RGLS and RLS in Covariance Structure Analysis
Structural Equation Modeling: A Multidisciplinary Journal(2022)
摘要
This paper assesses the performance of regularized generalized least squares (RGLS) and reweighted least squares (RLS) methodologies in a confirmatory factor analysis model. Normal theory maximum likelihood (ML) and generalized least squares (GLS) statistics are based on large sample statistical theory. However, ML and GLS goodness-of-fit tests often make incorrect decisions on the true model, when sample size is small. The novel methods RGLS and RLS aim to correct the over-rejection by ML and under-rejection by GLS. Both methods outperform ML and GLS when samples are small, yet no studies have compared their relative performance. A Monte Carlo simulation study was carried out to examine the statistical performance of these two methods. We find that RLS and RGLS have equivalent performance when N >= 70; whereas when N <70, RLS outperforms RGLS. Both methods clearly outperform ML and GLS with N <= 400. Nonetheless, adopting mean and variance adjusted test for non-normal data, RGLS slightly outperforms RLS. Power analyses found that RLS generally showed small loss in power compared to ML and performed better than RGLS.
更多查看译文
关键词
Covariance structure,eigenvalue,estimation method,goodness of fit,weight matrix
AI 理解论文
溯源树
样例
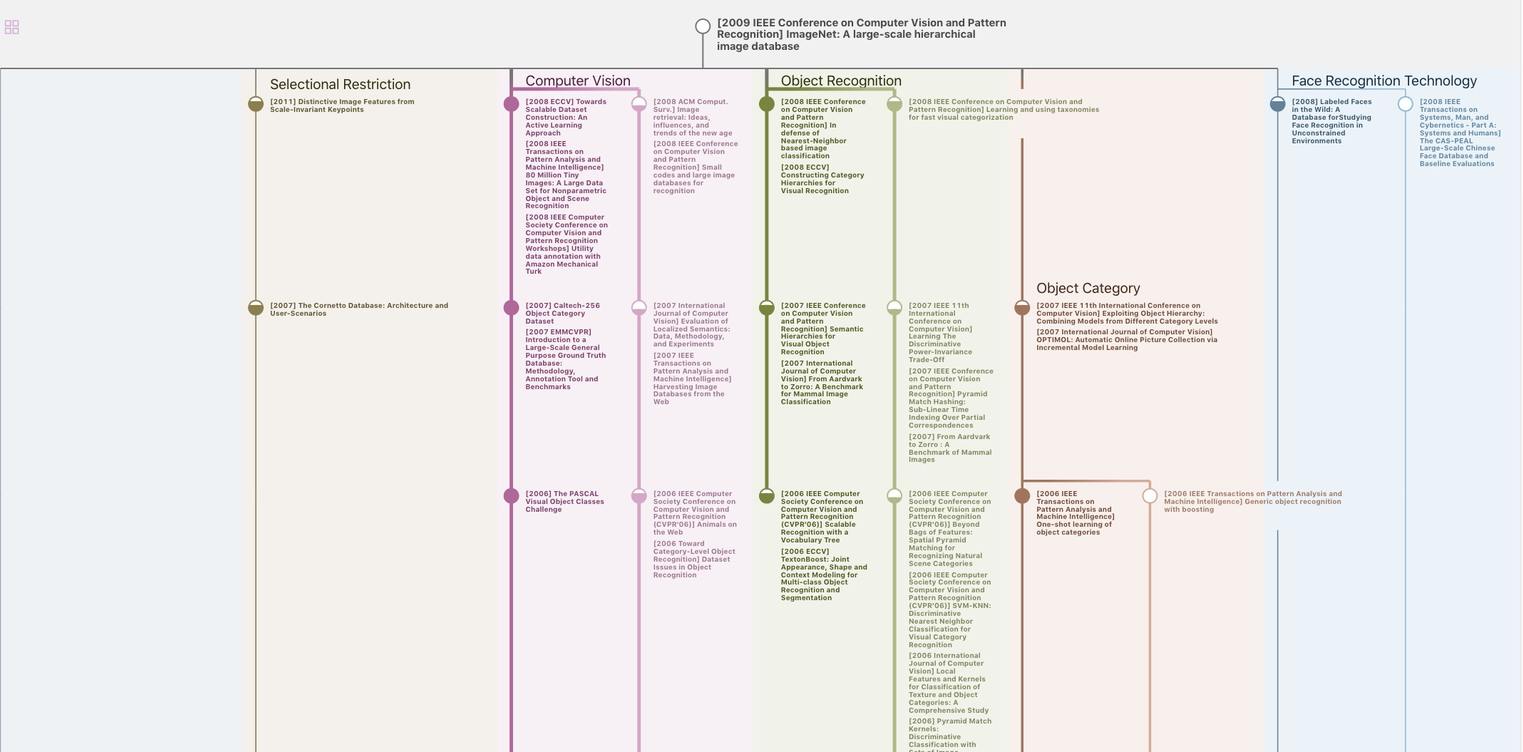
生成溯源树,研究论文发展脉络
Chat Paper
正在生成论文摘要