A Robust Dynamic EEG Channel Selection using Time–Frequency Extended Renyi Entropy
2022 7th International Conference on Image and Signal Processing and their Applications (ISPA)(2022)
摘要
Epilepsy is a chronic disorder characterized by repeated seizures that may be detected by examining Electroencephalogram (EEG) data. Several analysis approaches represent seizure-related content within long-term EEG signals. However, the dimensionality issue inherent in processing many EEG channels hampers the performance. Therefore, many channel selection algorithms define the most relevant channels to overcome this dimensionality issue. Nonetheless, these techniques adopt static selection towards EEG channels, making them unable to follow the dynamic behavior of the cerebral activity. In this paper, we propose a dynamic channel selection algorithm based on the time–frequency extended Renyi Entropy (RE) and apply this algorithm on high–resolution quadratic time–frequency distributions, namely; the Spectrogram (SP), the Smoothed Pseudo Wigner–Ville Distribution (SPWVD), and the Choi–Williams Distribution (CWD) for comparison purposes. Combining the SPWVD with a kNN classifier, the suggested algorithm produces encouraging results, reaching an overall accuracy of 99.23%, a sensitivity of 100%, and an F1–score of 90.90% with a Matthews Correlation Coefficient (MCC) of 0.90, which makes it a reliable technique for selecting relevant channels to reduce EEG dimensionality.
更多查看译文
关键词
Dynamic channel selection,spectrogram,Smoothed Pseudo Wigner–Ville Distribution,Choi–Williams Distribution,time–frequency Renyi entropy
AI 理解论文
溯源树
样例
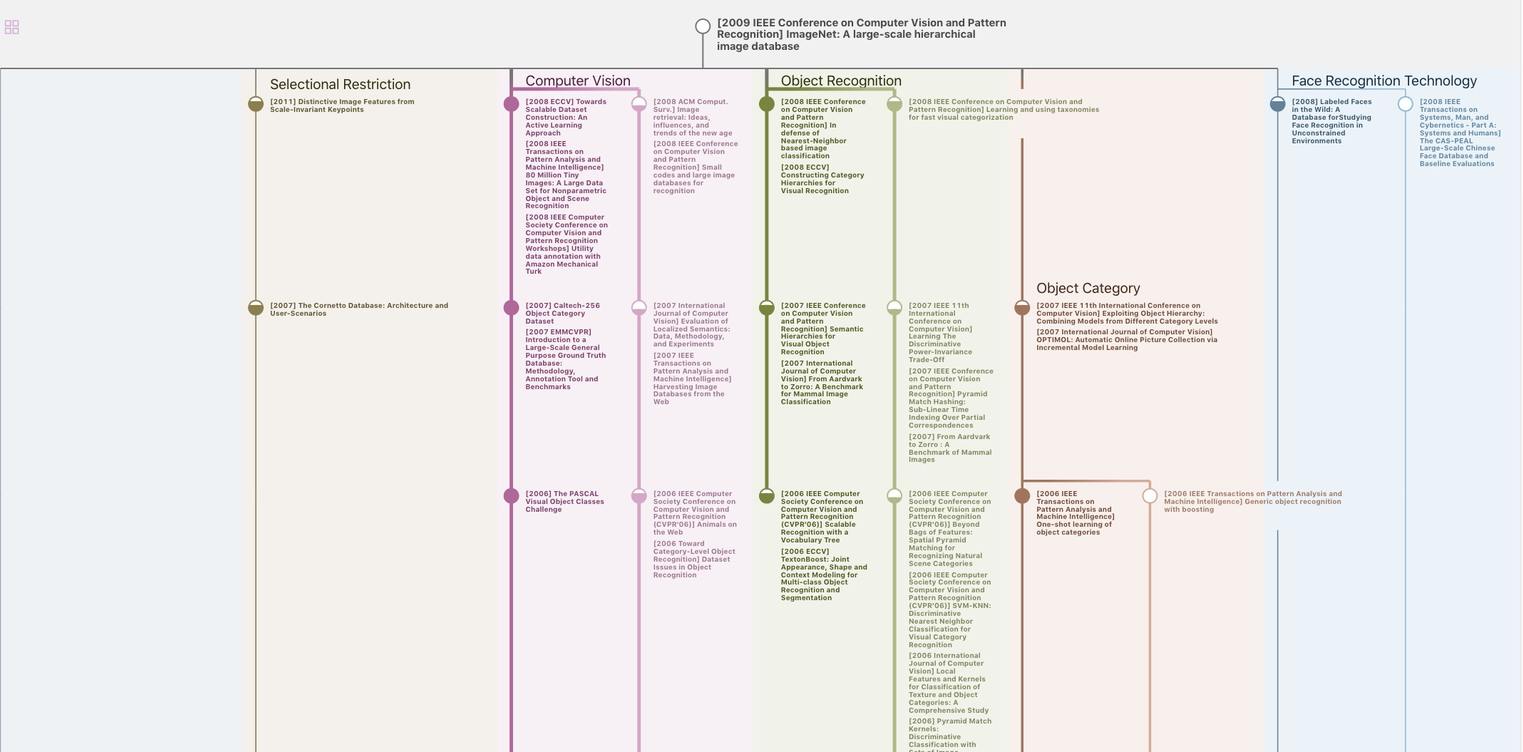
生成溯源树,研究论文发展脉络
Chat Paper
正在生成论文摘要