Regulatory Genes Through Robust-SNR for Binary Classification Within Functional Genomics Experiments
Computers, Materials & Continua(2023)
摘要
The current study proposes a novel technique for feature selection by inculcating robustness in the conventional Signal to noise Ratio (SNR). The proposed method utilizes the robust measures of location i.e., the "Median " as well as the measures of variation i.e., "Median absolute deviation (MAD) and Interquartile range (IQR) " in the SNR. By this way, two independent robust signal-to-noise ratios have been proposed. The proposed method selects the most informative genes/features by combining the mini-mum subset of genes or features obtained via the greedy search approach with top-ranked genes selected through the robust signal-to-noise ratio (RSNR). The results obtained via the proposed method are compared with well-known gene/feature selection methods on the basis of performance metric i.e., classification error rate. A total of 5 gene expression datasets have been used in this study. Different subsets of informative genes are selected by the proposed and all the other methods included in the study, and their efficacy in terms of classification is investigated by using the classifier models such as support vector machine (SVM), Random forest (RF) and k-nearest neighbors (k-NN). The results of the analysis reveal that the proposed method (RSNR) produces minimum error rates than all the other competing feature selection methods in majority of the cases. For further assessment of the method, a detailed simulation study is also conducted.
更多查看译文
关键词
Median absolute deviation (MAD),classification,feature selection,high dimensional gene expression datasets,signal to noise ratio
AI 理解论文
溯源树
样例
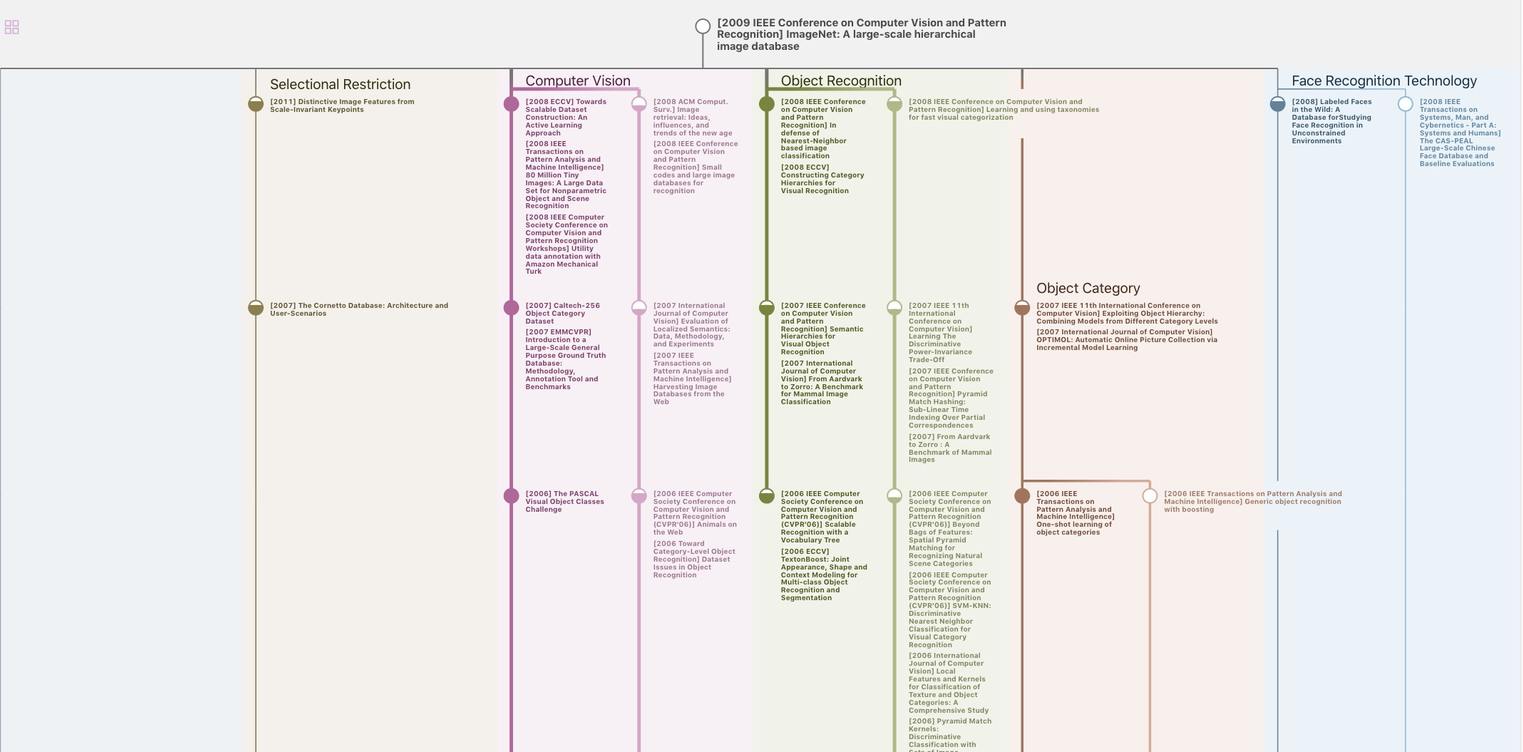
生成溯源树,研究论文发展脉络
Chat Paper
正在生成论文摘要