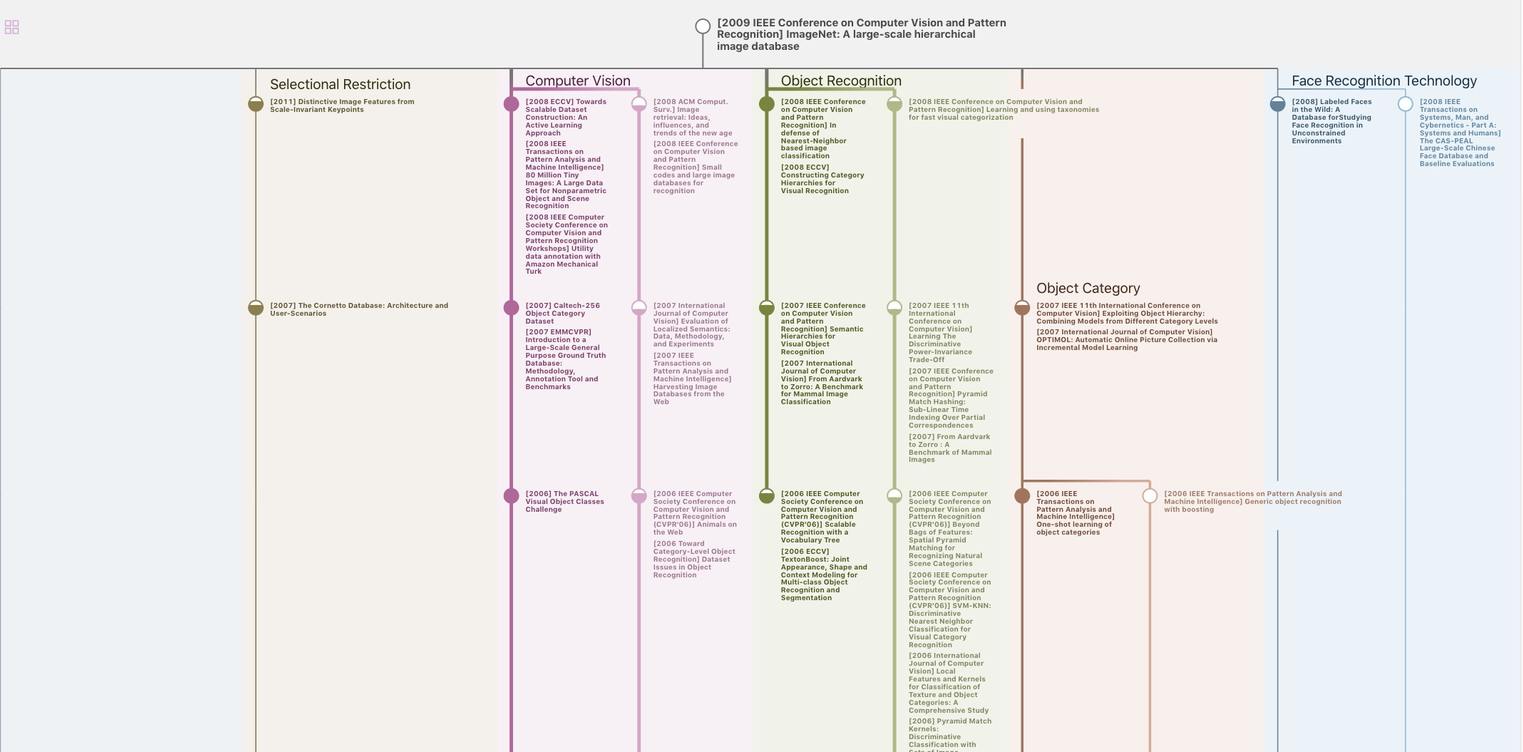
生成溯源树,研究论文发展脉络
Chat Paper
正在生成论文摘要