Deep dilated CNN based image denoising
International Journal of Information Technology(2022)
摘要
Over the past few years various convolutional neural network (CNN) based approaches have been applied in image denoising. Acknowledging the size of the receptive field in an image denoising problem can increase the network’s performance. The present research has altered the receptive field and studied its effect on image denoising. Three networks have been designed and compared– (1) CNN with dilated kernels (2) CNN without dilation but with increased kernel size with similar receptive field and (3) CNN without any dilation and without increased kernel size. After analyzing the results of three cases an additional fourth case is added with an optimum receptive field, which improves upon the state-of-the-art results. Most methods are based on some assumptions on natural images, here a method relying on a purely learning based approach is presented. The simulation has been conducted with randomized noise levels. A single network is able to handle a wide range of noise levels (σ). For 24-bit images with 256 gray scales for each color, it can reduce noise for standard deviation (σ) in the range of [0–75]. Comparable (with contemporary research) results have been presented in terms of qualitative measurements and visual perspectives. Performance of our approach can be validated with various test sets used in our study. We have achieved comparable PSNR values on existing standard test sets.
更多查看译文
关键词
Image denoising,Convolutional neural networks,Deep learning
AI 理解论文
溯源树
样例
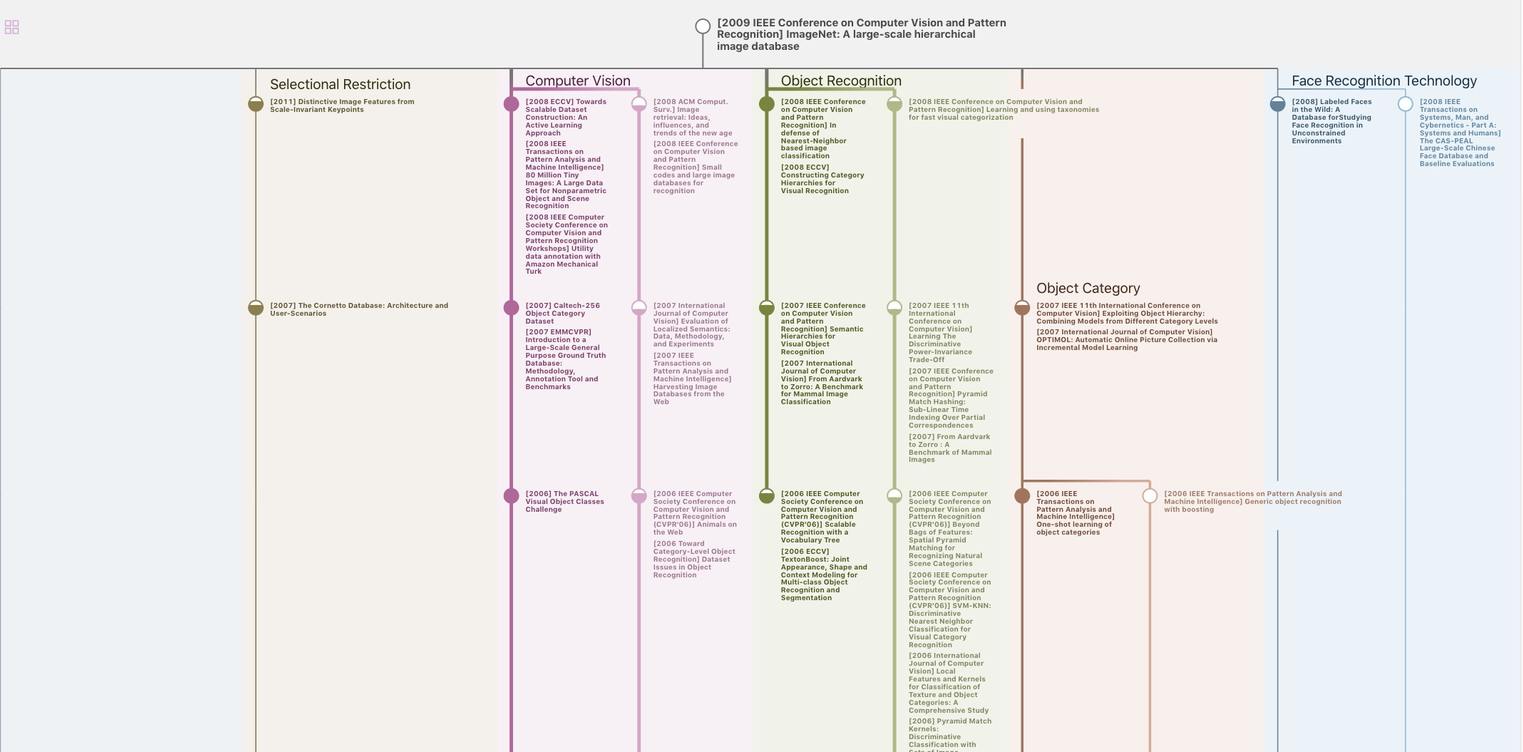
生成溯源树,研究论文发展脉络
Chat Paper
正在生成论文摘要