A mathematical model of coronavirus transmission by using the heuristic computing neural networks
Engineering Analysis with Boundary Elements(2023)
摘要
In this study, the nonlinear mathematical model of COVID-19 is investigated by stochastic solver using the scaled conjugate gradient neural networks (SCGNNs). The nonlinear mathematical model of COVID-19 is represented by coupled system of ordinary differential equations and is studied for three different cases of initial conditions with suitable parametric values. This model is studied subject to seven class of human population N(t) and individuals are categorized as: susceptible S(t), exposed E(t), quarantined Q(t), asymptotically diseased IA(t), symptomatic diseased IS(t) and finally the persons removed from COVID-19 and are denoted by R(t). The stochastic numerical computing SCGNNs approach will be used to examine the numerical performance of nonlinear mathematical model of COVID-19. The stochastic SCGNNs approach is based on three factors by using procedure of verification, sample statistics, testing and training. For this purpose, large portion of data is considered, i.e., 70%, 16%, 14% for training, testing and validation, respectively. The efficiency, reliability and authenticity of stochastic numerical SCGNNs approach are analysed graphically in terms of error histograms, mean square error, correlation, regression and finally further endorsed by graphical illustrations for absolute errors in the range of 10−05 to 10−07 for each scenario of the system model.
更多查看译文
关键词
Nonlinear,COVID-19,Quarantine,Scaled conjugate gradient,SCGNNs,Numerical results,Runge-Kutta scheme
AI 理解论文
溯源树
样例
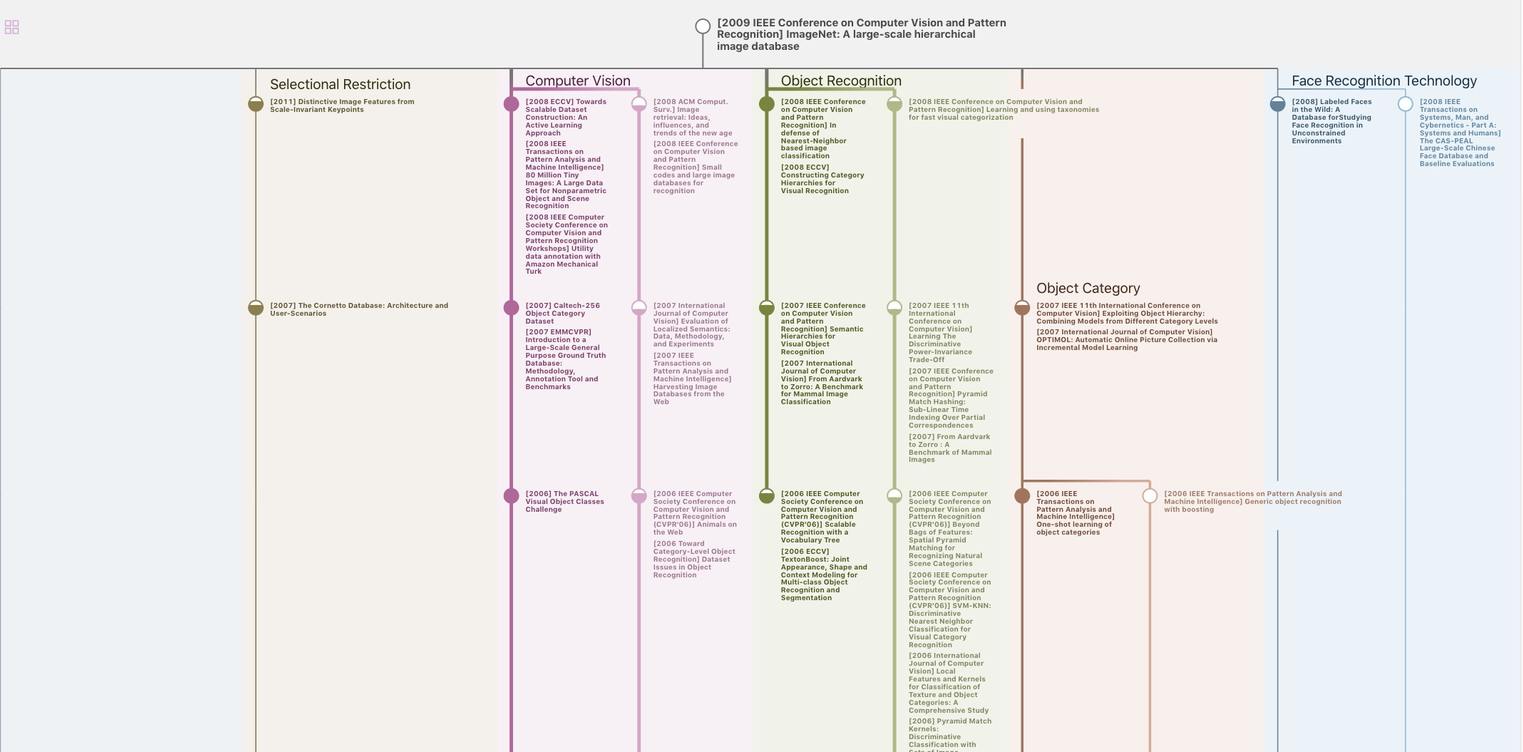
生成溯源树,研究论文发展脉络
Chat Paper
正在生成论文摘要