A discrete clonal selection algorithm for filter-based local feature selection
2022 IEEE Congress on Evolutionary Computation (CEC)(2022)
摘要
Feature selection algorithms aim to improve the per-formance of machine learning algorithms by removing irrelevant and redundant features. Various feature selection algorithms have been proposed, but most of them select a global feature subset for characterizing the entire sample space. In contrast, this study proposes an efficient discrete clonal selection algorithm for local feature selection called DCSA-LFS with three features: (1) local sample behaviors are considered, and a local clustering-based evaluation criterion is used to select a distinct optimized feature subset for each different sample region; (2) an improved discrete clonal selection algorithm is proposed, which uses a differential evolution-based mutation operator to enhance the search capability of clonal selection algorithms; and (3) a two-part antibody representation is adopted to automatically adjust the weight-related parameter. Experimental results on twelve UCI datasets show that DCSA-LFS is competitive with traditional filter-based feature selection algorithms and a clonal selection algorithm-based local feature selection algorithm.
更多查看译文
关键词
local feature selection,clonal selection algorithm,artificial immune system,dimensionality reduction
AI 理解论文
溯源树
样例
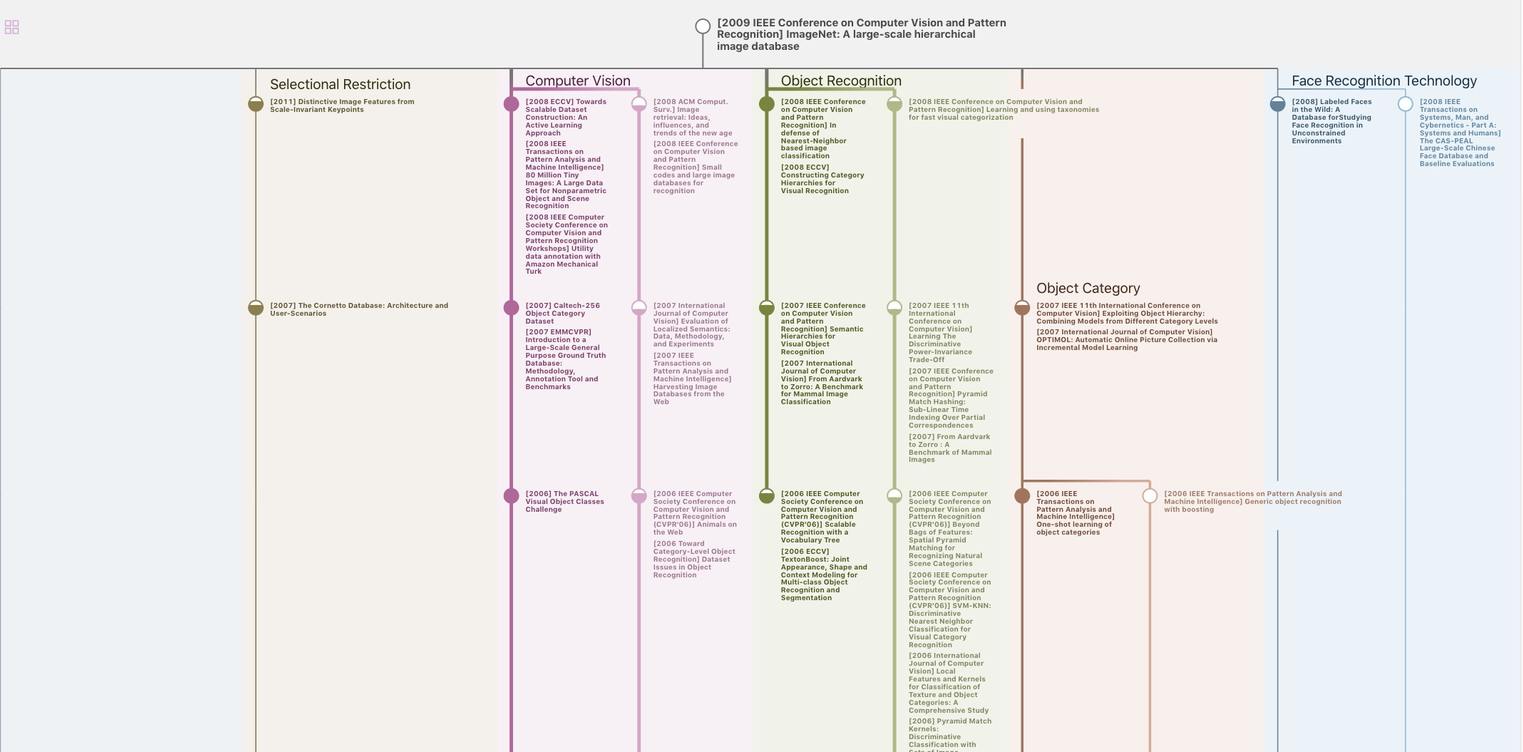
生成溯源树,研究论文发展脉络
Chat Paper
正在生成论文摘要