Learning to Optimize Permutation Flow Shop Scheduling via Graph-Based Imitation Learning
AAAI 2024(2024)
摘要
The permutation flow shop scheduling (PFSS), aiming at finding the optimal permutation of jobs, is widely used in manufacturing systems. When solving large-scale PFSS problems, traditional optimization algorithms such as heuristics could hardly meet the demands of both solution accuracy and computational efficiency, thus learning-based methods have recently garnered more attention. Some work attempts to solve the problems by reinforcement learning methods, which suffer from slow convergence issues during training and are still not accurate enough regarding the solutions. To that end, we propose to train the model via expert-driven imitation learning, which accelerates convergence more stably and accurately. Moreover, in order to extract better feature representations of input jobs, we incorporate the graph structure as the encoder. The extensive experiments reveal that our proposed model obtains significant promotion and presents excellent generalizability in large-scale problems with up to 1000 jobs. Compared to the state-of-the-art reinforcement learning method, our model's network parameters are reduced to only 37% of theirs, and the solution gap of our model towards the expert solutions decreases from 6.8% to 1.3% on average. The code is available at: https://github.com/longkangli/PFSS-IL.
更多查看译文
关键词
PRS: Scheduling,PRS: Planning/Scheduling and Learning
AI 理解论文
溯源树
样例
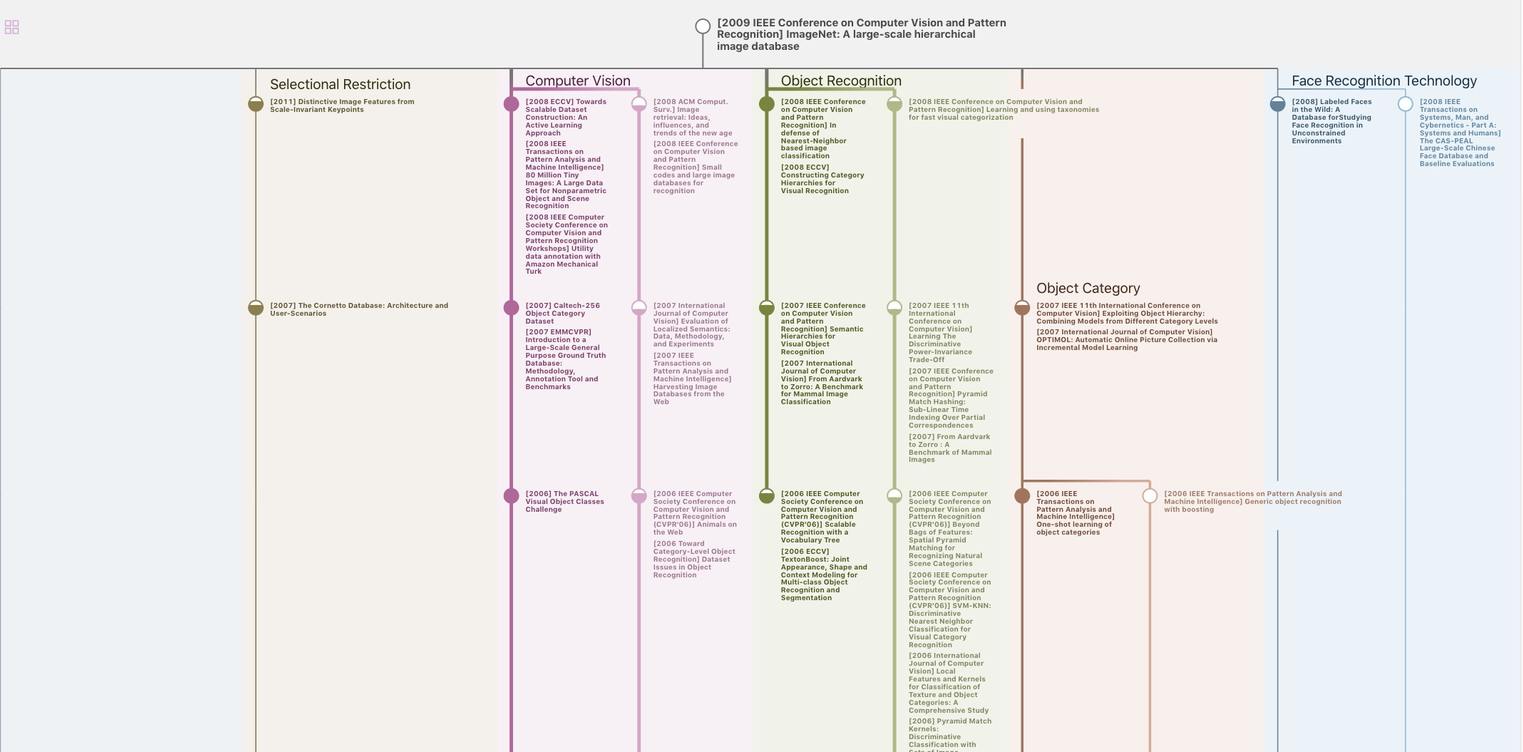
生成溯源树,研究论文发展脉络
Chat Paper
正在生成论文摘要