Deep-Learning Approach for Fusarium Head Blight Detection in Wheat Seeds Using Low-Cost Imaging Technology
Agriculture(2022)
摘要
Modern techniques that enable high-precision and rapid identification/elimination of wheat seeds infected by Fusarium head blight (FHB) can help to prevent human and animal health risks while improving agricultural sustainability. Robust pattern-recognition methods, such as deep learning, can achieve higher precision in detecting infected seeds using more accessible solutions, such as ordinary RGB cameras. This study used different deep-learning approaches based on RGB images, combining hyperparameter optimization, and fine-tuning strategies with different pretrained convolutional neural networks (convnets) to discriminate wheat seeds of the TBIO Toruk cultivar infected by FHB. The models achieved an accuracy of 97% using a low-complexity design architecture with hyperparameter optimization and 99% accuracy in detecting FHB in seeds. These findings suggest the potential of low-cost imaging technology and deep-learning models for the accurate classification of wheat seeds infected by FHB. However, FHB symptoms are genotype-dependent, and therefore the accuracy of the detection method may vary depending on phenotypic variations among wheat cultivars.
更多查看译文
关键词
seed quality,convolutional neural networks,Triticum aestivum,Fusarium graminearum,RGB images,TBIO Toruk cultivar
AI 理解论文
溯源树
样例
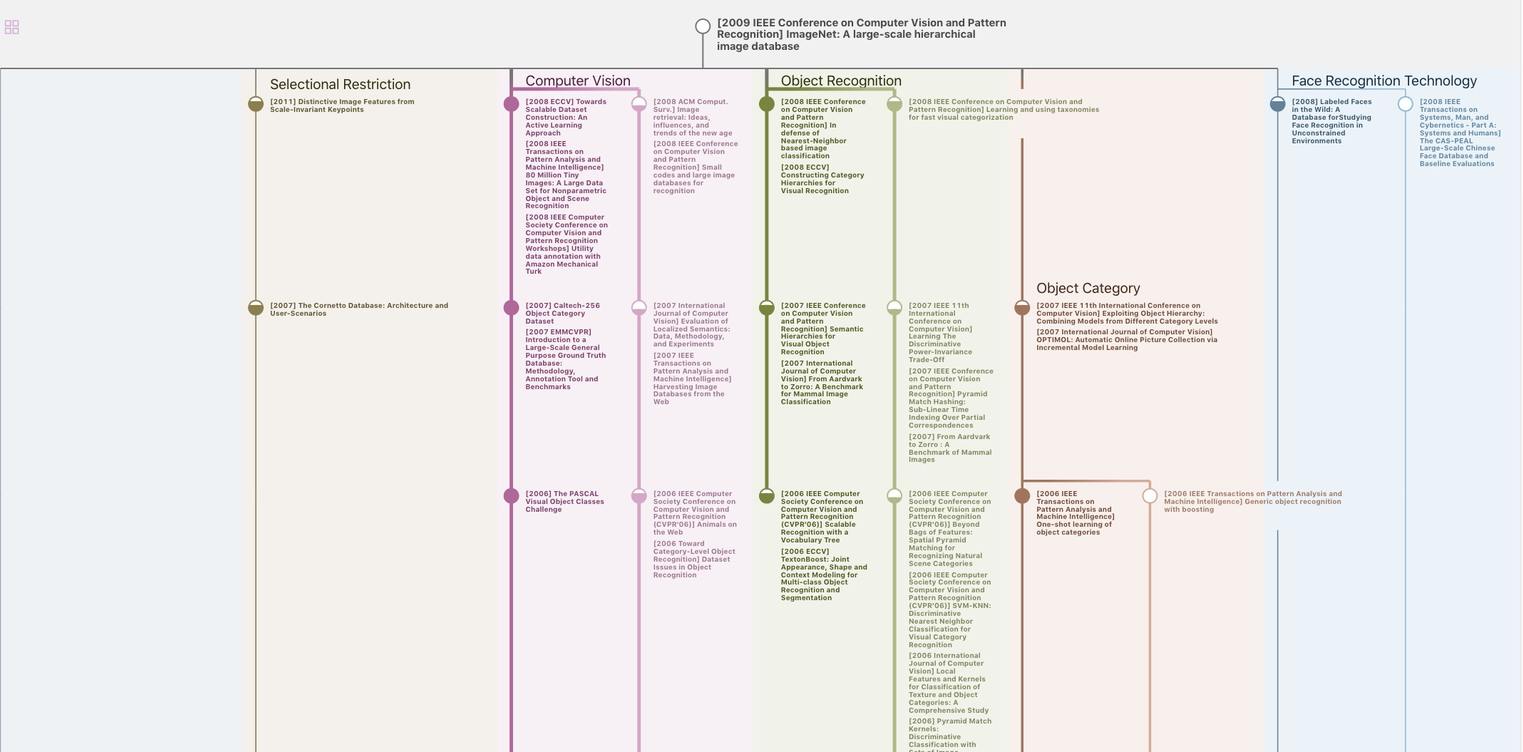
生成溯源树,研究论文发展脉络
Chat Paper
正在生成论文摘要