Combining noisy well data and expert knowledge in a Bayesian calibration of a flow model under uncertainties: an application to solute transport in the Ticino basin
arxiv(2023)
摘要
Groundwater flow modeling is commonly used to calculate groundwater heads, estimate groundwater flow paths and travel times, and provide insights into solute transport processes within an aquifer. However, the values of input parameters that drive groundwater flow models are often highly uncertain due to subsurface heterogeneity and geologic complexity in combination with lack of measurements/unreliable measurements. This uncertainty affects the accuracy and reliability of model outputs. Therefore, parameters’ uncertainty must be quantified before adopting the model as an engineering tool. In this study, we model the uncertain parameters as random variables and use a Bayesian inversion approach to obtain a posterior, data-informed, probability density function (pdf) for them: in particular, the likelihood function we consider takes into account both well measurements and our prior knowledge about the extent of the springs in the domain under study. To keep the modelistic and computational complexities under control, we assume Gaussianity of the posterior pdf of the parameters. To corroborate this assumption, we run an identifiability analysis of the model: we apply the inversion procedure to several sets of synthetic data polluted by increasing levels of noise, and we determine at which levels of noise we can effectively recover the “true value” of the parameters. We then move to real well data (coming from the Ticino River basin, in northern Italy, and spanning a month in summer 2014), and use the posterior pdf of the parameters as a starting point to perform an uncertainty quantification analysis on groundwater travel-time distributions.
更多查看译文
关键词
Bayesian inversion, MODFLOW, MODPATH, Travel time distribution, Uncertainty quantification, Model validation
AI 理解论文
溯源树
样例
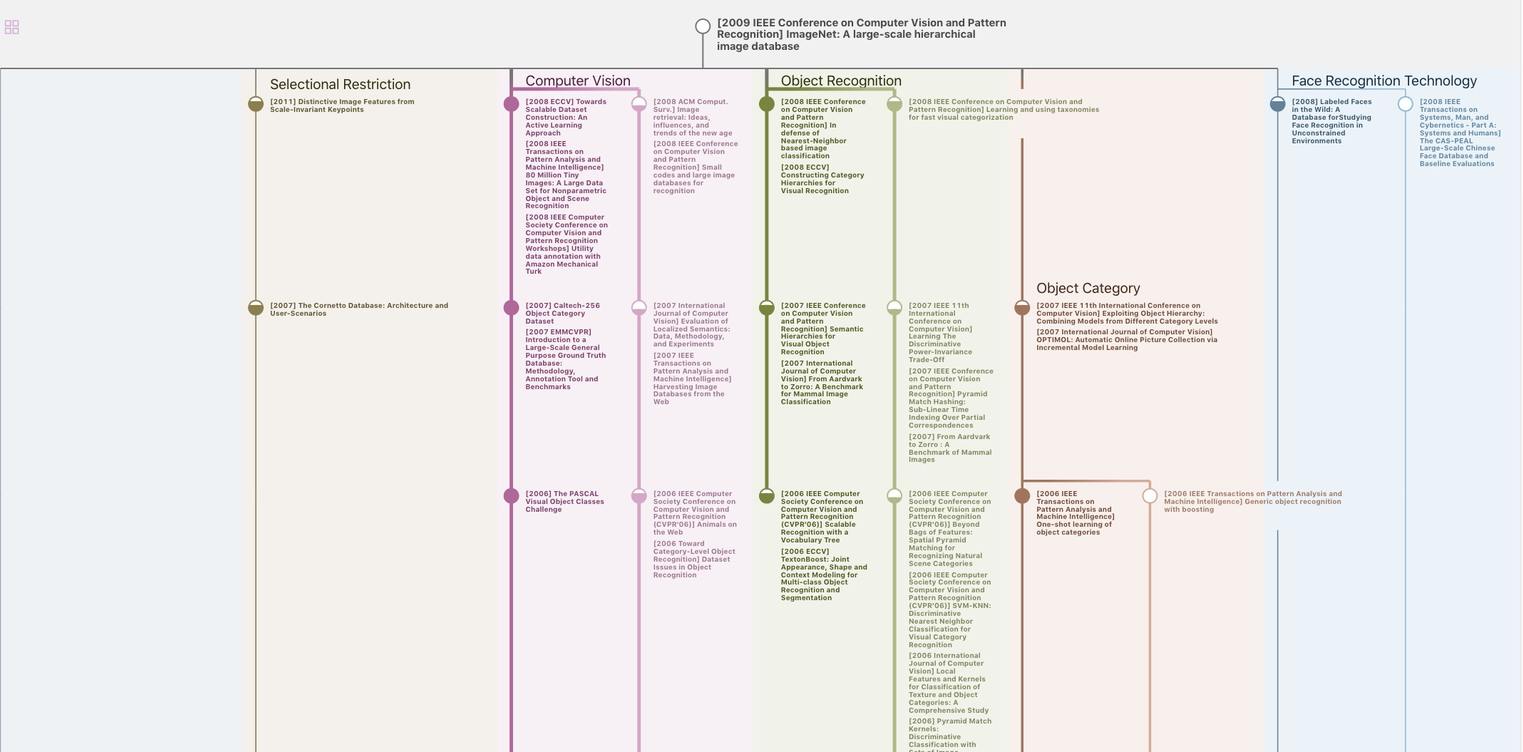
生成溯源树,研究论文发展脉络
Chat Paper
正在生成论文摘要