IB-U-Nets: Improving medical image segmentation tasks with 3D Inductive Biased kernels
arxiv(2022)
摘要
Despite the success of convolutional neural networks for 3D medical-image segmentation, the architectures currently used are still not robust enough to the protocols of different scanners, and the variety of image properties they produce. Moreover, access to large-scale datasets with annotated regions of interest is scarce, and obtaining good results is thus difficult. To overcome these challenges, we introduce IB-U-Nets, a novel architecture with inductive bias, inspired by the visual processing in vertebrates. With the 3D U-Net as the base, we add two 3D residual components to the second encoder blocks. They provide an inductive bias, helping U-Nets to segment anatomical structures from 3D images with increased robustness and accuracy. We compared IB-U-Nets with state-of-the-art 3D U-Nets on multiple modalities and organs, such as the prostate and spleen, using the same training and testing pipeline, including data processing, augmentation and cross-validation. Our results demonstrate the superior robustness and accuracy of IB-U-Nets, especially on small datasets, as is typically the case in medical-image analysis. IB-U-Nets source code and models are publicly available.
更多查看译文
关键词
medical image segmentation tasks,biased kernels,medical image,3d inductive
AI 理解论文
溯源树
样例
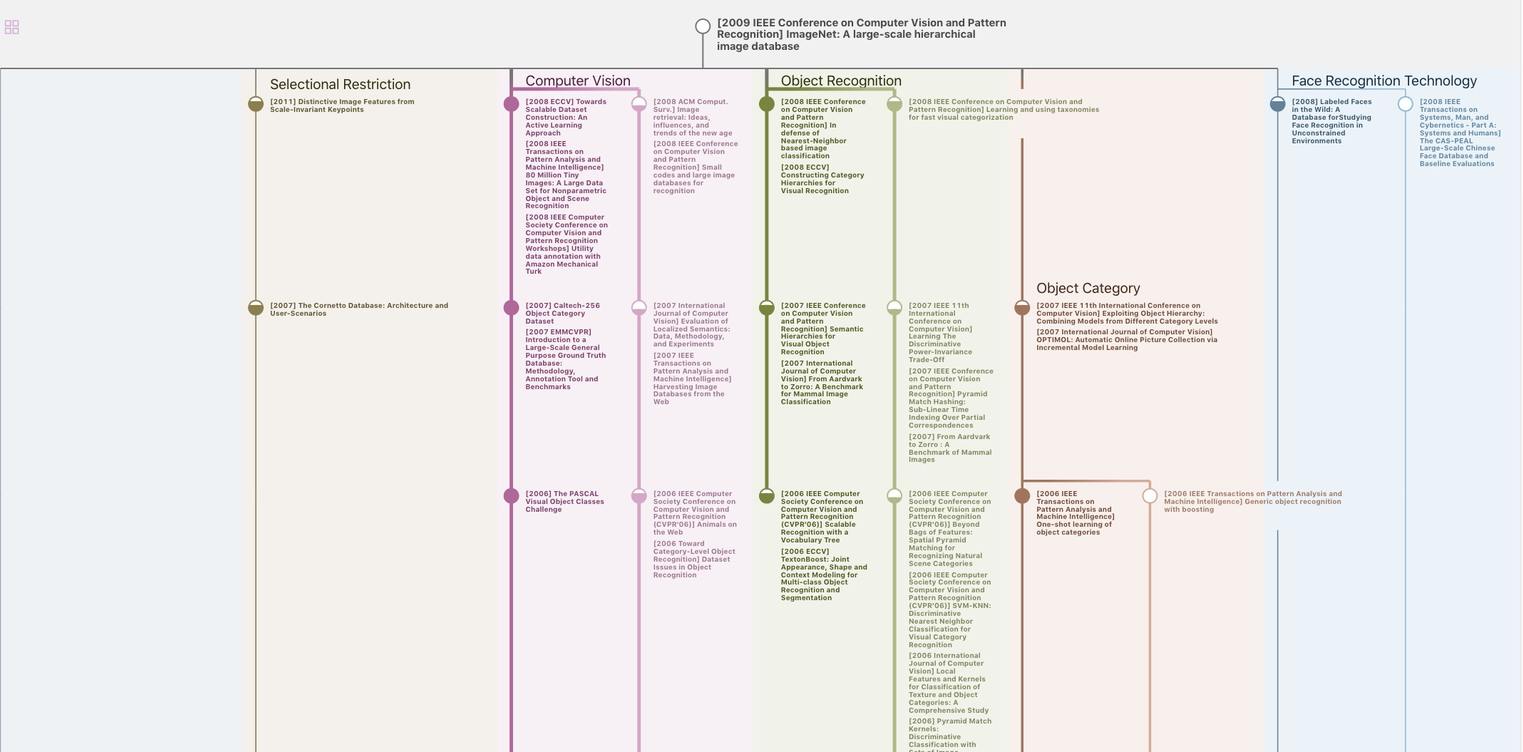
生成溯源树,研究论文发展脉络
Chat Paper
正在生成论文摘要