Quality Quantification in Deep Convolutional Neural Networks for Skin Lesion Segmentation using Fuzzy Uncertainty Measurement
2022 IEEE International Conference on Fuzzy Systems (FUZZ-IEEE)(2022)
摘要
Deep convolutional neural networks (DCNN)-based methods have achieved promising performance in semantic image segmentation. However, in practical applications, it is important not only to produce the segmentation result but also to inform the segmentation quality (e.g. confidence of the segmentation result). In this paper, we propose to utilize fuzzy sets for estimating segmentation uncertainty, therefore to infer the quality of segmentation produced by a DCNN model. The proposed method combines test-time augmentation and fuzzy sets to estimate an image-level uncertainty. Six different fuzziness measures are implemented and compared, in order to select the best fuzzy uncertainty metric for the proposed method. A public skin lesion dataset is used to evaluate the method. The results show a strong correlation (Pearson correlation coefficient of 0.736) between our proposed uncertainty measure and image segmentation quality measured by Dice coefficient.
更多查看译文
关键词
fuzzy sets,image segmentation,quality quantification,uncertainty,skin lesion
AI 理解论文
溯源树
样例
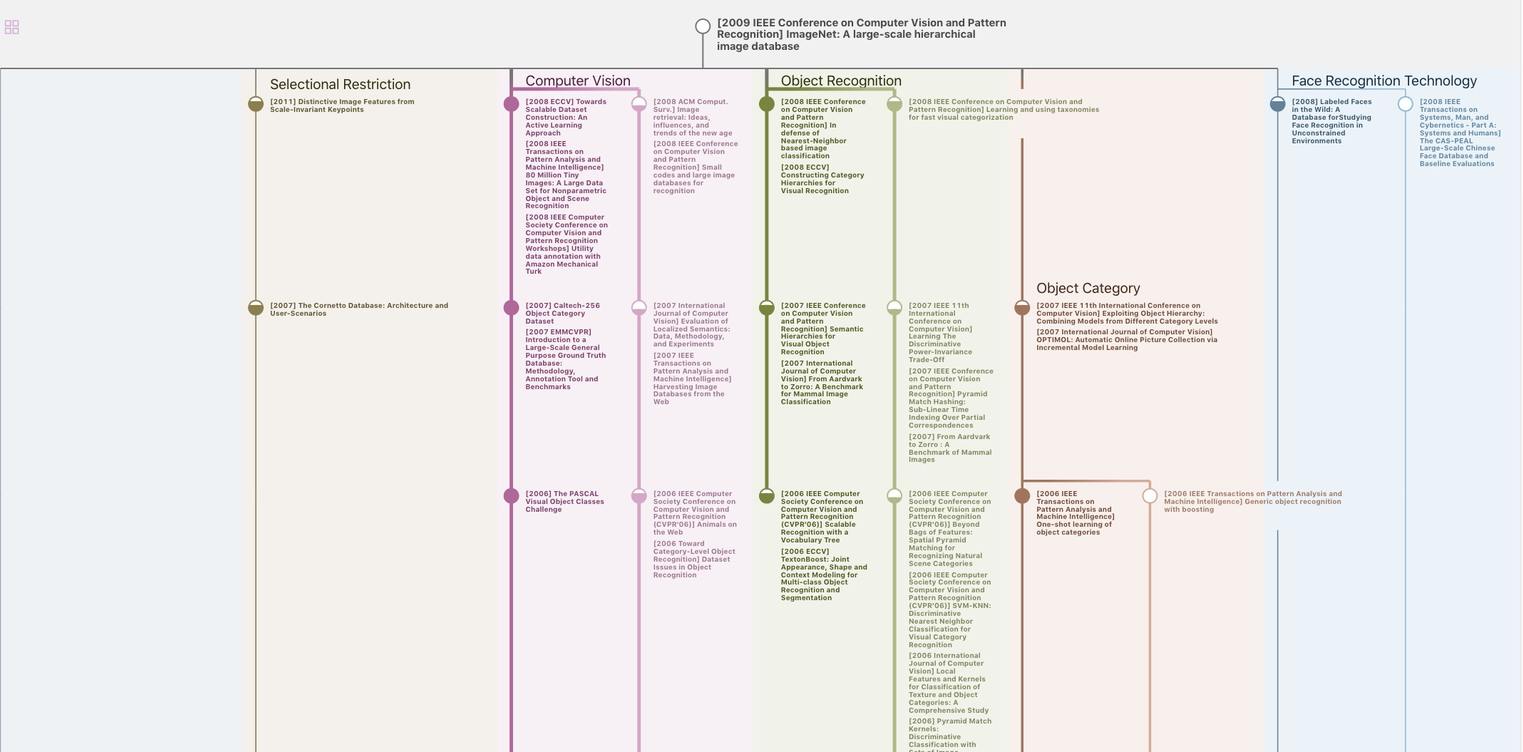
生成溯源树,研究论文发展脉络
Chat Paper
正在生成论文摘要