Probability Prediction Approach of Fatigue Failure for the Subsea Wellhead Using Bayesian Regularization Artificial Neural Network
JOURNAL OF MARINE SCIENCE AND ENGINEERING(2022)
摘要
The subsea wellhead (SW) system is a crucial connection between blowout preventors (BOPs) and subsea oil and gas wells. Excited by cyclical fatigue dynamic loadings, the SW is prone to fatigue failure, which would lead to the loss of well integrity and catastrophic accidents. Based on the Bayesian Regularization Artificial Neuron Network (BRANN), this paper proposes an efficient probability approach to predict the fatigue failure probability of SW during its entire life. In the proposed method, the BRANN fatigue damage (BRANN-FD) model reflecting the non-linear relationship between the input and output data was developed by the limited fatigue damage analysis data, which was utilized to generate thousands of non-numerical fatigue damage data of SW rapidly. Combining parametric and non-parametric estimation methods, the probability density function (PDF) of SW fatigue damage was determined to calculate the accumulation fatigue damage during service life. Using the logistic regression, the fatigue failure probability of SW was predicted. The application of the proposed approach was demonstrated by a case study. The results illustrated that the fatigue damage of SW would be viewed as obeying the Lognormal distribution, which could be used to obtain the accumulation fatigue damage in operation conveniently. Furthermore, the fatigue failure probability of SW nonlinearly increased with the increment in the accumulation fatigue damage of SW, which could be helpful to ensure the operation safety of SW in deepwater oil and gas development, especially for aged wellhead.
更多查看译文
关键词
subsea wellhead,fatigue damage,Bayesian Regularization Artificial Neuron Network,probability density function,fatigue failure
AI 理解论文
溯源树
样例
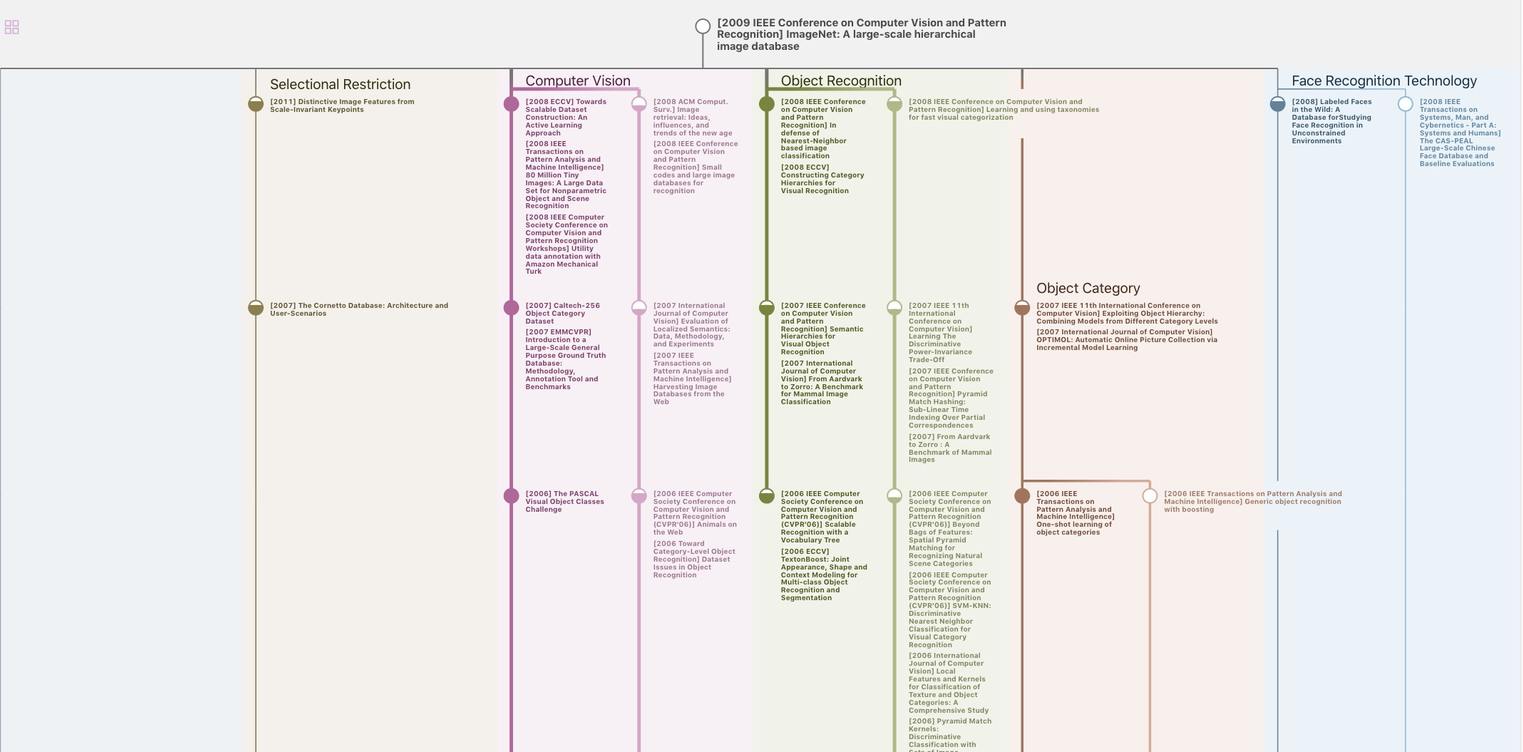
生成溯源树,研究论文发展脉络
Chat Paper
正在生成论文摘要