Kernel Fuzzy Clustering based on Quasi-Monte Carlo Feature Map with Neighbor Affinity Constraint
2022 IEEE International Conference on Fuzzy Systems (FUZZ-IEEE)(2022)
摘要
In recent years, kernel-based fuzzy clustering has attracted significant attention, primarily benefiting from the outstanding performance of capturing the potential non-linear structure in data clustering. However, many existing kernel clustering methods are not available for large datasets due to computational costs. To overcome this limitation, the low-rank random feature map is utilized to approximate the kernel space. Nevertheless, this kind of feature approximation method ignores the graph structure information hidden in the data and does not take the correlations between data samples in the clustering into account. Thus, we present a new kernel fuzzy clustering based on Quasi-Monte Carlo feature map with neighbor affinity constraint (Na_QMC_KFC). In this scheme, the Quasi-Monte Carlo method is adopted to approximate the Gaussian kernel function so as to reduce the computational costs. Meanwhile, the neighbor affinity constraint is designed to maintain the graph structure information of the data and further facilitate the consistency of the membership degrees and the raw data. What’s more, the Alternating Direction Method of Multipliers method is utilized to optimize the problem with respect to the neighbor affinity lasso. The experiments on several non-linear and real-world datasets exhibits the efficiency of the presented algorithm.
更多查看译文
关键词
kernel fuzzy clustering,Quasi-Monte Carlo method,neighbor affinity
AI 理解论文
溯源树
样例
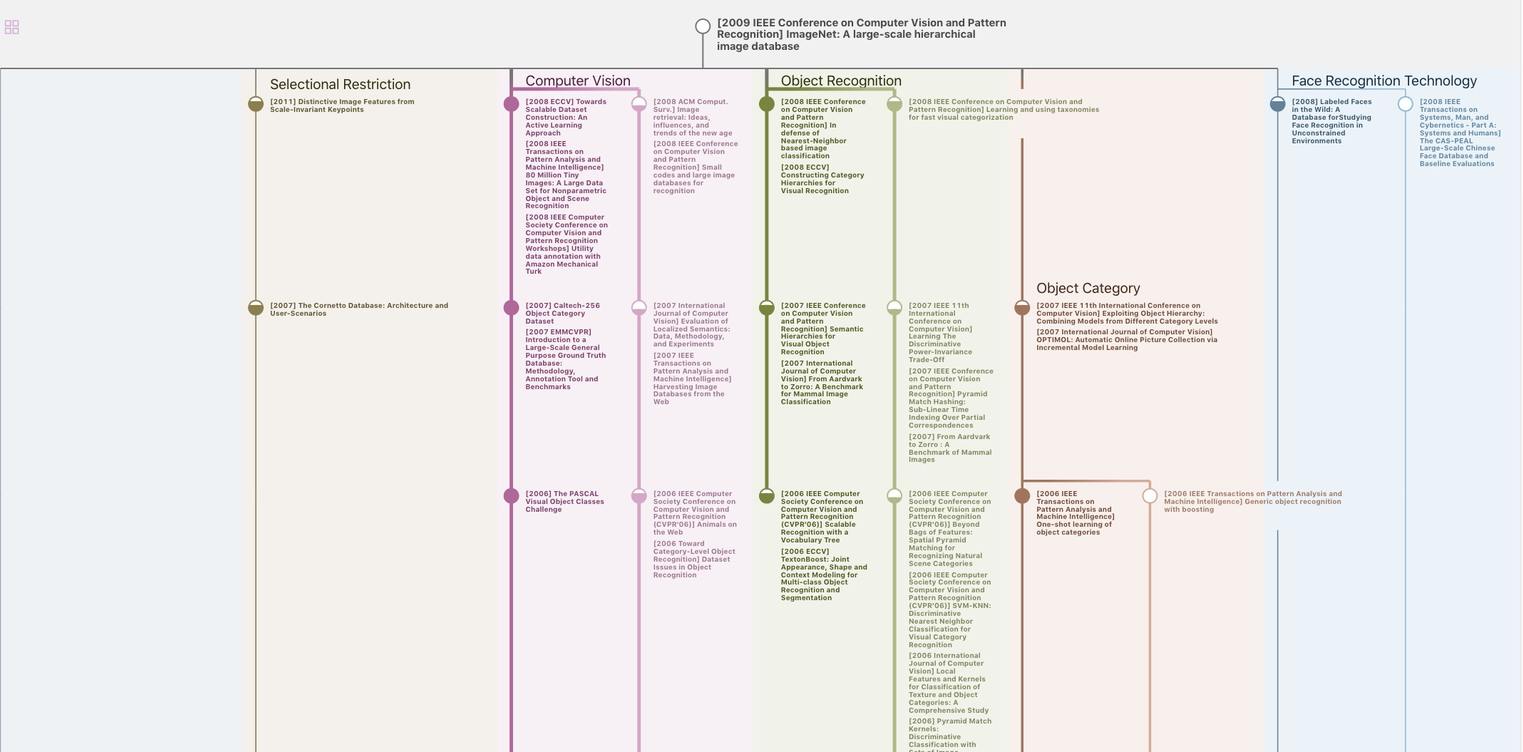
生成溯源树,研究论文发展脉络
Chat Paper
正在生成论文摘要