OPA-3D: Occlusion-Aware Pixel-Wise Aggregation for Monocular 3D Object Detection
IEEE Robotics and Automation Letters(2023)
摘要
Monocular 3D object detection has recently made a significant leap forward thanks to the use of pre-trained depth estimators for pseudo-LiDAR recovery. Yet, such two-stage methods typically suffer from overfitting and are incapable of explicitly encapsulating the geometric relation between depth and object bounding box. To overcome this limitation, we instead propose to jointly estimate dense scene depth with depth-bounding box residuals and object bounding boxes, allowing a two-stream detection of 3D objects that harnesses both geometry and context information. Thereby, the geometry stream combines visible depth and depth-bounding box residuals to recover the object bounding box via explicit occlusion-aware optimization. In addition, a bounding box based geometry projection scheme is employed in an effort to enhance distance perception. The second stream, named as the Context Stream, directly regresses 3D object location and size. This novel two-stream representation enables us to enforce cross-stream consistency terms, which aligns the outputs of both streams, and further improves the overall performance. Extensive experiments on the public benchmark demonstrate that OPA-3D outperforms state-of-the-art methods on the main Car category, whilst keeping a real-time inference speed.
更多查看译文
关键词
Computer vision for transportation,deep learning for visual perception,object detection
AI 理解论文
溯源树
样例
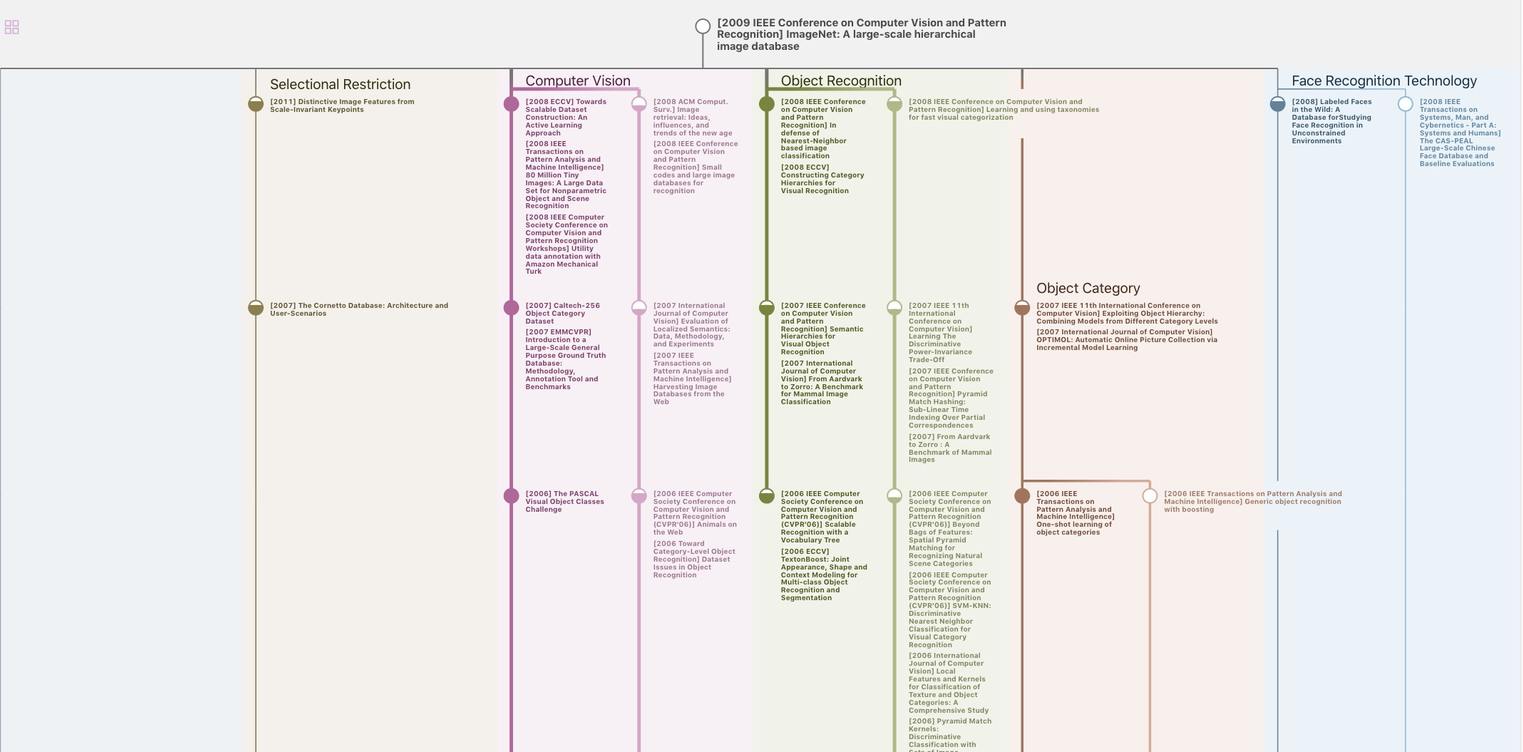
生成溯源树,研究论文发展脉络
Chat Paper
正在生成论文摘要