Representation and analysis of time-series data via deep embedding and visual exploration
Journal of Visualization(2022)
摘要
Time-series representation is fundamental for various analysis tasks on time-series data, such as similarity analysis, clustering, and classification. Traditional representation methods produce indistinguishable representations based on statistical information. Given the good performance of deep learning techniques in feature extraction, we apply it to the task of time-series data representation. However, such methods are usually evaluated only through a few quantitative metrics that reflect the limited model performance and lack further explanation. In this paper, we design TSRNet, a deep neural network containing two weight-sharing auto-encoders to learn representations effectively. To better analyze the representation, we design an interactive visual analytics system TSRVis in collaboration with seven experts from the fields of visual analytics and machine learning. Experimental evaluations on 18 datasets verify the effectiveness of TSRNet in terms of clustering accuracy. Three case studies demonstrate that TSRVis is practicable for assisting analysts to perform overview, comparison, and point-of-interest analyses and assess the validity of representations from multiple perspectives. Graphical abstract
更多查看译文
关键词
Visual analytics, Deep learning, Time-series data
AI 理解论文
溯源树
样例
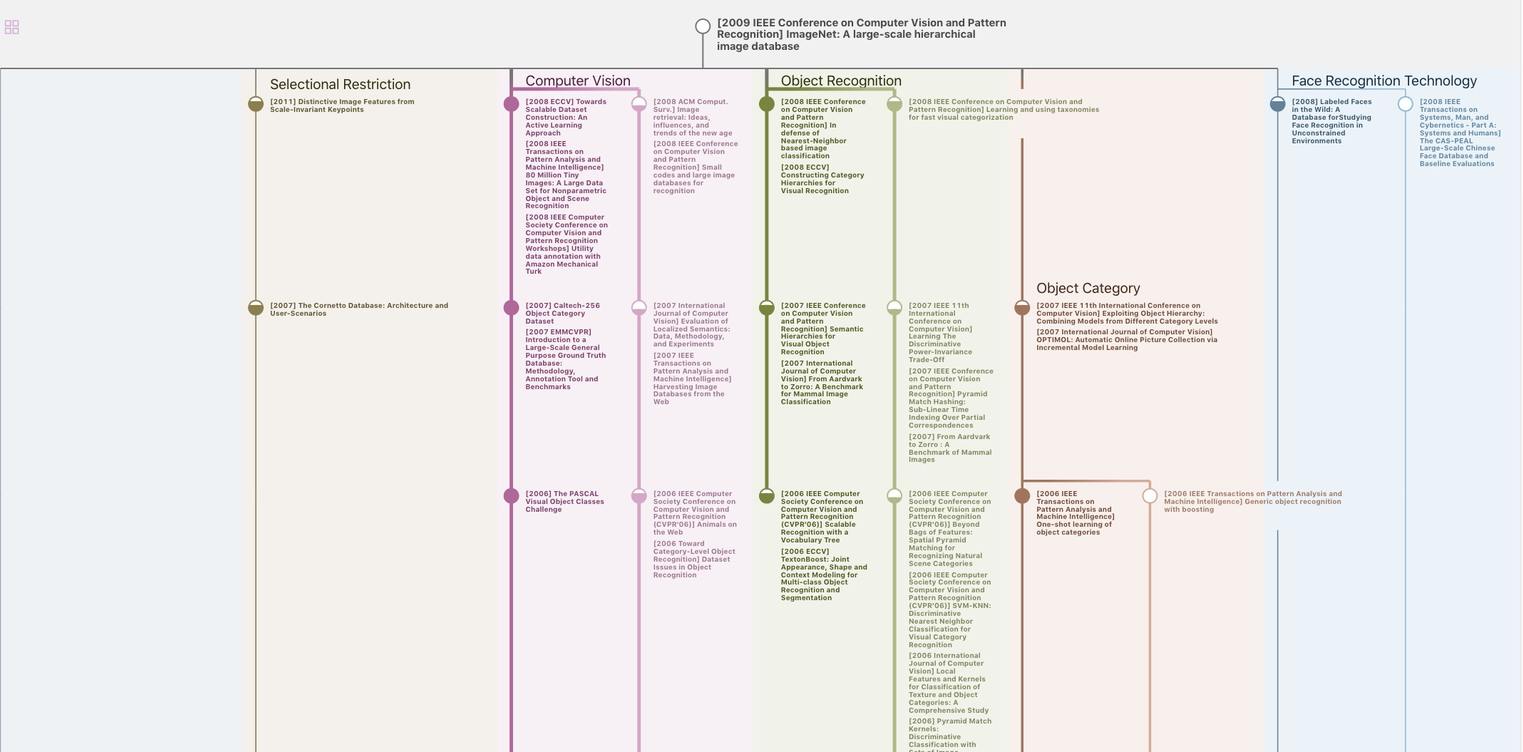
生成溯源树,研究论文发展脉络
Chat Paper
正在生成论文摘要