Qualitative and quantitative prediction of food allergen epitopes based on machine learning combined with in vitro experimental validation
FOOD CHEMISTRY(2023)
Abstract
An allergen epitope is a part of molecules that can specifically bind to immunoglobulin E (IgE), causing an allergic reactions. To predict protein epitopes and their binding ability to IgE, quantitative structure-activity relationship (QSAR) models were established using four algorithms combined with the selected chemical descriptors. The model predicted the binding capabilities of the epitopes to IgE with the R2 and root mean squared error (RMSE) as 0.7494 and 0.2375, respectively. The model's performance was validated using an enzymelinked immunosorbent assay (ELISA). The results showed that the established QSAR model could efficiently and accurately predict the allergic reaction of food protein epitopes. The prediction results of the model and the experimental results were consistent, with a Pearson correlation coefficient of 0.8956. The results from both the QSAR model and in vitro experiments indicated that amino acid sequence 116-130 was a novel IgE-binding epitope of beta-LG.
MoreTranslated text
Key words
IgE-binding epitopes,Prediction,Machine learning,QSAR,ELISA
AI Read Science
Must-Reading Tree
Example
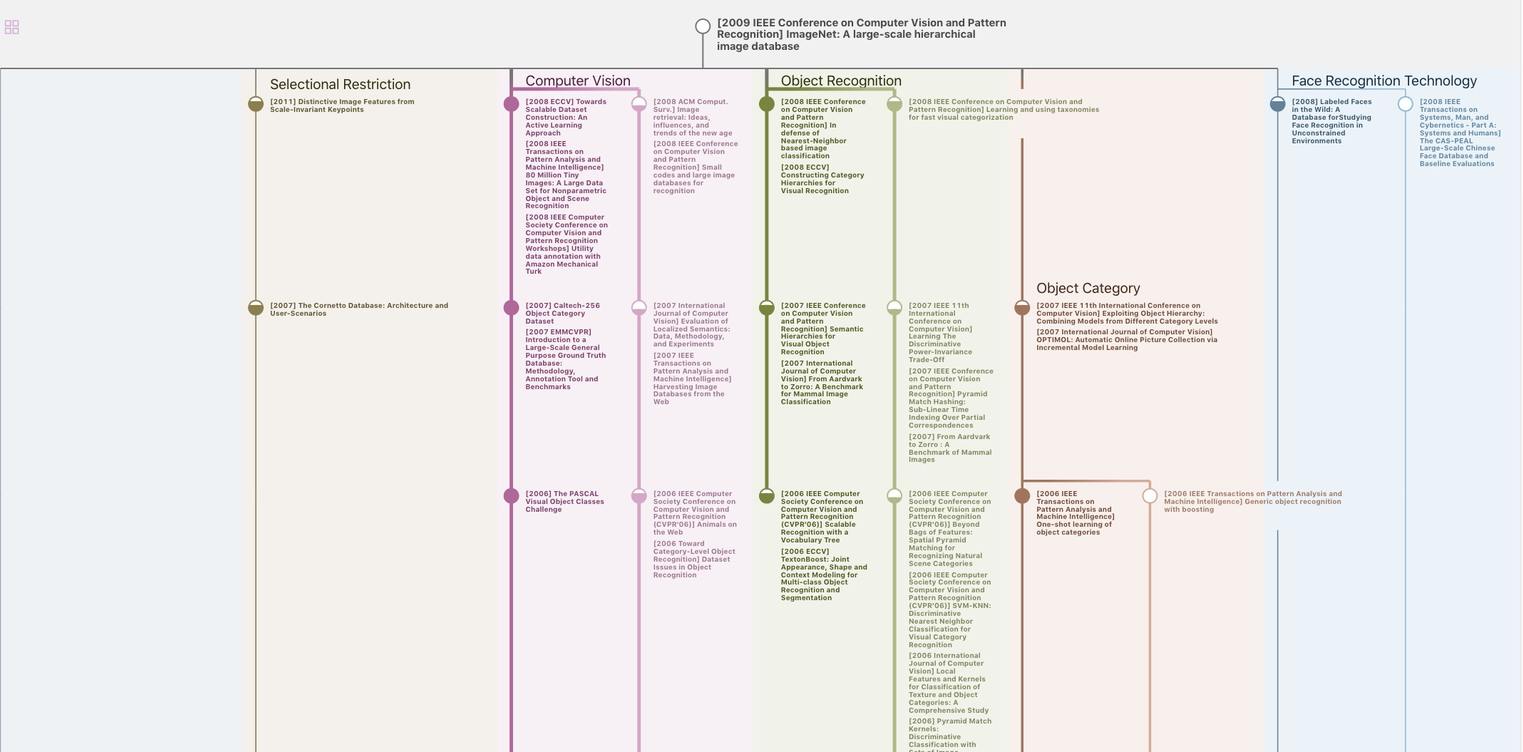
Generate MRT to find the research sequence of this paper
Chat Paper
Summary is being generated by the instructions you defined