Improving scalability of multi-agent reinforcement learning with parameters sharing
2022 IEEE International Conference on Joint Cloud Computing (JCC)(2022)
摘要
Improving the scalability of a multi-agent system is one of the key challenges for applying reinforcement learning to learn an effective policy. Parameter sharing is a common approach used to improve the efficiency of learning by reducing the volume of policy network parameters that need to be updated. However, sharing parameters also reduces the variance between agents’ policies, which further restricts the diversity of their behaviors. In this paper, we introduce a policy parameter sharing approach, it maintains a policy network for each agent, and only updates one of them. The differentiated behavior of agents is maintained by the policy, while sharing parameters are updated through a soft way. Experiments in foraging scenarios demonstrate that our method can effectively improve the performance and also the scalability of the multi-agent systems.
更多查看译文
关键词
Deep reinforcement learning,Multi-agent sys-tem,Scalability
AI 理解论文
溯源树
样例
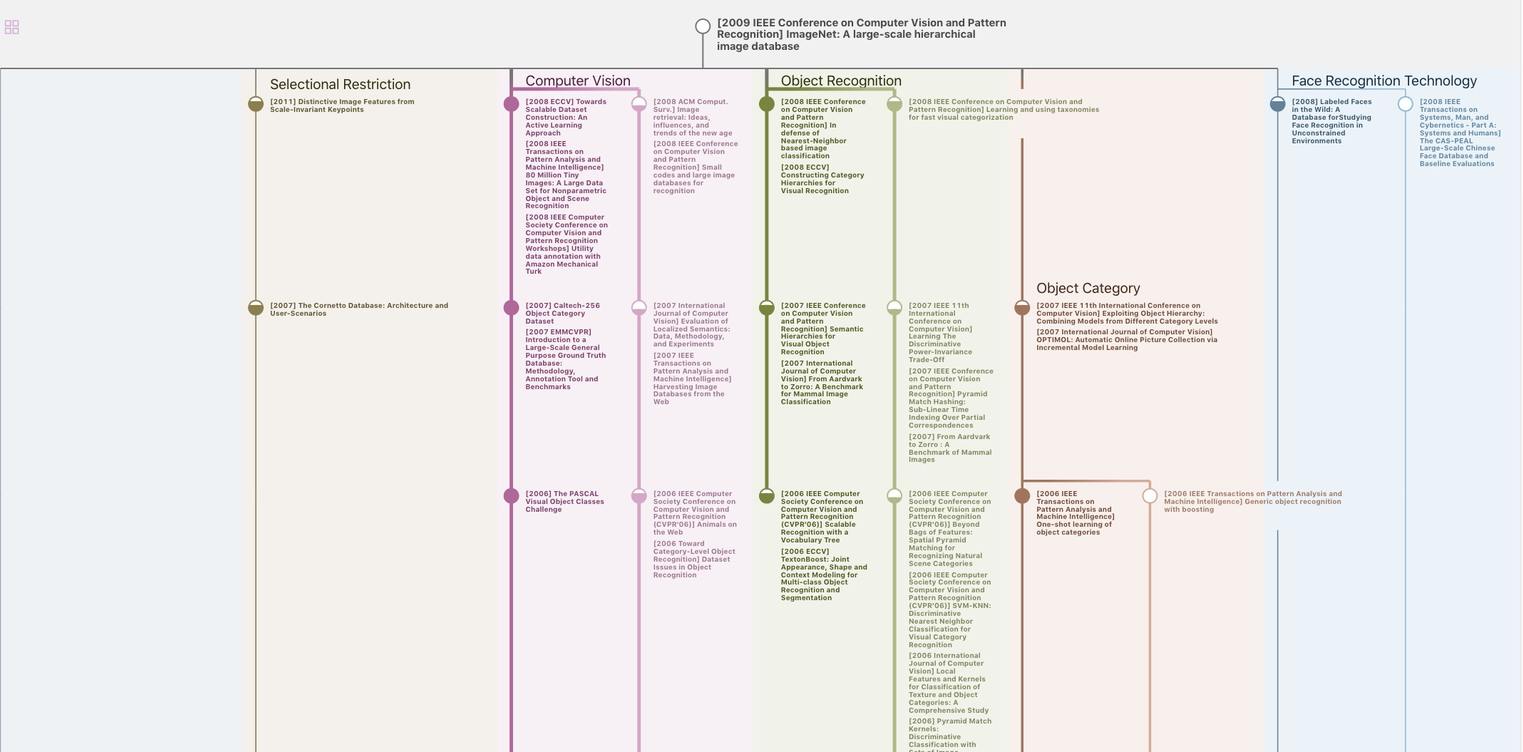
生成溯源树,研究论文发展脉络
Chat Paper
正在生成论文摘要