Assimilation of Deep Learning and Machine Learning Schemes into a Remote Sensing-Incorporated Crop Model to Simulate Barley and Wheat Productivities
REMOTE SENSING(2022)
Abstract
Deep learning (DL) and machine learning (ML) procedures are prevailing data-driven schemes capable of advancing crop-modelling practices that assimilate these techniques into a mathematical crop model. A DL or ML modelling scheme can effectively represent complicated algorithms. This study reports on an advanced fusion methodology for evaluating the leaf area index (LAI) of barley and wheat that employs remotely sensed information based on deep neural network (DNN) and ML regression approaches. We investigated the most appropriate ML regressors for exploring LAI estimations of barley and wheat through the relationships between the LAI values and four vegetation indices. After analysing ten ML regression models, we concluded that the gradient boost (GB) regressor most effectively estimated the LAI for both barley and wheat. Furthermore, the GB regressor outperformed the DNN regressor, with model efficiencies of 0.89 for barley and 0.45 for wheat. Additionally, we verified that it would be possible to simulate LAI using proximal and remote sensing data based on assimilating the DNN and ML regressors into a process-based mathematical crop model. In summary, we have demonstrated that if DNN and ML schemes are integrated into a crop model, they can facilitate crop growth and boost productivity monitoring.
MoreTranslated text
Key words
barley,crop model,deep neural network,integration,machine learning,wheat
AI Read Science
Must-Reading Tree
Example
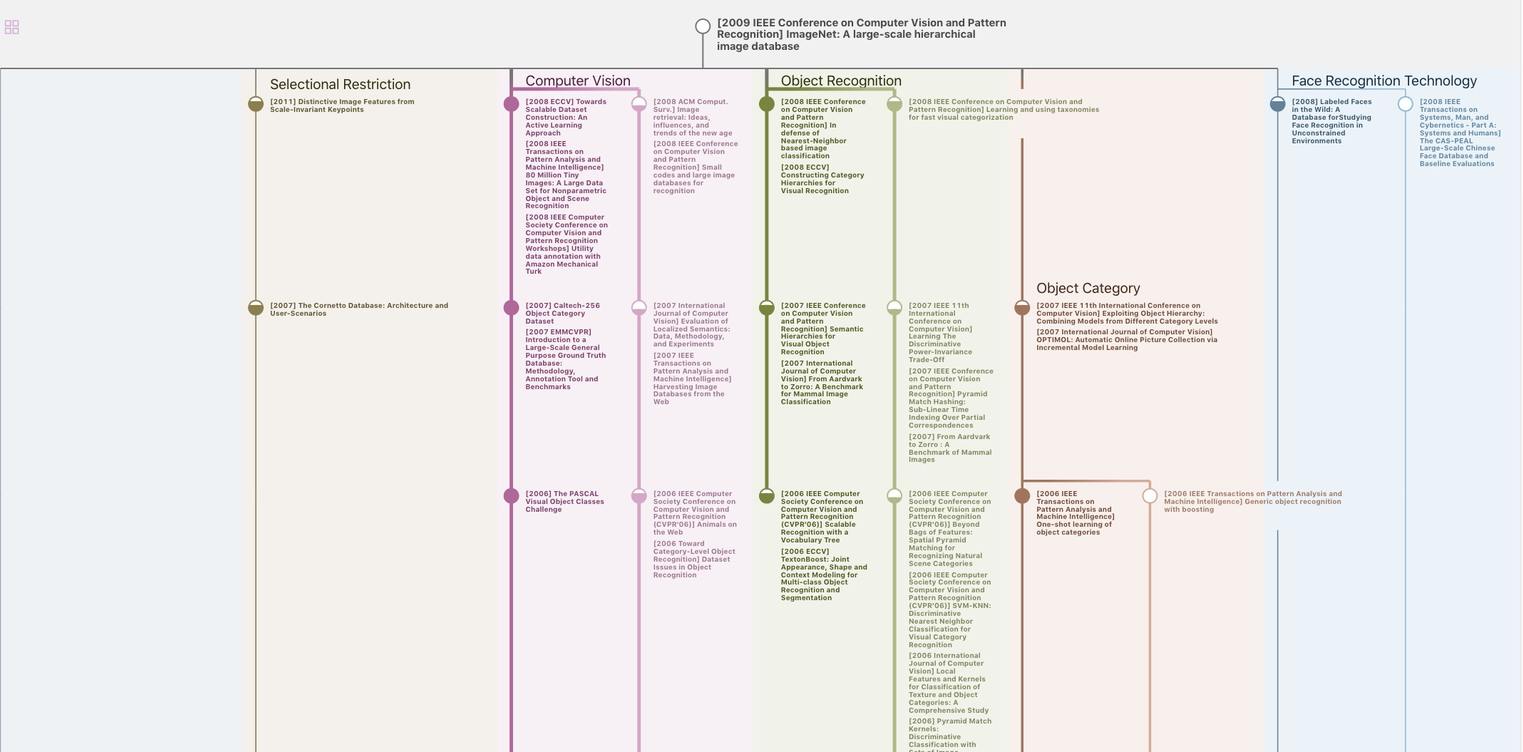
Generate MRT to find the research sequence of this paper
Chat Paper
Summary is being generated by the instructions you defined