Globally Scalable Approach to Estimate Net Ecosystem Exchange Based on Remote Sensing, Meteorological Data, and Direct Measurements of Eddy Covariance Sites
REMOTE SENSING(2022)
摘要
Despite a rapid development of Nature-Based Solutions (NBS) for carbon removal in recent years, the methods for evaluating NBS still have certain gaps. We propose an approach based on a combination of remote sensing data and meteorological variables to reconstruct the spatiotemporal variation of net ecosystem exchange from eddy-covariance stations. A Lagrangian particle dispersion model was used for upscaling satellite images and flux towers. We trained data-driven models based on kernel methods separately for each selected land-cover class. The results suggest that the proposed approach to quantifying carbon exchange on a medium-to-large scale by blending eddy covariance flux data with moderate resolution satellite and weather data provides a set of key advantages over previously deployed methods: (1) scalability, achieved via the validation design based on a separate set of eddy covariance stations; (2) high spatial and temporal resolution thanks to the use of Landsat imagery; and (3) robust and accurate predictions due to improved data quality control, advanced machine learning techniques, and rigorous validation. The machine learning models yielded high cross-validation results. Stratification that uses separate Fluxnet stations for each fold of validation ensures that the models are accurate across the area covered by the Fluxnet sites. Overall, we present here a globally scaled technology for the land sector based on high resolution remote sensing imagery, meteorological variables, and direct carbon flux measurements of eddy covariance flux stations.
更多查看译文
关键词
net ecosystem exchange,eddy-covariance,regression,upscaling,data augmentation,feature selection
AI 理解论文
溯源树
样例
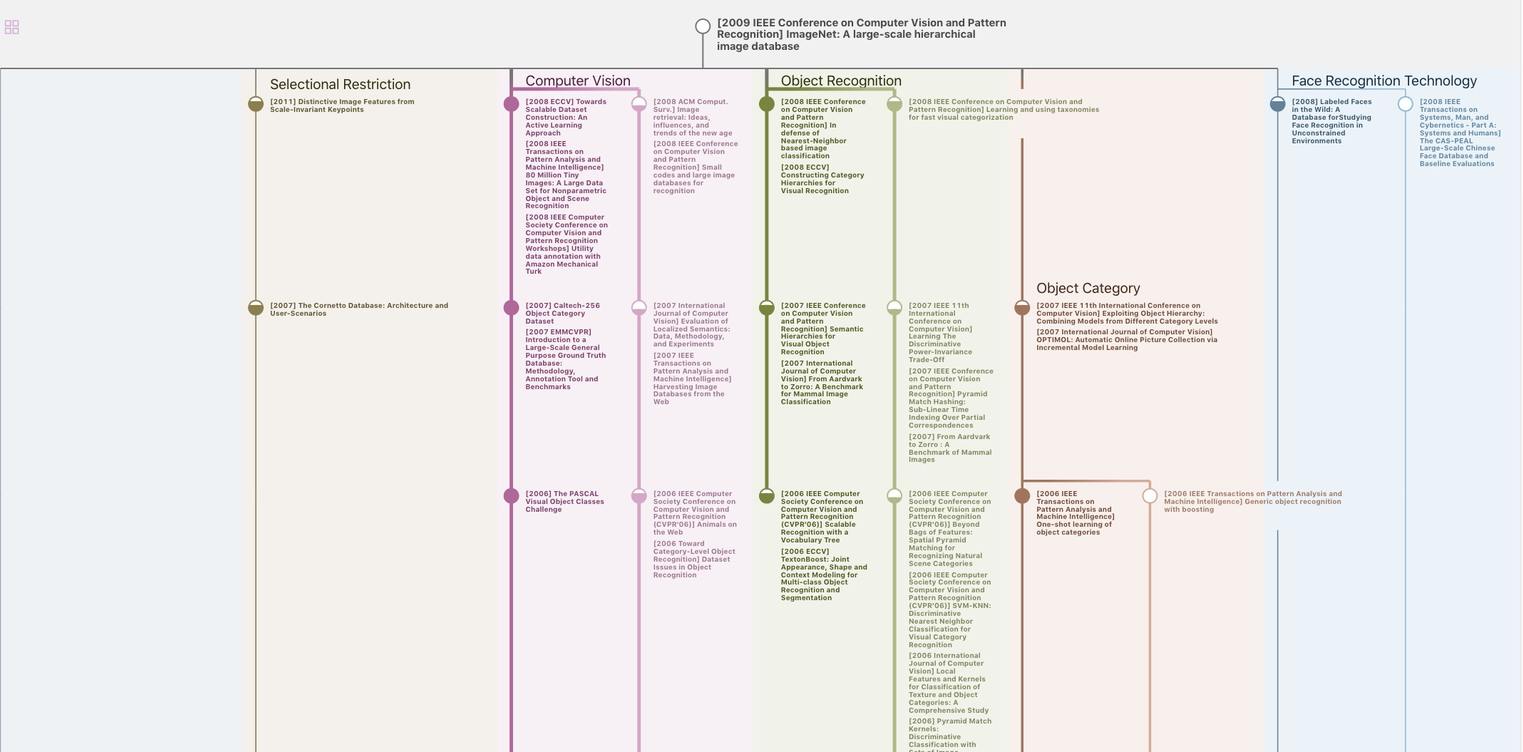
生成溯源树,研究论文发展脉络
Chat Paper
正在生成论文摘要