Saliency Can Be All You Need in Contrastive Self-supervised Learning.
ISVC (2)(2022)
Abstract
We propose an augmentation policy for Contrastive Self-Supervised Learning (SSL) in the form of an already established Salient Image Segmentation technique entitled Global Contrast based Salient Region Detection. This detection technique, which had been devised for unrelated Computer Vision tasks, was empirically observed to play the role of an augmentation facilitator within the SSL protocol. This observation is rooted in our practical attempts to learn, by SSL-fashion, aerial imagery of solar panels, which exhibit challenging boundary patterns. Upon the successful integration of this technique on our problem domain, we formulated a generalized procedure and conducted a comprehensive, systematic performance assessment with various Contrastive SSL algorithms subject to standard augmentation techniques. This evaluation, which was conducted across multiple datasets, indicated that the proposed technique indeed contributes to SSL. We hypothesize whether salient image segmentation may suffice as the only augmentation policy in Contrastive SSL when treating downstream segmentation tasks.
MoreTranslated text
Key words
learning,self-supervised
AI Read Science
Must-Reading Tree
Example
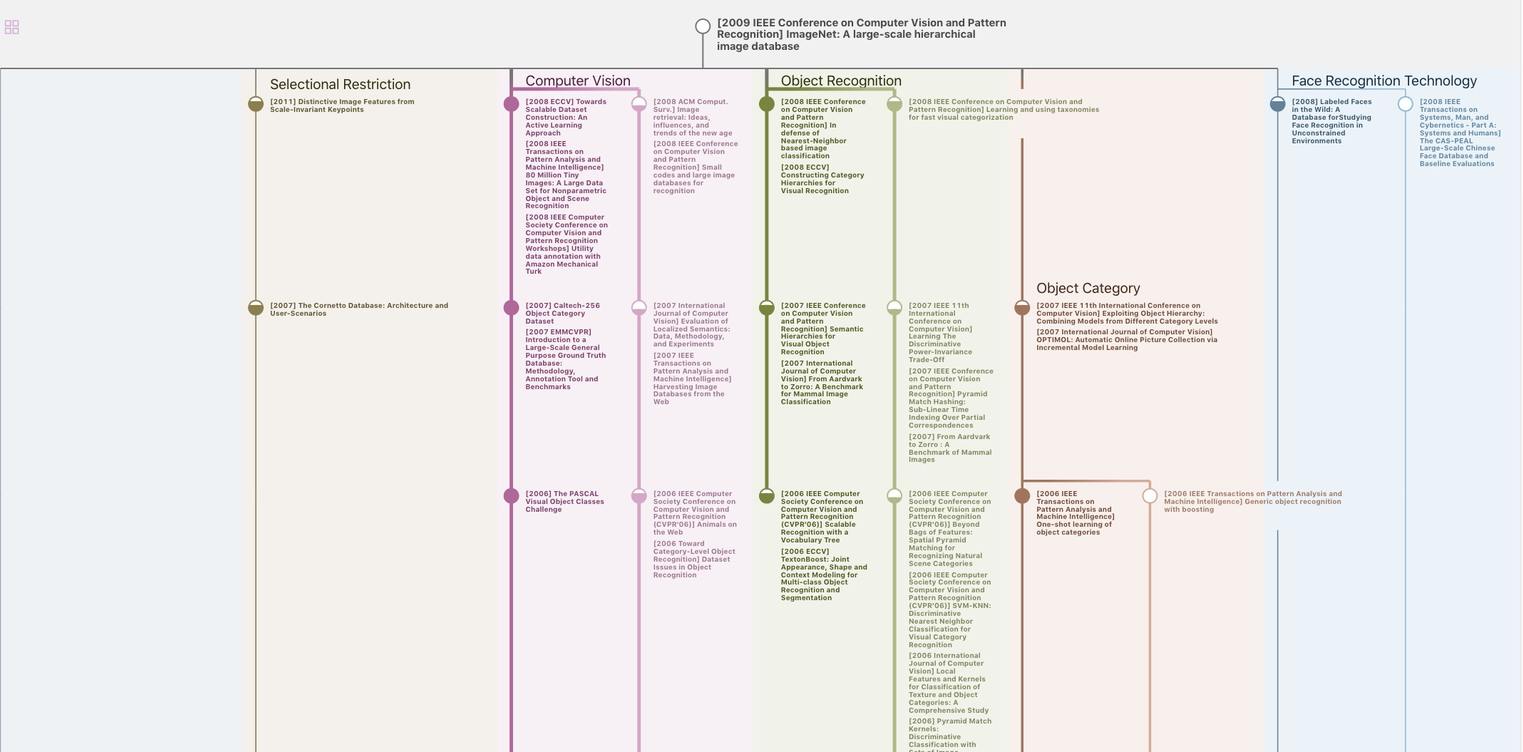
Generate MRT to find the research sequence of this paper
Chat Paper
Summary is being generated by the instructions you defined