Wespeaker: A Research and Production oriented Speaker Embedding Learning Toolkit
arxiv(2022)
摘要
Speaker modeling is essential for many related tasks, such as speaker recognition and speaker diarization. The dominant modeling approach is fixed-dimensional vector representation, i.e., speaker embedding. This paper introduces a research and production oriented speaker embedding learning toolkit, Wespeaker. Wespeaker contains the implementation of scalable data management, state-of-the-art speaker embedding models, loss functions, and scoring back-ends, with highly competitive results achieved by structured recipes which were adopted in the winning systems in several speaker verification challenges. The application to other downstream tasks such as speaker diarization is also exhibited in the related recipe. Moreover, CPU- and GPU-compatible deployment codes are integrated for production-oriented development. The toolkit is publicly available at https://github.com/wenet-e2e/wespeaker.
更多查看译文
关键词
Wespeaker,Speaker embedding,Speaker verification,Speaker diarization
AI 理解论文
溯源树
样例
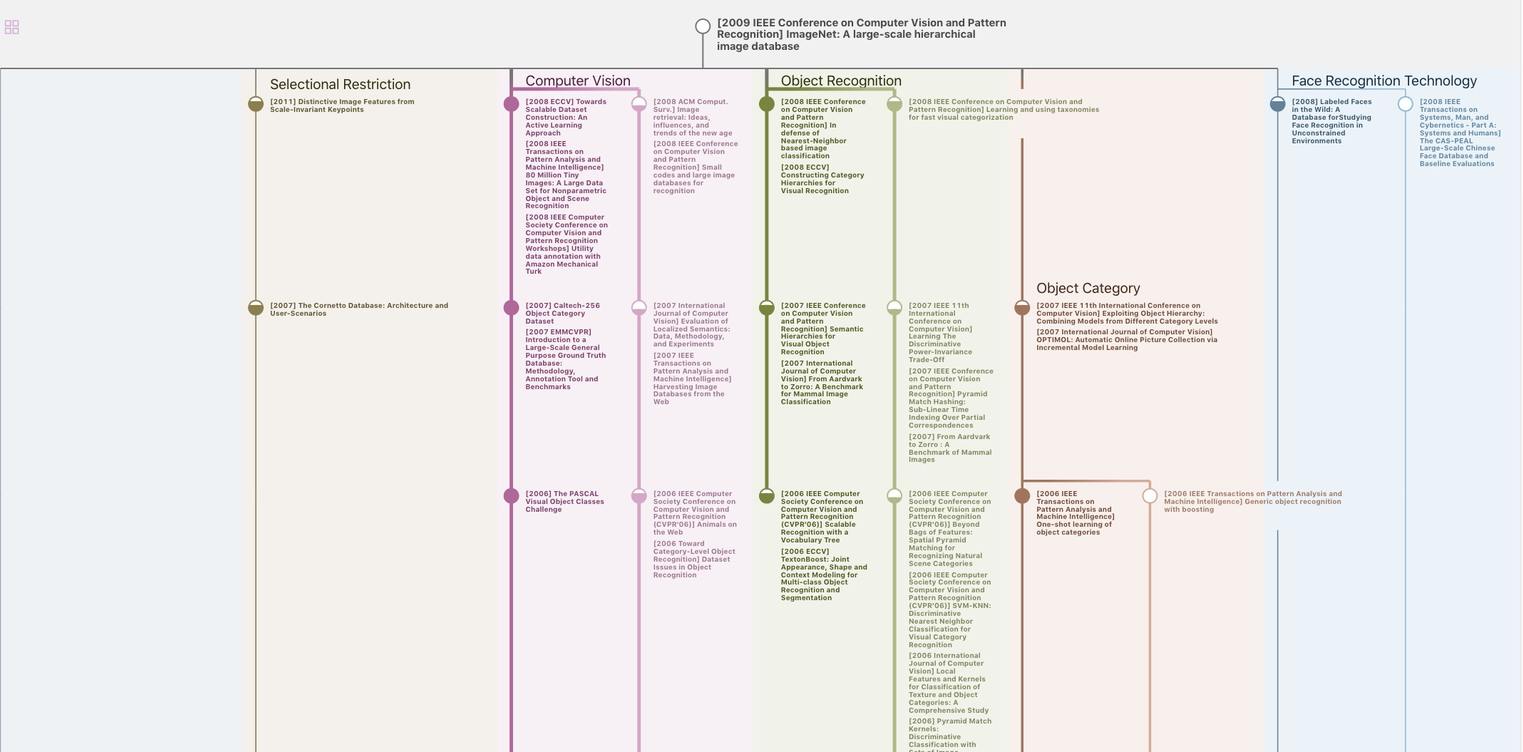
生成溯源树,研究论文发展脉络
Chat Paper
正在生成论文摘要