Low Complexity Detect and Avoid for Autonomous Agents in Cluttered Environments
arxiv(2022)
摘要
In this paper, we propose a fast and low complexity detect and avoid (DAA) algorithm for unknown cluttered environments. Examples of such scenarios include but are not limited to various terrestrial missions e.g., search and rescue missions in jungles, and in space-exploration e.g., navigation of rovers on the Moon. The proposed method is based on the concept of artificial potential fields (APF) in which the target is attractive while the obstacles are repulsive to the mobile agent. To the original APF algorithm (by Khatib), we propose two major updates which significantly improve the performance of DAA using APF. First, we propose to improve an existing classical method that replaces the gradient descent optimization of the potential field cost function on a continuous domain with a combinatorial optimization on a set of predefined points (called bacteria points) around the agent's current location. Our proposition includes an adaptive hyperparameter that changes the value of the potential function associated to each bacteria point based on the current environmental measurements. Our proposed solution improves the navigation performance in terms of convergence to the target at the expense of minimal increase in computational complexity. Second, we propose an improved potential field cost function of the bacteria points by introducing a new branching cost function which further improves the navigation performance. The algorithms were tested on a set of Monte Carlo simulation trials where the environment changes for each trial. Our simulation results show 25% lower navigation time and around 200% higher success rate compared to the conventional potential field method.
更多查看译文
AI 理解论文
溯源树
样例
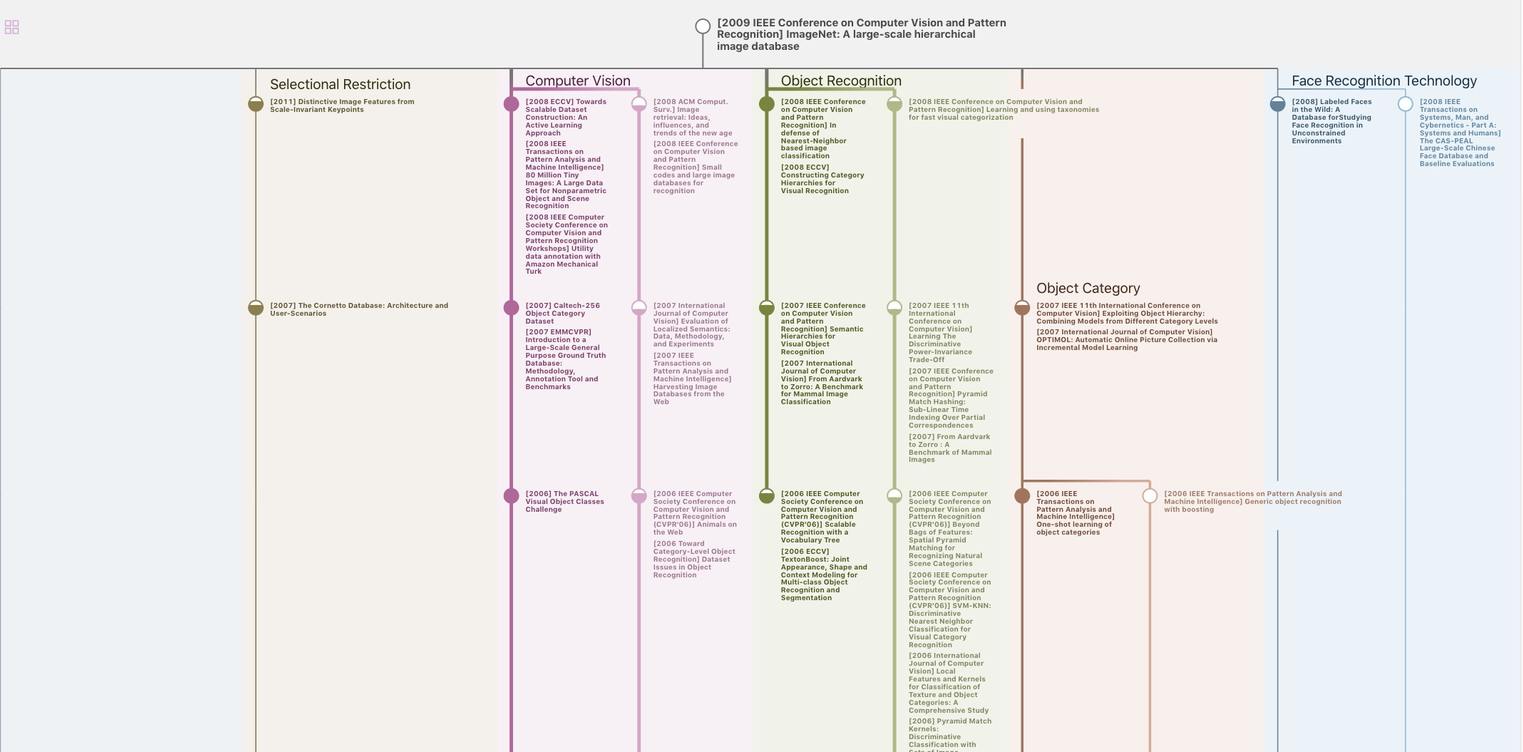
生成溯源树,研究论文发展脉络
Chat Paper
正在生成论文摘要