A Business-Oriented Feature Selection Method for Enhancing Machine Learning Based Digital Tools Adoption in the Energy Industry
Day 2 Tue, November 01, 2022(2022)
Abstract
Abstract Objectives/Scope To ensure that predictive models based on Machine Learning (ML) techniques can be fully exploited by energy plant operators, these should be trained on "actionable" features, i.e., a set of features that are explainable and on which operators can directly act to take mitigative and/or preventive actions. To this aim, we have developed a business-oriented feature selection approach which identifies actionable features while optimizing the model predictive capability at the same time. Methods, Procedures, Process We have developed a solution which frames the feature selection step as a Multi-Objective Optimization (MOO) problem which relies on genetic algorithms as search engine. The MOO returns a set of optimal feature subsets referred to as Pareto optimal front on which the best compromise solution in terms of model performance and ‘actionability’ must be chosen. For selecting an optimal solution from the Pareto optimal front, the TOPSIS method has been embraced which automatically ranks the candidate solutions based on their relative importance that the users assign to the two objectives to be optimized. Results, Observations, Conclusions The proposed methodology has been successfully applied to a real case study concerning cracking furnaces of a petrochemical plant. The optimized predictive model resulting from the application of the developed feature selection strategy has shown to be properly suited for plant operators allowing them to easily derive insights and properly take actions based on the model output. Further, due to the agnostic nature of the proposed methodology, this can be easily extended and applied to other Energy case studies where "actionability" is a driving key for the successful adoption of digital tools based on ML models. Novel/Additive Information To the best of Authors knowledge, the proposed approach which embeds the "actionability" property of a feature as criterium in the feature selection step and automates the process of selecting an optimal solution through the TOPSIS method, is novel from a methodological point of view in the field of machine learning applied to energy transition use cases.
MoreTranslated text
Key words
feature selection method,machine learning,selection method,business-oriented
AI Read Science
Must-Reading Tree
Example
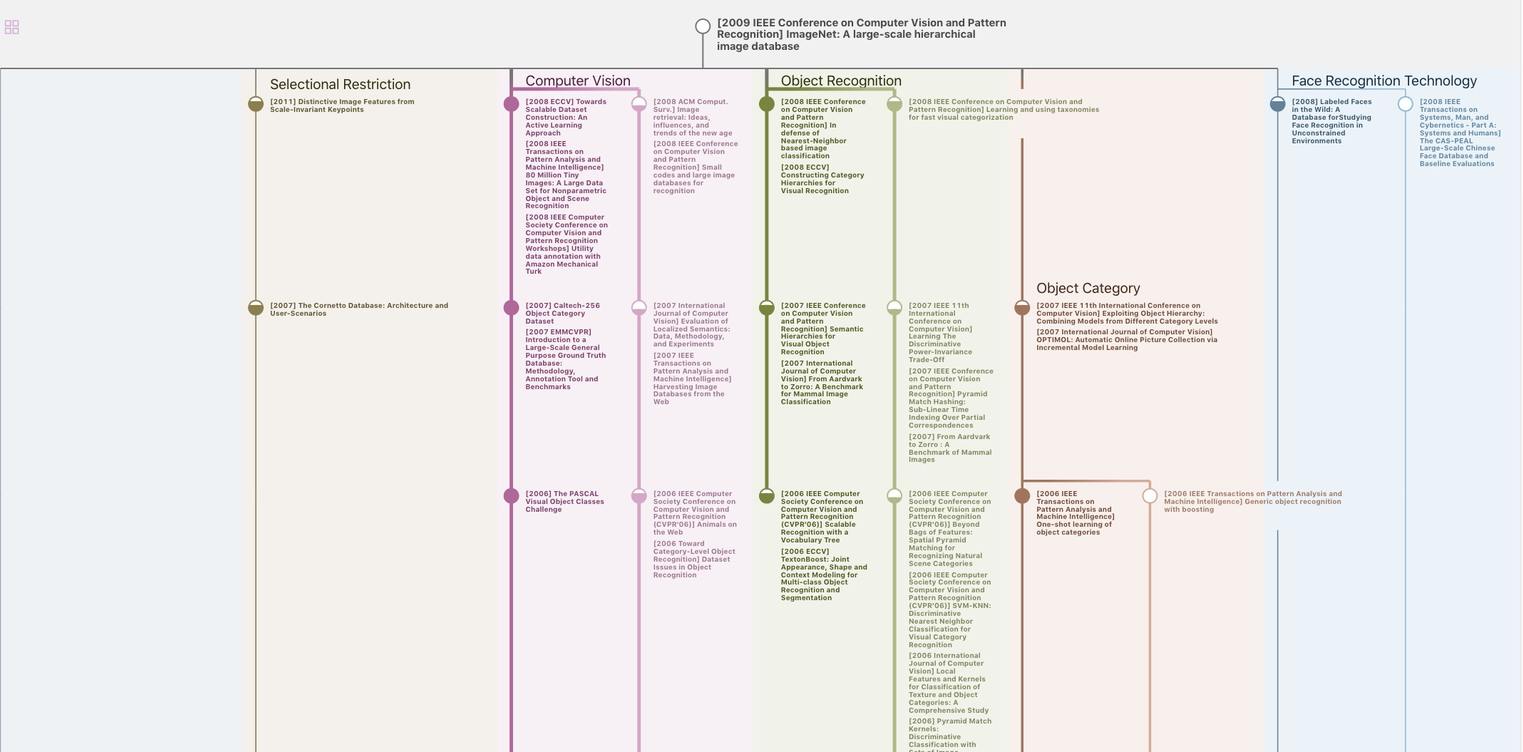
Generate MRT to find the research sequence of this paper
Chat Paper
Summary is being generated by the instructions you defined