Interpretability in the Wild: a Circuit for Indirect Object Identification in GPT-2 small
ICLR 2023(2022)
摘要
Research in mechanistic interpretability seeks to explain behaviors of machine learning models in terms of their internal components. However, most previous work either focuses on simple behaviors in small models, or describes complicated behaviors in larger models with broad strokes. In this work, we bridge this gap by presenting an explanation for how GPT-2 small performs a natural language task called indirect object identification (IOI). Our explanation encompasses 26 attention heads grouped into 7 main classes, which we discovered using a combination of interpretability approaches relying on causal interventions. To our knowledge, this investigation is the largest end-to-end attempt at reverse-engineering a natural behavior "in the wild" in a language model. We evaluate the reliability of our explanation using three quantitative criteria--faithfulness, completeness and minimality. Though these criteria support our explanation, they also point to remaining gaps in our understanding. Our work provides evidence that a mechanistic understanding of large ML models is feasible, opening opportunities to scale our understanding to both larger models and more complex tasks.
更多查看译文
关键词
Mechanistic Interpretability,Transformers,Language Models,Interpretability,Transparency,Science of ML
AI 理解论文
溯源树
样例
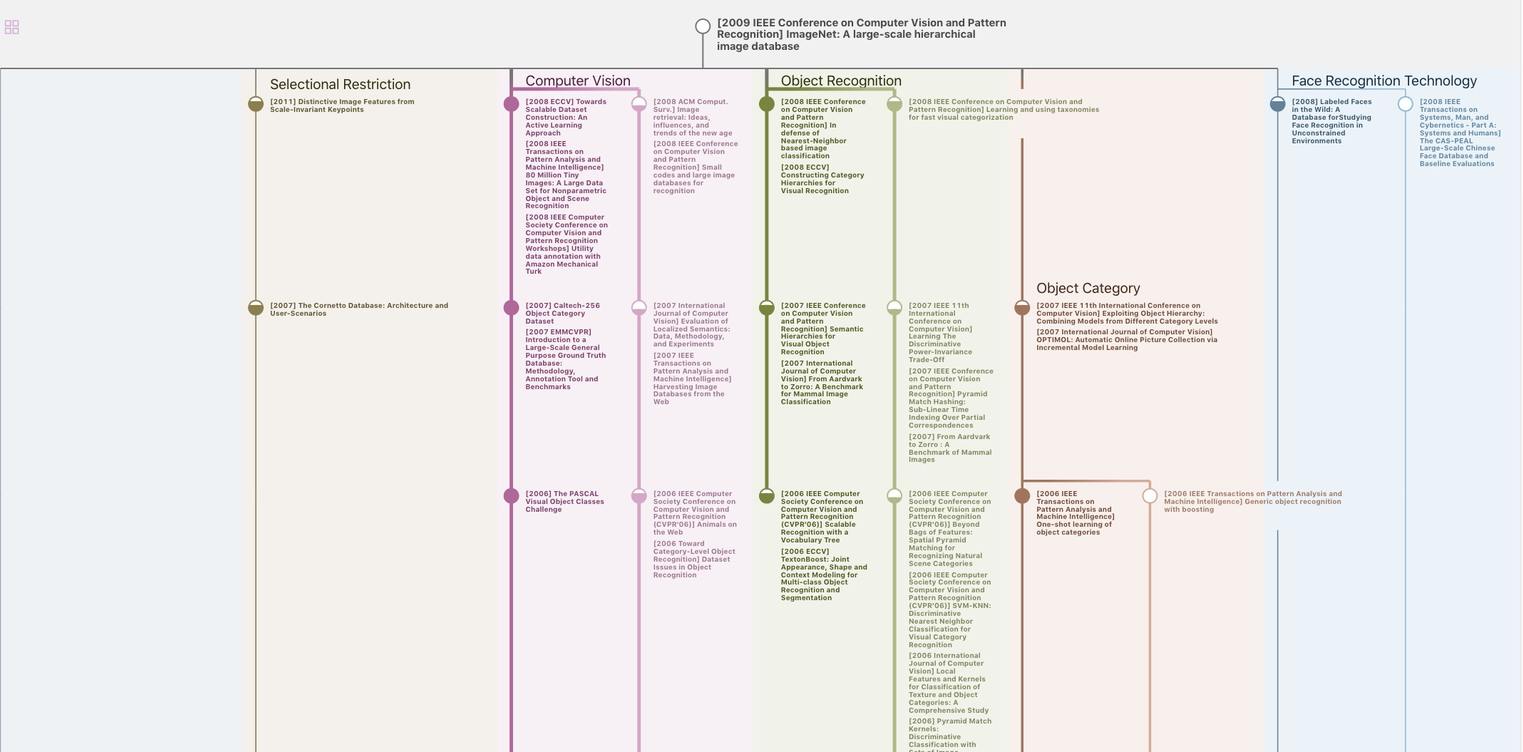
生成溯源树,研究论文发展脉络
Chat Paper
正在生成论文摘要