Classification of an 8-Band Multi-Spectral Dataset Using DCNNs with Weight Initializations Derived from Pre-Trained RGB Networks
IGARSS 2022 - 2022 IEEE International Geoscience and Remote Sensing Symposium(2022)
摘要
Modern satellite sensors can capture reflected optical energy from wavelengths well outside of the range of human perception. Indeed, it is well known that electromagnetic energy from the “non-visible” spectrum can be used to identify materials in geological, oceanographic, and agricultural contexts. What is less understood, however, is if and how this additional spectral information can be leveraged to aid in difficult computer vision tasks, e.g., classification or detection of man-made objects. Thus, we present the results from a series of experiments that evaluate the benefits that Deep Convolutional Neural Networks (DCNN) can garner through the inclusion of more spectral information. For training and testing these networks in image classification, we extract image tiles from the xView multi-spectral dataset. These images contain eight channels of spectral data including five wavelengths bands beyond the three bands traditionally used in computer vision researcher (red, green, and blue). In this work, we report the results of experiments that use an 80–20 train-test split with four DCNN architectures on full 8-band Multispectral Imagery (MSI) and various subsets of this eight banded imagery. The results show that networks trained on MSI have an average testing F1-score around 1.0 point higher than RGB networks trained with the same methods.
更多查看译文
关键词
Convolutional neural networks,image classification,Multispectral Imagery,remote sensing
AI 理解论文
溯源树
样例
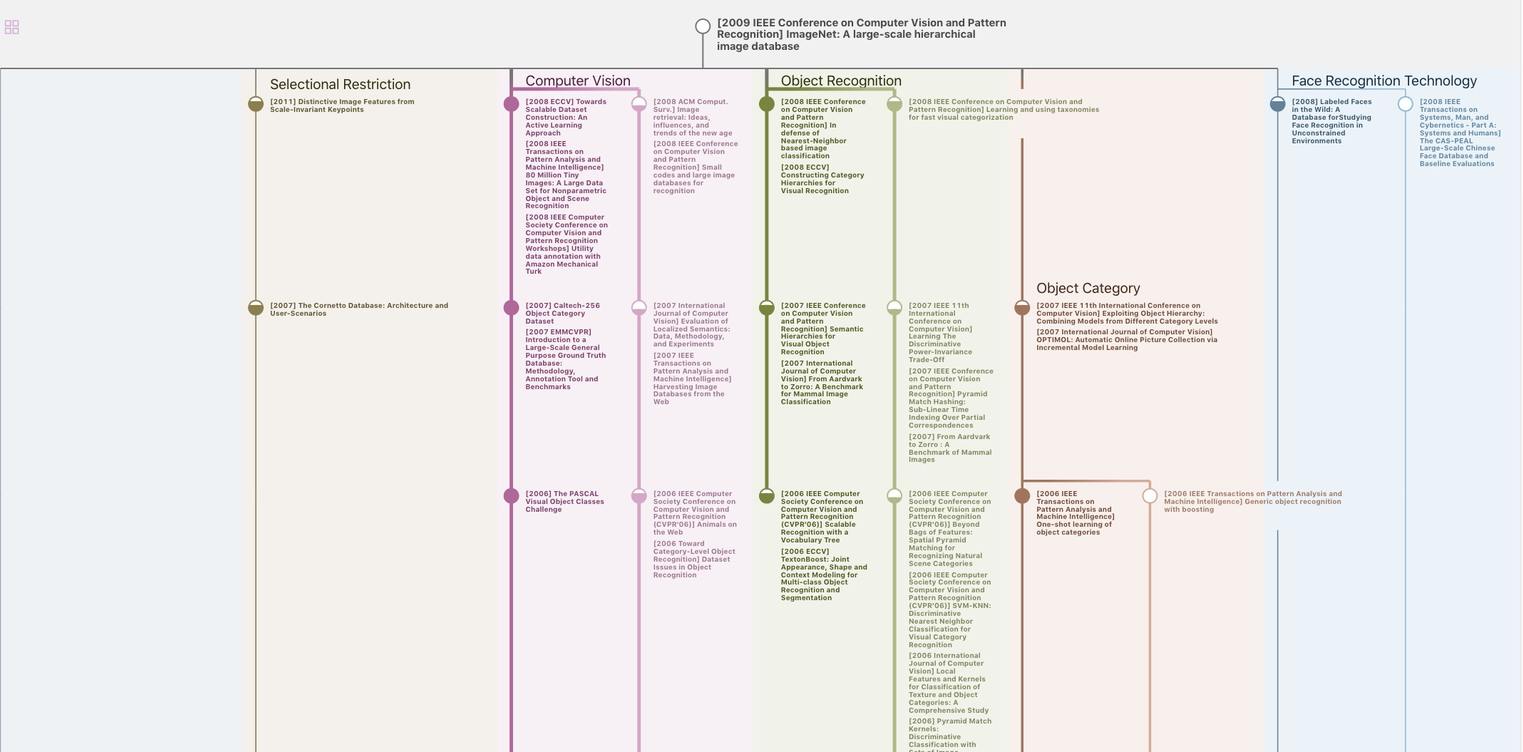
生成溯源树,研究论文发展脉络
Chat Paper
正在生成论文摘要