From driving behavior to energy consumption: A novel method to predict the energy consumption of electric bus
Energy(2022)
摘要
Accurate real-time energy consumption prediction of electric buses (EBs) is essential for bus operation and management, which can effectively mitigate the driving range anxiety while reducing the operation cost simultaneously. This paper presents a machine learning-based energy consumption prediction method for EB, which combines driving data with road characteristics data (such as road type), traffic condition (such as peak hour), and meteorology data (such as temperature). The importance of driving behavior features affecting energy consumption is quantitatively revealed by the novel Shapley additive explanation (SHAP). Given the road characteristics, traffic condition and meteorology information, a Long Short-Term Memory (LSTM) network is then used to predict driving microscopic parameters, including speed, acceleration, gas pedal position and brake pedal position. Finally, the instantaneous electricity consumption is predicted using an Extreme Gradient Boosting (XGBoost) model based on the predicted values from the LSTM. The results show that the proposed LSTM-XGBoost model with accurate time series prediction and regression is powerful for efficiently fitting the complex volatility of energy consumption. Moreover, the proposed model chain outperforms other model combinations (such as artificial neural networks and conventional regression methods) in terms of root mean squared error (RMSE = 0.079), mean absolute error (MAE = 0.086) and R-square (R2 = 0.814).
更多查看译文
关键词
Electric bus,Driving behavior,Energy consumption prediction,Long-short term memory network (LSTM),Extreme gradient boosting model (XGBoost)
AI 理解论文
溯源树
样例
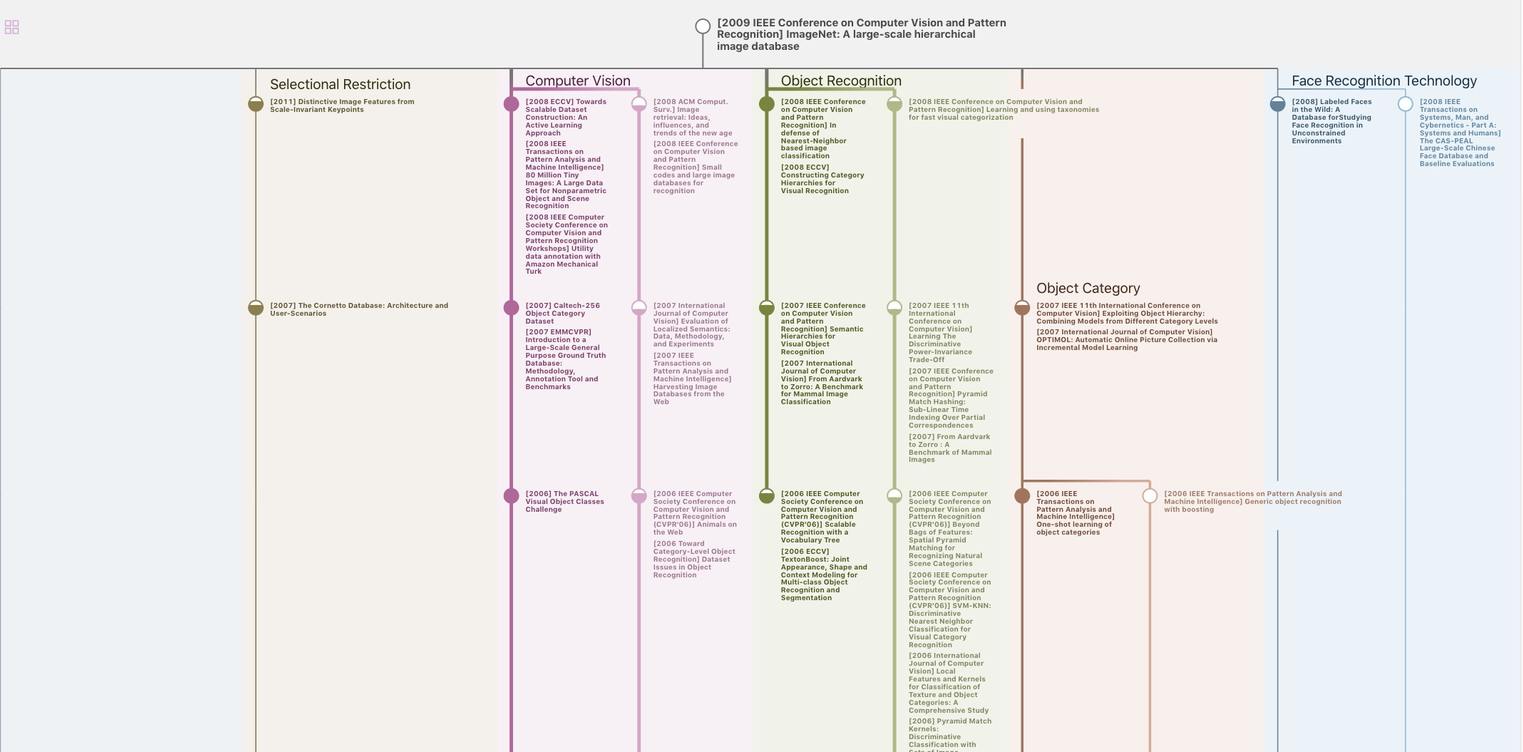
生成溯源树,研究论文发展脉络
Chat Paper
正在生成论文摘要