Taking full advantage of ‘low-quality’ datasets in watershed modeling and management: From a perspective of parameter calibration
SSRN Electronic Journal(2024)
摘要
The quality of calibration datasets is critical for establishing well-calibrated models for reliable decision-making support. However, the analysis of the influence of calibration dataset quality and the discussion on how to use flawed and/or incomplete datasets are still far from sufficient. An evaluation framework for the impact of model calibration data on parameter identifiability, sensitivity, and uncertainty (ISU) was established. Three quantitative and normalized indicators were designed to describe the magnitude of ISU. With the case study of the upper Daqing River watershed, China and the model SWAT (Soil and Water Assessment Tool), one ideal dataset without quality flaws and 79 datasets with different types of flaws including observation error, low monitoring frequency, short data duration and low data resolution were evaluated. The result showed that 4 of 13 parameters that control canopy, groundwater and channel processes have higher ISU values, indicating the high identifiability, high sensitivity, and low uncertainty. The largest gap of parameter ISU between dataset with quality flaw and ideal dataset was 0.61 due to short data duration, while the smallest gap was −0.28 due to low monitoring data frequency. Although some defective datasets caused unacceptable calibration results and model output, some defective datasets can still be valuable for model calibration which depends on the hydrological processes of interest when applying the model. Equivalent calibration results were yielded by the datasets with similar statistical properties. When using datasets with traditional defective issues for calibration, a new step checking the consistency among decision goal, representative system process, determinative parameters and calibration datasets is suggested. Practices including process-related data selection, dataset regrouping and risk self-reporting when using low-quality datasets are encouraged to increase the reliability of model-based watershed management.
更多查看译文
关键词
watershed modeling,parameter calibration,datasets,low-quality
AI 理解论文
溯源树
样例
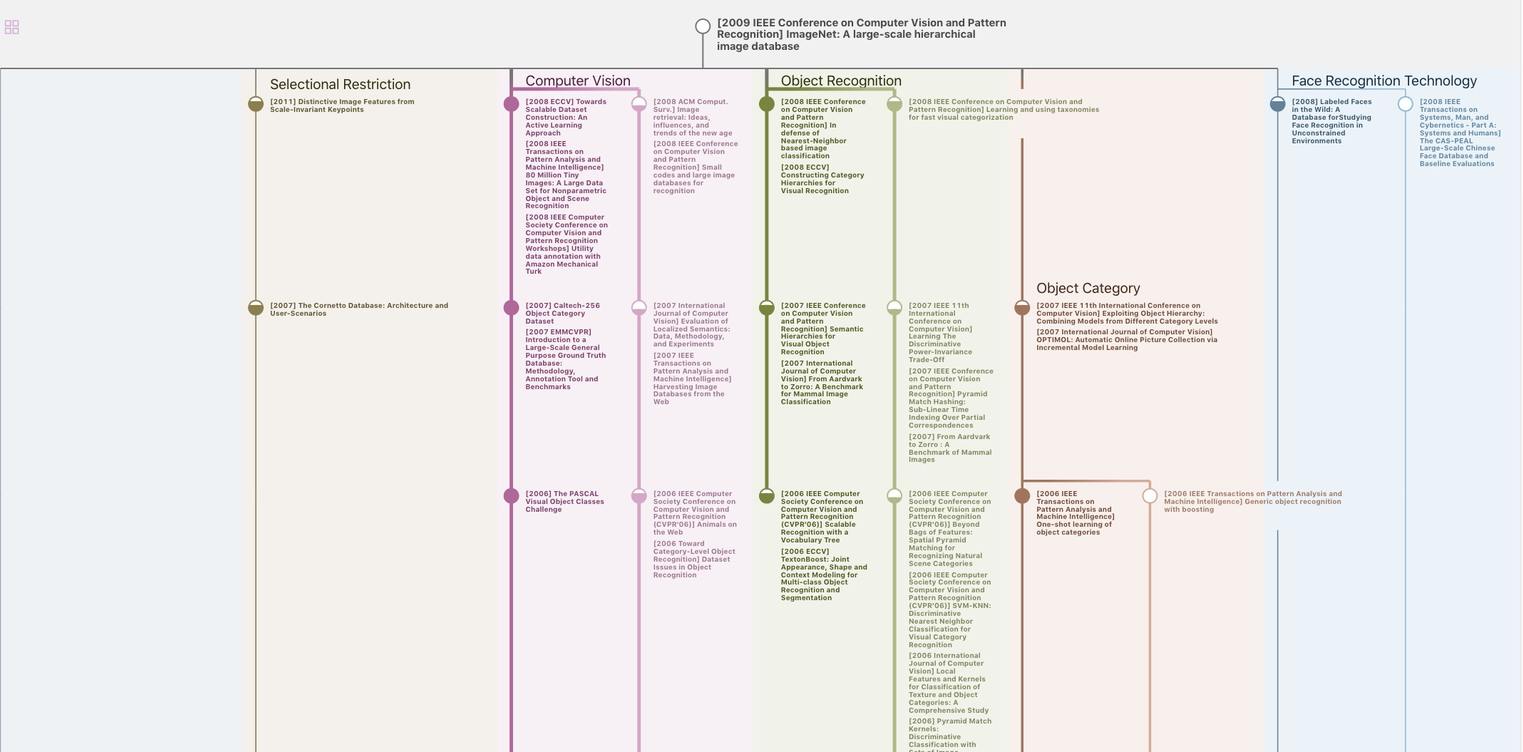
生成溯源树,研究论文发展脉络
Chat Paper
正在生成论文摘要