Speeding up NAS with Adaptive Subset Selection
arxiv(2022)
摘要
A majority of recent developments in neural architecture search (NAS) have been aimed at decreasing the computational cost of various techniques without affecting their final performance. Towards this goal, several low-fidelity and performance prediction methods have been considered, including those that train only on subsets of the training data. In this work, we present an adaptive subset selection approach to NAS and present it as complementary to state-of-the-art NAS approaches. We uncover a natural connection between one-shot NAS algorithms and adaptive subset selection and devise an algorithm that makes use of state-of-the-art techniques from both areas. We use these techniques to substantially reduce the runtime of DARTS-PT (a leading one-shot NAS algorithm), as well as BOHB and DEHB (leading multifidelity optimization algorithms), without sacrificing accuracy. Our results are consistent across multiple datasets, and towards full reproducibility, we release our code at https: //anonymous.4open.science/r/SubsetSelection NAS-B132.
更多查看译文
关键词
nas,selection
AI 理解论文
溯源树
样例
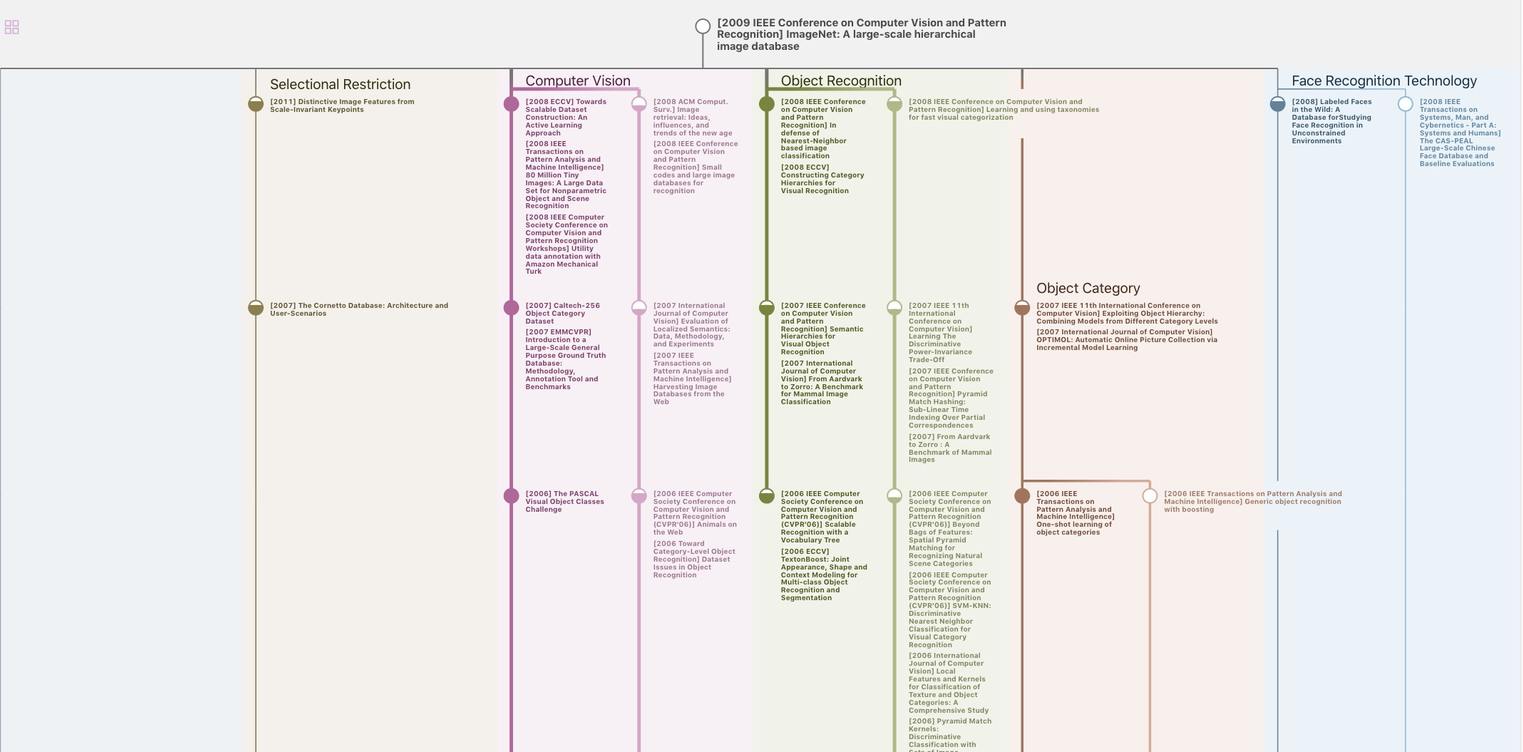
生成溯源树,研究论文发展脉络
Chat Paper
正在生成论文摘要