PolyODENet: Deriving mass-action rate equations from incomplete transient kinetics data.
The Journal of chemical physics(2022)
摘要
Kinetics of a reaction network that follows mass-action rate laws can be described with a system of ordinary differential equations (ODEs) with polynomial right-hand side. However, it is challenging to derive such kinetic differential equations from transient kinetic data without knowing the reaction network, especially when the data are incomplete due to experimental limitations. We introduce a program, PolyODENet, toward this goal. Based on the machine-learning method Neural ODE, PolyODENet defines a generative model and predicts concentrations at arbitrary time. As such, it is possible to include unmeasurable intermediate species in the kinetic equations. Importantly, we have implemented various measures to apply physical constraints and chemical knowledge in the training to regularize the solution space. Using simple catalytic reaction models, we demonstrate that PolyODENet can predict reaction profiles of unknown species and doing so even reveal hidden parts of reaction mechanisms.
更多查看译文
关键词
kinetics,mass-action
AI 理解论文
溯源树
样例
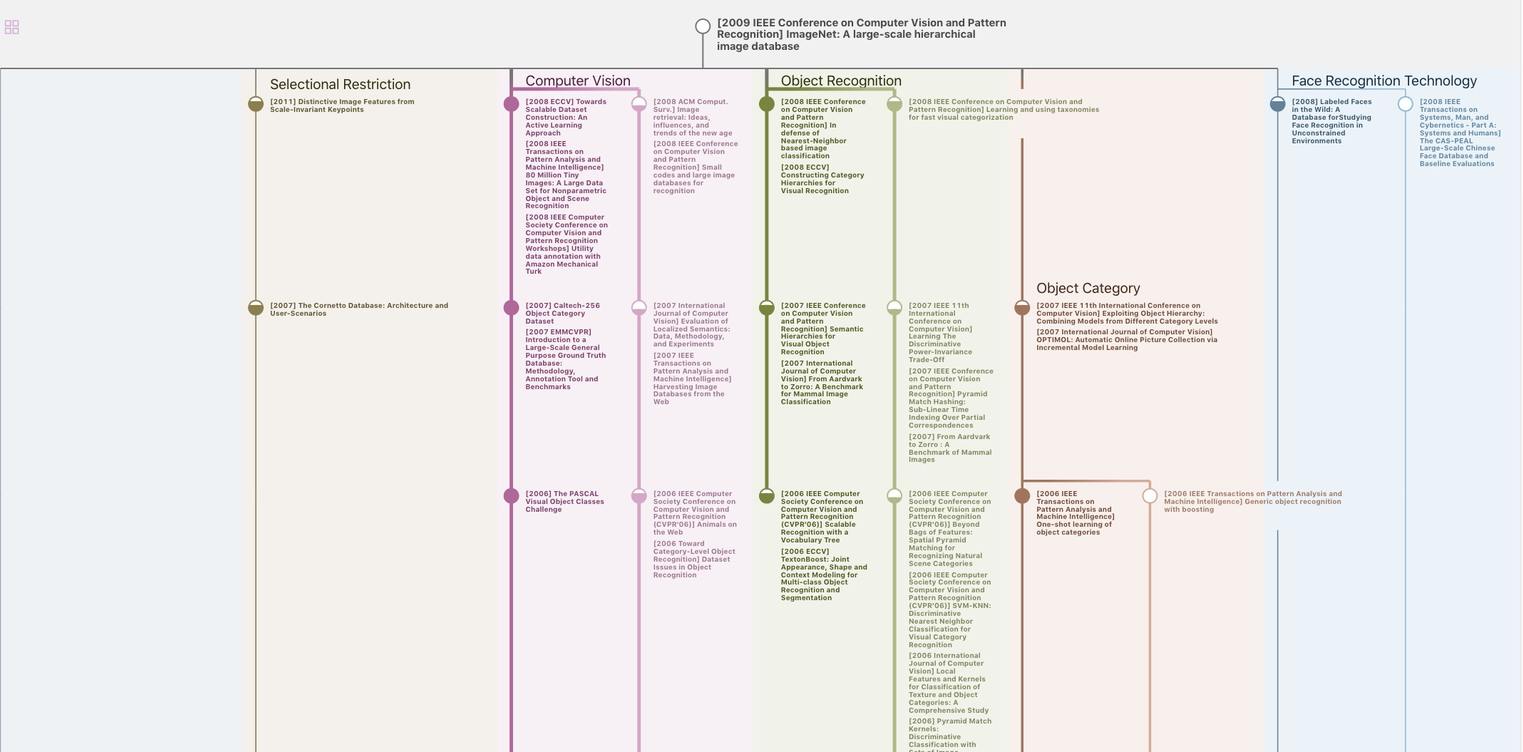
生成溯源树,研究论文发展脉络
Chat Paper
正在生成论文摘要