In-situ monitoring system for weld geometry of laser welding based on multi-task convolutional neural network model
Measurement(2022)
Abstract
This paper presents a low-cost, robust, in-situ monitoring system for weld geometry that can achieve multi-task prediction. First, the system uses a low-cost CCD camera to monitor the melt pool in the laser keyhole welding process. Then, the proposed novel multi-task convolutional neural network (Multi-task CNN) model is used to simultaneously complete the two prediction tasks of weld depth and width. Furthermore, the learning process of the Multi-task CNN model is explored using a visual feature map approach and the robustness of the model is demonstrated. Compared with Support Vector Machine, K-Nearest Neighbor, Bayesian Ridge, Decision Tree, the proposed Multi-task CNN model has the highest prediction accuracy. The model predicts a mean absolute per-centage error (MAPE, relative to ground truth) of 3.0% and 1.9% for weld depth and width. The in-situ moni-toring results show that the system can achieve accurate predictions, and the average time-consuming of the system is 23.35 ms.
MoreTranslated text
Key words
In -situ monitoring,CCD camera,Laser keyhole welding,Data augmentation,Multi -task convolutional neural network,Feature map
AI Read Science
Must-Reading Tree
Example
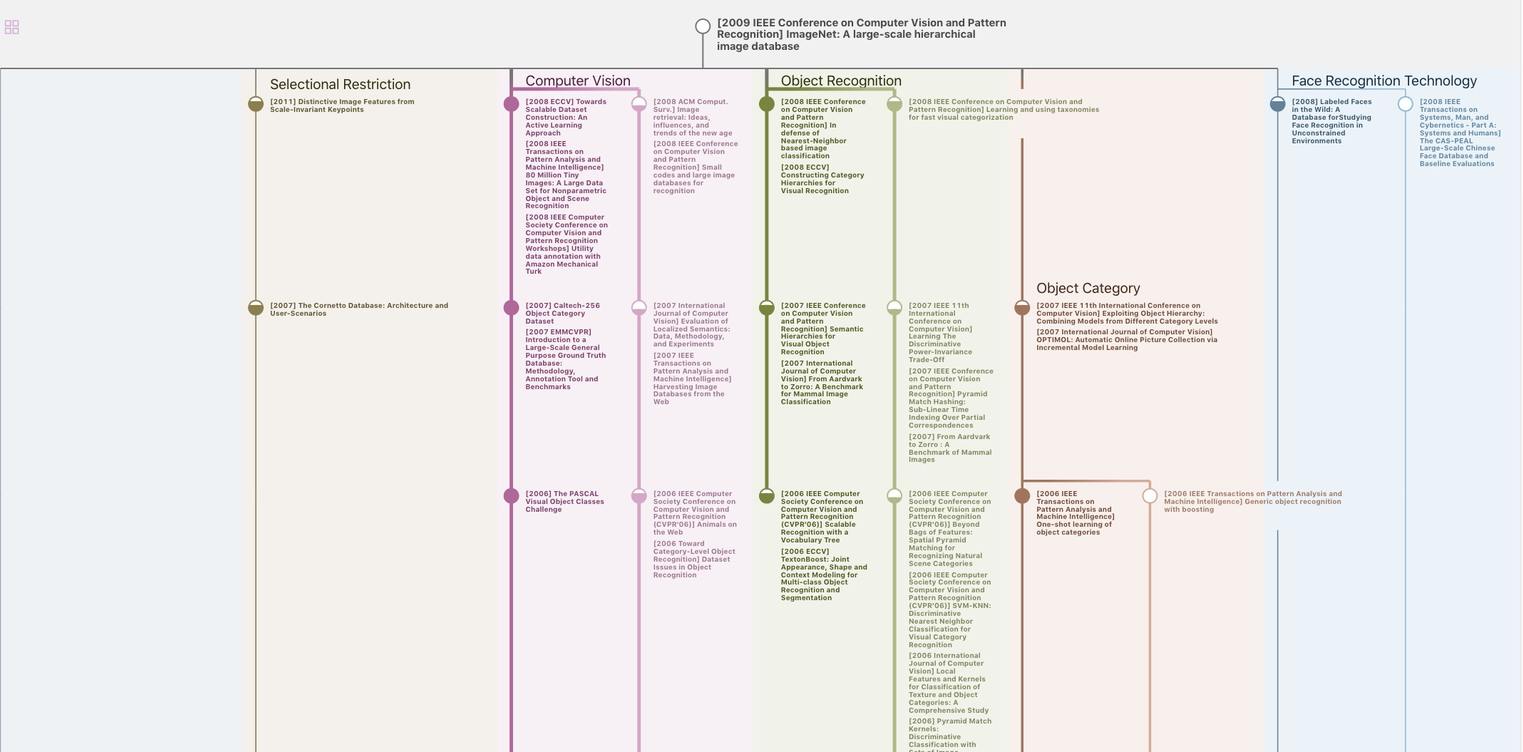
Generate MRT to find the research sequence of this paper
Chat Paper
Summary is being generated by the instructions you defined