Estimating oil recovery factor using machine learning: Applications of XGBoost classification
arxiv(2022)
摘要
In petroleum engineering, it is essential to determine the ultimate recovery factor, RF, particularly before exploitation and exploration. However, accurately estimating requires data that is not necessarily available or measured at early stages of reservoir development. We, therefore, applied machine learning (ML), using readily available features, to estimate oil RF for ten classes defined in this study. To construct the ML models, we applied the XGBoost classification algorithm. Classification was chosen because recovery factor is bounded from 0 to 1, much like probability. Three databases were merged, leaving us with four different combinations to first train and test the ML models and then further evaluate them using an independent database including unseen data. The cross-validation method with ten folds was applied on the training datasets to assess the effectiveness of the models. To evaluate the accuracy and reliability of the models, the accuracy, neighborhood accuracy, and macro averaged f1 score were determined. Overall, results showed that the XGBoost classification algorithm could estimate the RF class with reasonable accuracies as high as 0.49 in the training datasets, 0.34 in the testing datasets and 0.2 in the independent databases used. We found that the reliability of the XGBoost model depended on the data in the training dataset meaning that the ML models were database dependent. The feature importance analysis and the SHAP approach showed that the most important features were reserves and reservoir area and thickness.
更多查看译文
AI 理解论文
溯源树
样例
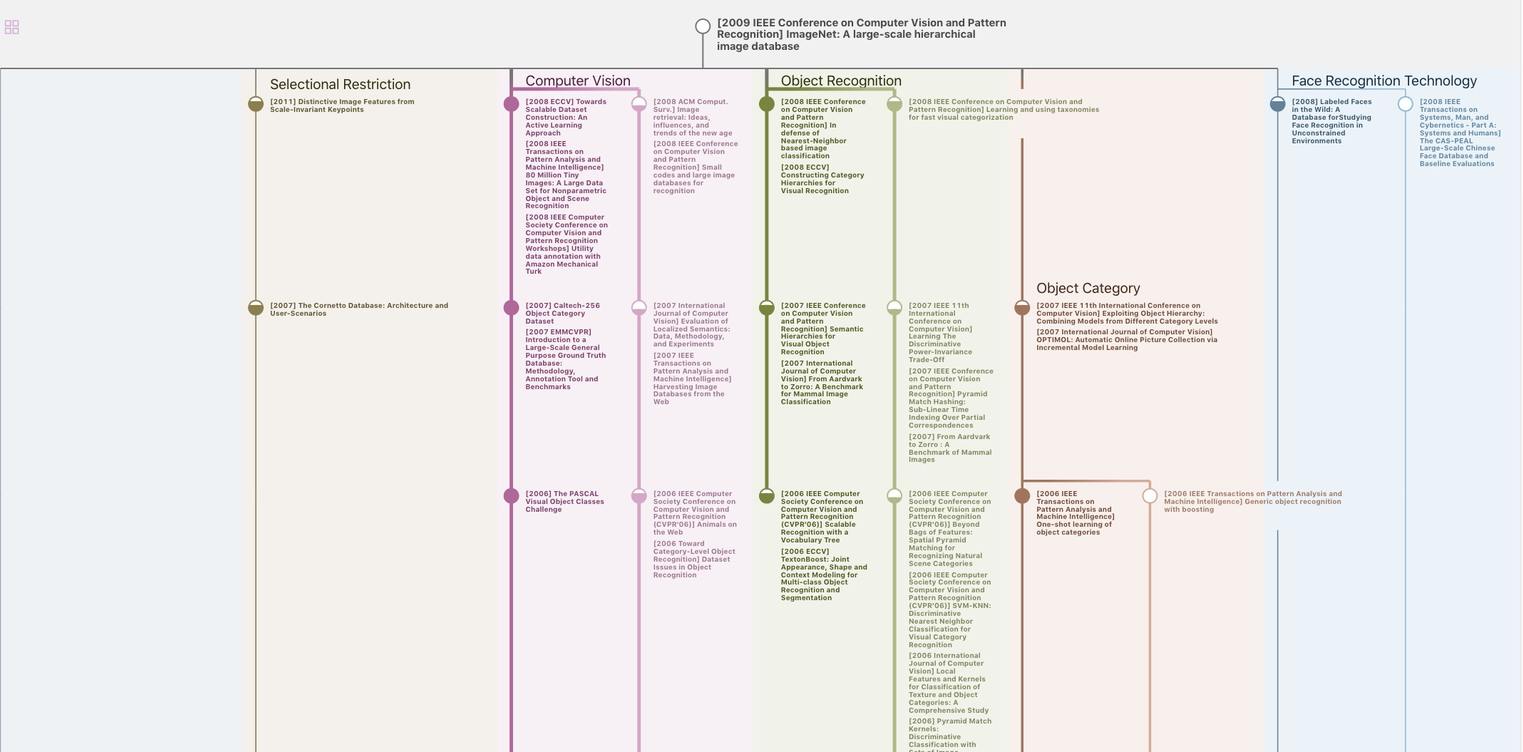
生成溯源树,研究论文发展脉络
Chat Paper
正在生成论文摘要