Pattern Matching and Information-Aware Between Reviews and Ratings for Recommendation.
Pacific Rim International Conference on Artificial Intelligence (PRICAI)(2022)
摘要
Real recommendation scenarios have diverse and multi-modal features, which are often high-dimensional, sparse, and heterogeneous, making it difficult to learn. To improve model performance, many studies jointly model the semantic information of user and item reviews. However, due to the inherent changes in natural language processing, it has become a challenge to more accurately model the semantic interaction and matching relationship between user and item reviews. In addition, most of the previous work directly connects text representations and numerical features without considering their natural gap. In view of this, we propose a novel Pattern Matching and Information-aware Network (MIAN). Specifically, we design a matching network composed of global matching and specific matching, which is used to model the matching information between user and item reviews. The specific matching module uses the item description as a bridge to assist in modeling more fine-grained matching relationships. In addition, we construct an information perception layer to align the information between reviews and numerical features. Furthermore, we adopt a joint learning manner for better model training by employing a matching task fusion, which benefits from the prior matching knowledge. Comprehensive experiments on five real-world datasets show that MIAN outperforms the state-of-the-art methods.
更多查看译文
关键词
Pattern matching,Information perception,Attention mechanism,Review-based recommendation
AI 理解论文
溯源树
样例
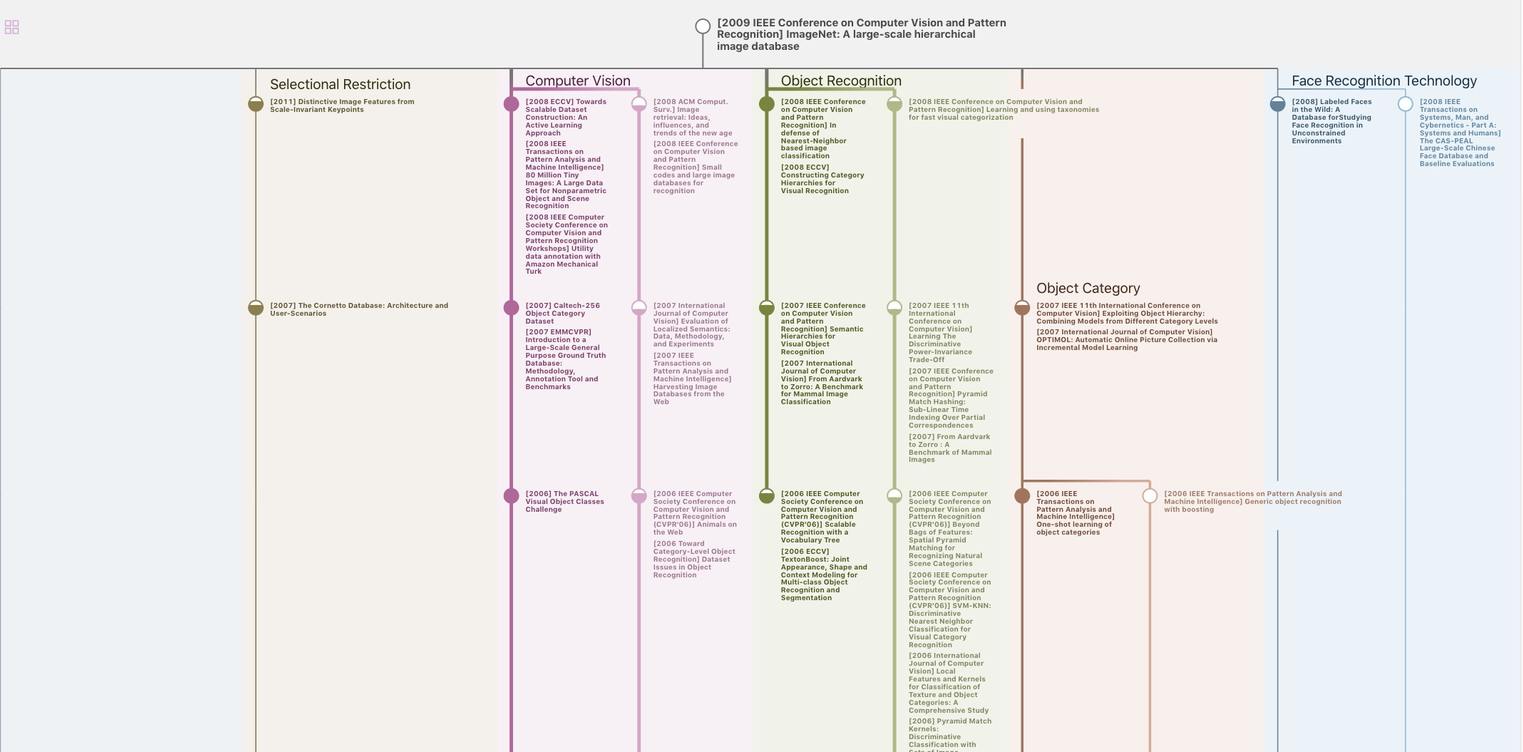
生成溯源树,研究论文发展脉络
Chat Paper
正在生成论文摘要