Robust Prediction and Protein Selection with Adaptive PENSE.
Methods in molecular biology (Clifton, N.J.)(2023)
Abstract
Adaptive PENSE is a method that can be used to build models for predicting clinical outcomes from a small subset of a potentially large number of candidate proteins. Adaptive PENSE is designed to give reliable results under two common challenges often encountered in these kinds of studies: (1) the number of samples with known clinical outcome and proteomic data is small, while the number of candidate proteins is large and/or (2) proteomic data and the clinical outcome measurements suffer from data quality issues in a small fraction of samples. Even in the presence of these challenges, adaptive PENSE reliably identifies proteins relevant for prediction and estimates accurate predictive models. Adaptive PENSE is designed to be resilient to data quality issues in up to 50% of samples. Almost half of the samples could have aberrant values in the measured protein levels and clinical outcome values without causing severe detrimental effects to the estimated predictive model. The method is implemented as an R package and supports the user in the model selection process by automating most steps and providing diagnostic visualizations to guide the user. Users can choose among several predictive models to select the model with high prediction accuracy and an appropriate number of selected proteins.
MoreTranslated text
Key words
High-dimensional data,Linear regression,Prediction,Protein selection,Robust estimation
AI Read Science
Must-Reading Tree
Example
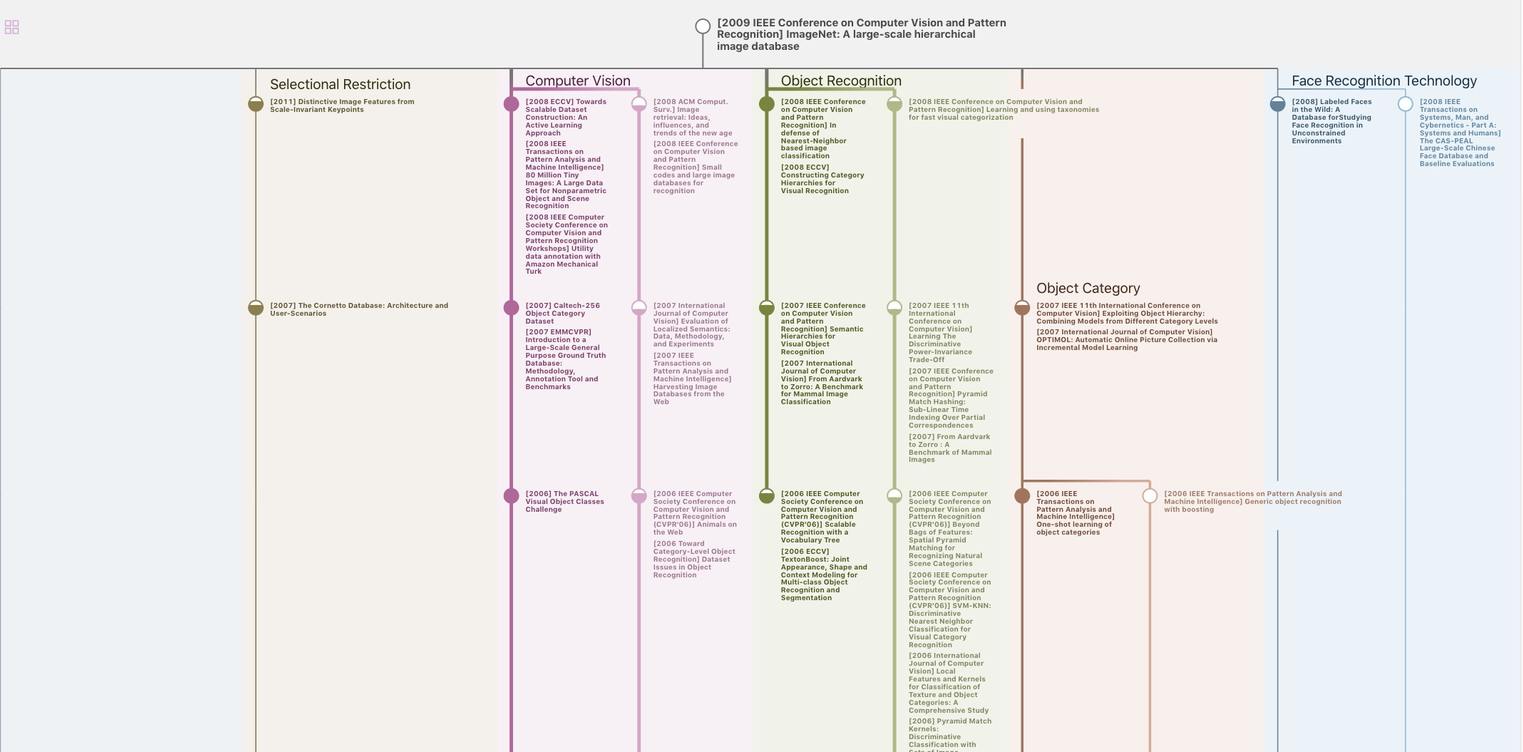
Generate MRT to find the research sequence of this paper
Chat Paper
Summary is being generated by the instructions you defined