Identifying exploration profiles for higher education and their relationship with different student variables and outcomes
EUROPEAN JOURNAL OF PSYCHOLOGY OF EDUCATION(2022)
摘要
This study aims to better understand differences in the decision-making process behind study choices for higher education by investigating the presence of exploration profiles and then explore the explanatory base. To achieve this, we first identified different exploration profiles of students transitioning to higher education ( n = 5660), and then investigated whether they were predicted by different student variables (i.e., learning strategies, gender, and educational track) and linked with different outcomes of the decision-making process (i.e., the amount of information acquired regarding higher education, decisional status, and commitment). A latent profile analysis identified three exploration profiles based on the decisional tasks of orientation, self-, broad, and in-depth exploration: passive (35%), moderately active (52%), and highly active explorers (13%). Students’ learning strategies (regulation and processing strategies) were associated with these profiles. Students with more effective regulation and processing strategies were more likely to be highly active than passive or moderately active explorers. Female students and students from the technical track were more likely to be found in the highly active profile compared to the moderately active and the passive or moderately active profile, respectively. Finally, highly active explorers had the most favorable outcomes, measured by decisional status, commitment, and amount of information. Based on a substantial dataset, our findings contribute to a more comprehensive understanding of the explanatory base of important differences in the study choice making process of students opting for higher education. This may ultimately lead to more fitting support for students in less beneficial profiles.
更多查看译文
关键词
Study choice,Transition to higher education,Learning strategies,Latent profile analysis,Person-centered
AI 理解论文
溯源树
样例
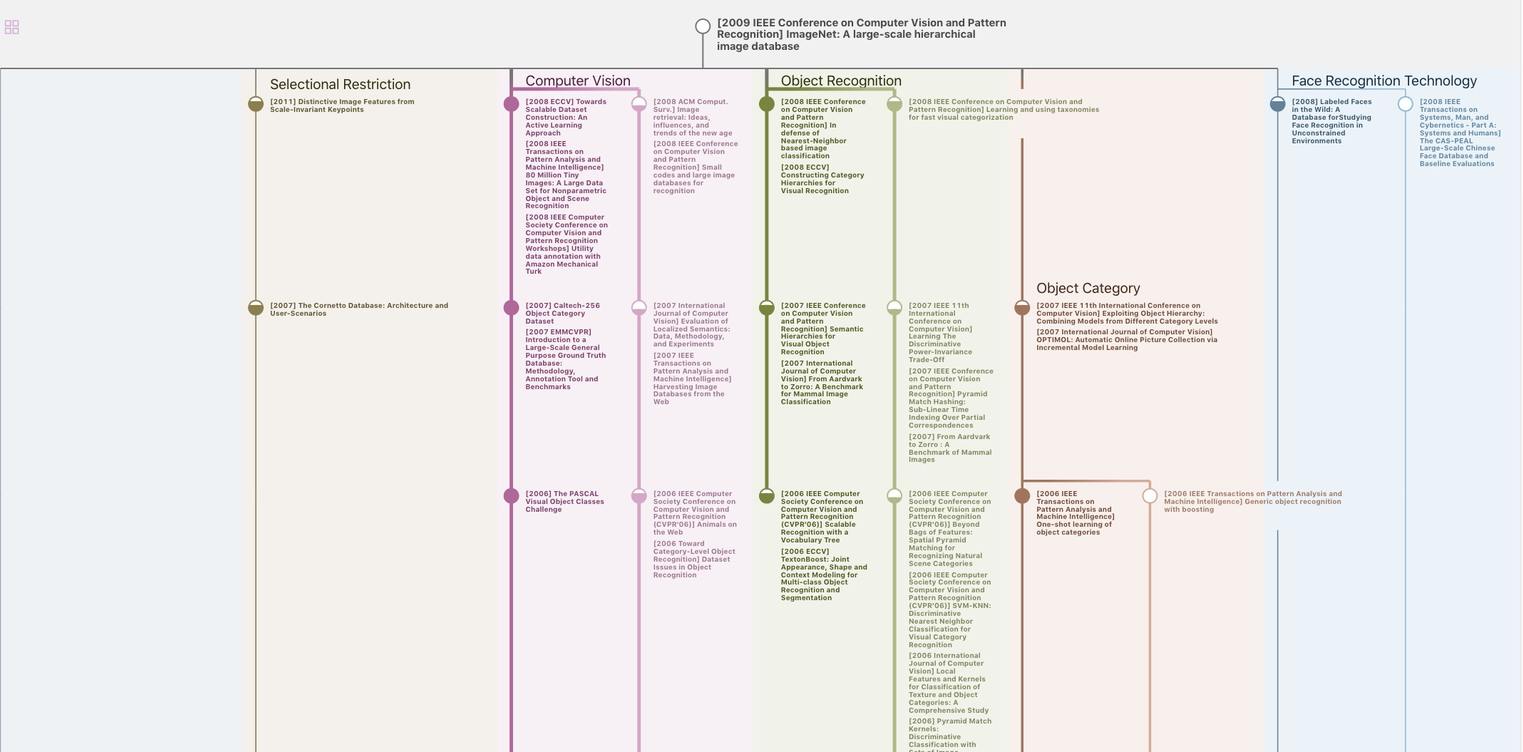
生成溯源树,研究论文发展脉络
Chat Paper
正在生成论文摘要