Adversarial supervised contrastive learning
MACHINE LEARNING(2022)
摘要
Contrastive learning is prevalently used in pre-training deep models, followed with fine-tuning in downstream tasks for better performance or faster training. However, pre-trained models from contrastive learning are barely robust against adversarial examples in downstream tasks since the representations learned by self-supervision may lack the robustness and also the class-wise discrimination. To tackle the above problems, we adapt the contrastive learning scheme to adversarial examples for robustness enhancement, and also extend the self-supervised contrastive approach to the supervised setting for the ability to discriminate on classes. Equipped with our new designs, we proposed adversarial supervised contrastive learning (ASCL), a novel framework for robust pre-training. Despite its simplicity, extensive experiments show that ASCL achieves significant margins in adversarial robustness over the prior arts, proceeding towards either the lightweight standard fine-tuning or adversarial fine-tuning. Moreover, ASCL also shows benefits for robustness to diverse natural corruptions, suggesting the wide applicability to all sorts of practical scenarios. Notably, ASCL demonstrate impressive results in robust transfer learning.
更多查看译文
关键词
Adversarial robustness,Adversarial attack,Self-supervised learning,Contrastive learning,Consistency regularization
AI 理解论文
溯源树
样例
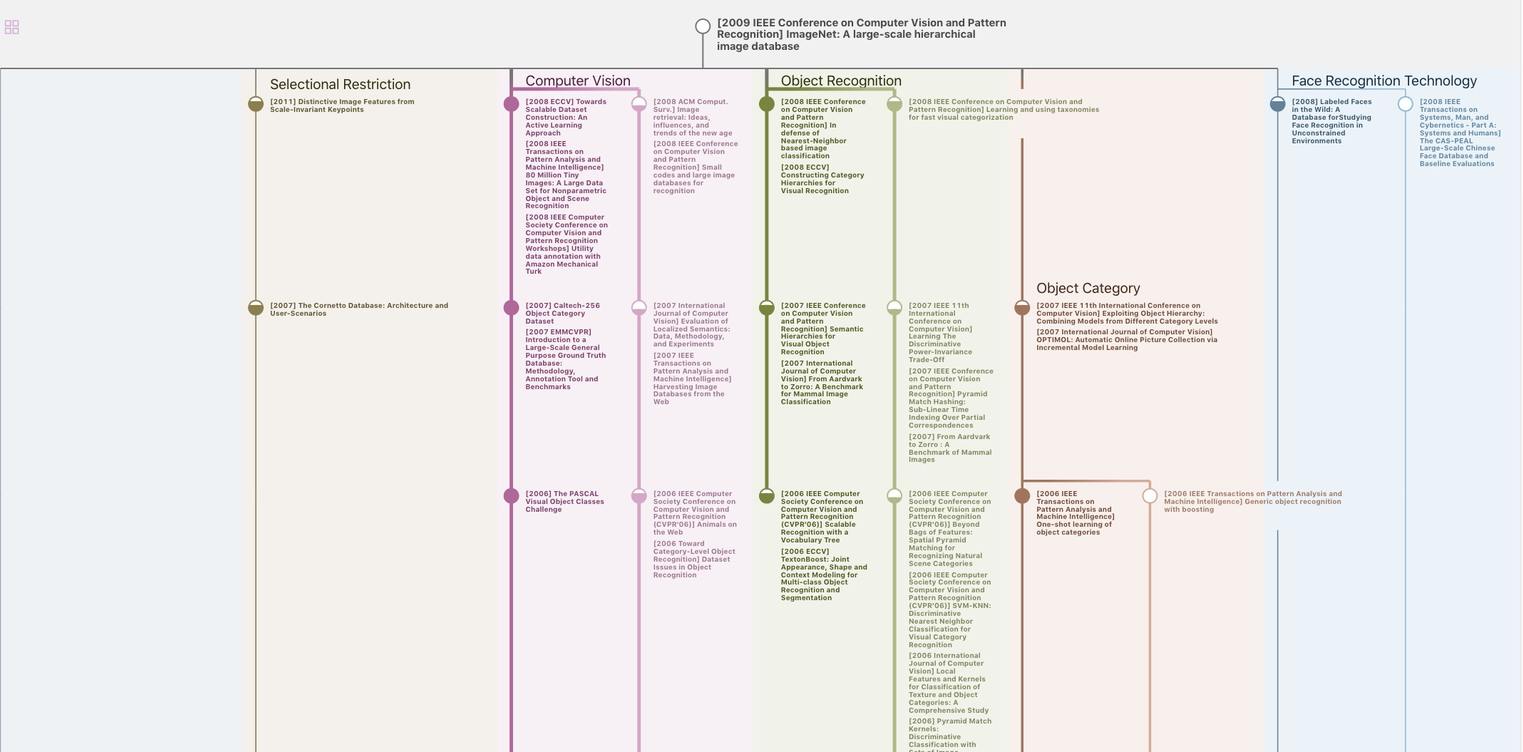
生成溯源树,研究论文发展脉络
Chat Paper
正在生成论文摘要