A federated multi-agent deep reinforcement learning for vehicular fog computing
The Journal of Supercomputing(2022)
摘要
Vehicular fog computing is an emerging paradigm for delay-sensitive computations. In this highly dynamic resource-sharing environment, optimal offloading decision for effective resource utilization is a challenging task. In recent years, deep reinforcement learning has emerged as an effective approach for dealing with resource allocation problems because of its self-adapting nature in a large state space scenario. However, due to high mobility and rapid changes in the network topology cause fluctuating task arrival rate. Similarly, the data sharing between the vehicles and the fog nodes raises a variety of security and privacy concerns. Therefore, the proposed system is based on local and global model training approaches. In this paper, we propose a federated multi-agent deep reinforcement learning solution that efficiently learns task-offloading decisions at multiple tiers i.e. locally and globally. The proposed work results in fast convergence due to its collaborative learning model among vehicles and fog servers. The local model runs at the vehicular nodes, and the global model runs at the fog servers. To reduce network overhead, the models are learned locally; thus, limited information is shared across the network this reduces the communication overhead and improves the privacy of the agents. The proposed system is compared with the greedy and stochastic approaches in terms of residence times, cost, delivery rate, and utilization ratio. We observed that the proposed approach has significantly reduced the task residence time, end-to-end delay and overall system cost.
更多查看译文
关键词
Deep reinforcement learning,Federated learning,Computation offloading,A3C,Advantage function,Residence time
AI 理解论文
溯源树
样例
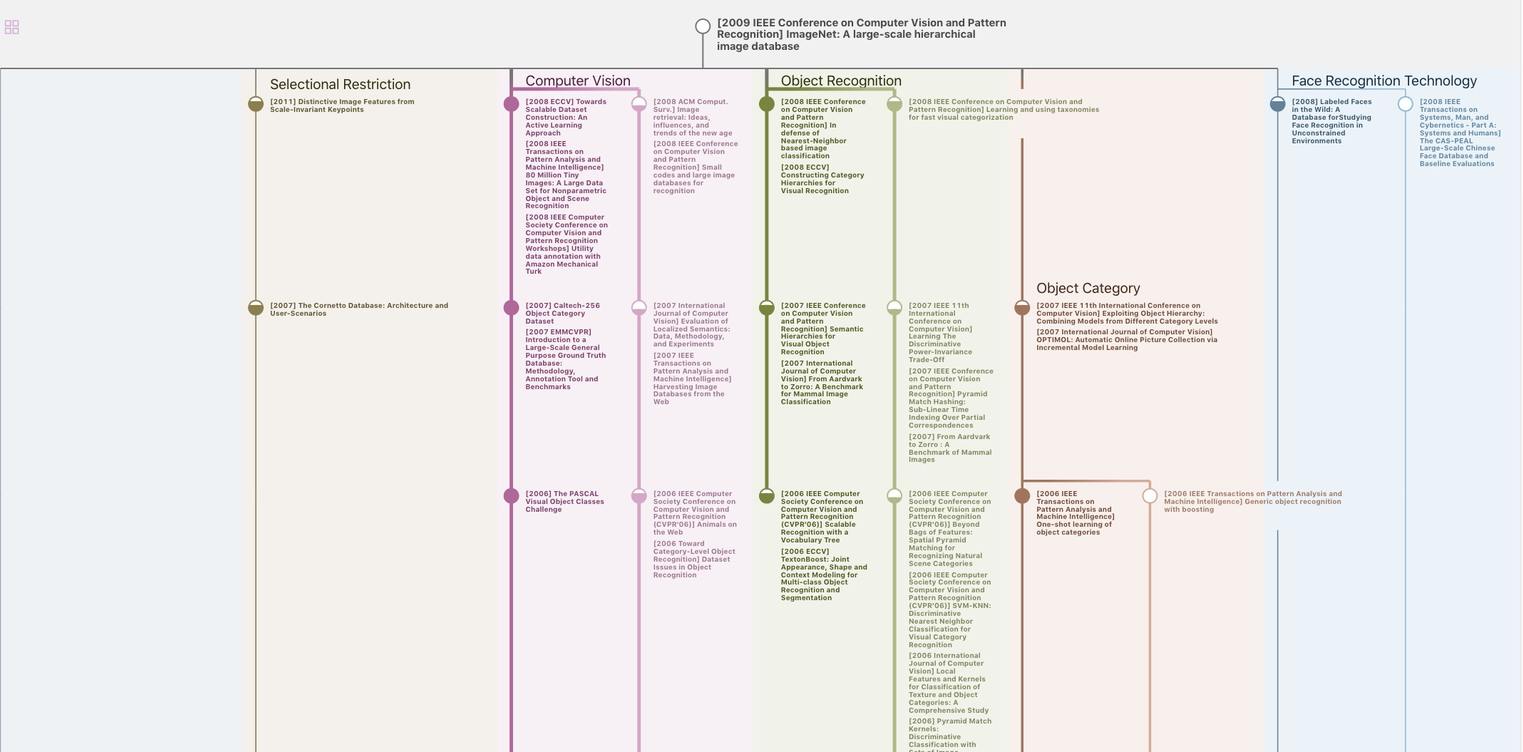
生成溯源树,研究论文发展脉络
Chat Paper
正在生成论文摘要