Does fluid structure encode predictions of glassy dynamics?
arxiv(2022)
摘要
Data-driven approaches to inferring the local structures responsible for plasticity in amorphous materials have made substantial contributions to our understanding of the failure, flow, and rearrangement dynamics of supercooled fluids. Some of these methods, such as the ``softness'' approach based on linear support vector machines, have identified combinations of local structural features of a supercooled particle's environment that predict energy barriers associated with particle rearrangements. This approach also predicts the onset temperature, often characterized as the temperature below which the system's dynamics becomes non-Arrhenius and above which local structures are no longer predictive of dynamical activity. We implement a transfer-learning approach in which we first show that classifiers can be trained to predict dynamical activity even far above the onset temperature. We then show that applying these classifiers to data from the supercooled phase recovers essentially the same physical information about the relationship between local structures and energy barriers that softness does.
更多查看译文
关键词
glassy dynamics,fluid structure,encode predictions
AI 理解论文
溯源树
样例
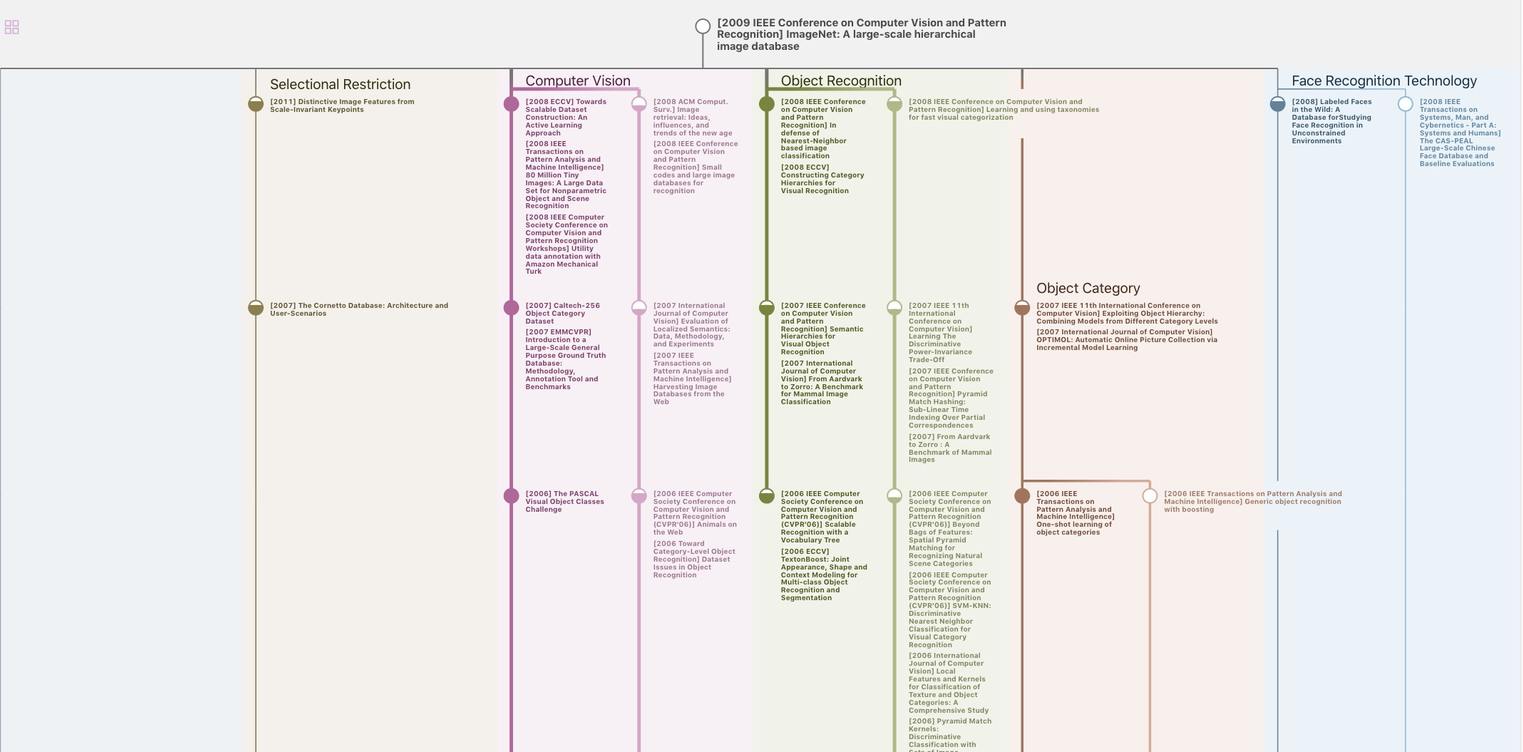
生成溯源树,研究论文发展脉络
Chat Paper
正在生成论文摘要