Time-aware Random Walk Diffusion to Improve Dynamic Graph Learning
arxiv(2023)
摘要
How can we augment a dynamic graph for improving the performance of dynamic graph neural networks? Graph augmentation has been widely utilized to boost the learning performance of GNN-based models. However, most existing approaches only enhance spatial structure within an input static graph by transforming the graph, and do not consider dynamics caused by time such as temporal locality, i.e., recent edges are more influential than earlier ones, which remains challenging for dynamic graph augmentation. In this work, we propose TiaRa (Time-aware Random Walk Diffusion), a novel diffusion-based method for augmenting a dynamic graph represented as a discrete-time sequence of graph snapshots. For this purpose, we first design a time-aware random walk proximity so that a surfer can walk along the time dimension as well as edges, resulting in spatially and temporally localized scores. We then derive our diffusion matrices based on the time-aware random walk, and show they become enhanced adjacency matrices that both spatial and temporal localities are augmented. Throughout extensive experiments, we demonstrate that TiaRa effectively augments a given dynamic graph, and leads to significant improvements in dynamic GNN models for various graph datasets and tasks.
更多查看译文
关键词
diffusion,graph,learning,time-aware
AI 理解论文
溯源树
样例
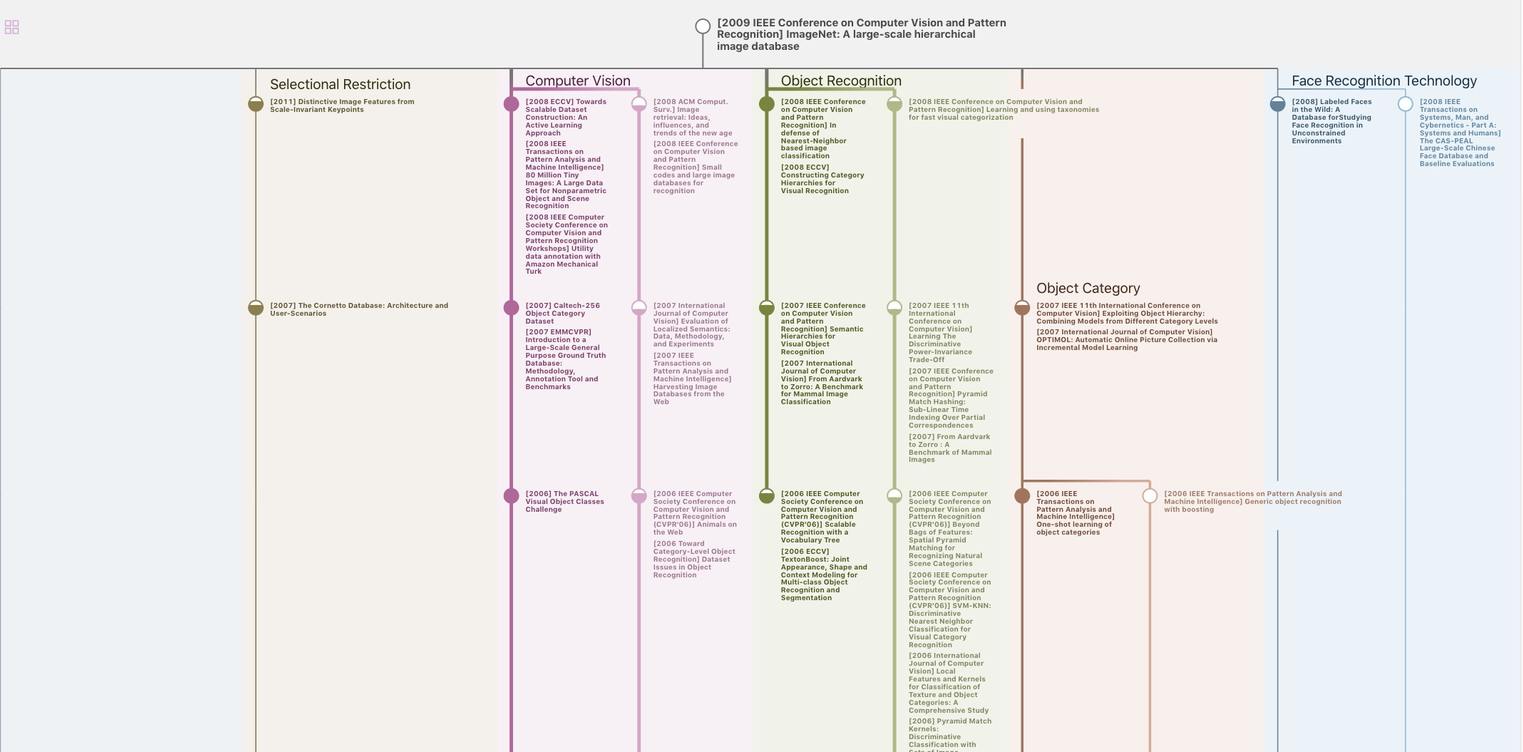
生成溯源树,研究论文发展脉络
Chat Paper
正在生成论文摘要