Caution Is Required When Clinically Implementing AI Models: What the COVID-19 Pandemic Taught Us About Regulation and Validation
HPHR Journal(2021)
Abstract
The novelty of COVID 19 ushered an expansion of artificial intelligence models designed to close clinical knowledge gaps, especially with regard to diagnosis and prognostication. These models emerged within a unique regulatory context that largely defers governance of clinical decision support tools. As such, we raise three concerns about the implementation of clinical artificial intelligence models, using COVID-19 as an important case study. First, flawed data underlying model development leads to flawed clinical resources. Second, models developed within one focus of geographic space and time leads to challenges in generalizability between clinical environments. Third, failure to implement ongoing monitoring locally leads to diminishing utility as diseases and implicated populations inevitably change. Experience with this pandemic has informed our assertion that machine learning models should be robustly vetted by facilities using local data to ensure that emerging technology does patients more good than harm.
MoreTranslated text
Key words
ai models,validation,pandemic
AI Read Science
Must-Reading Tree
Example
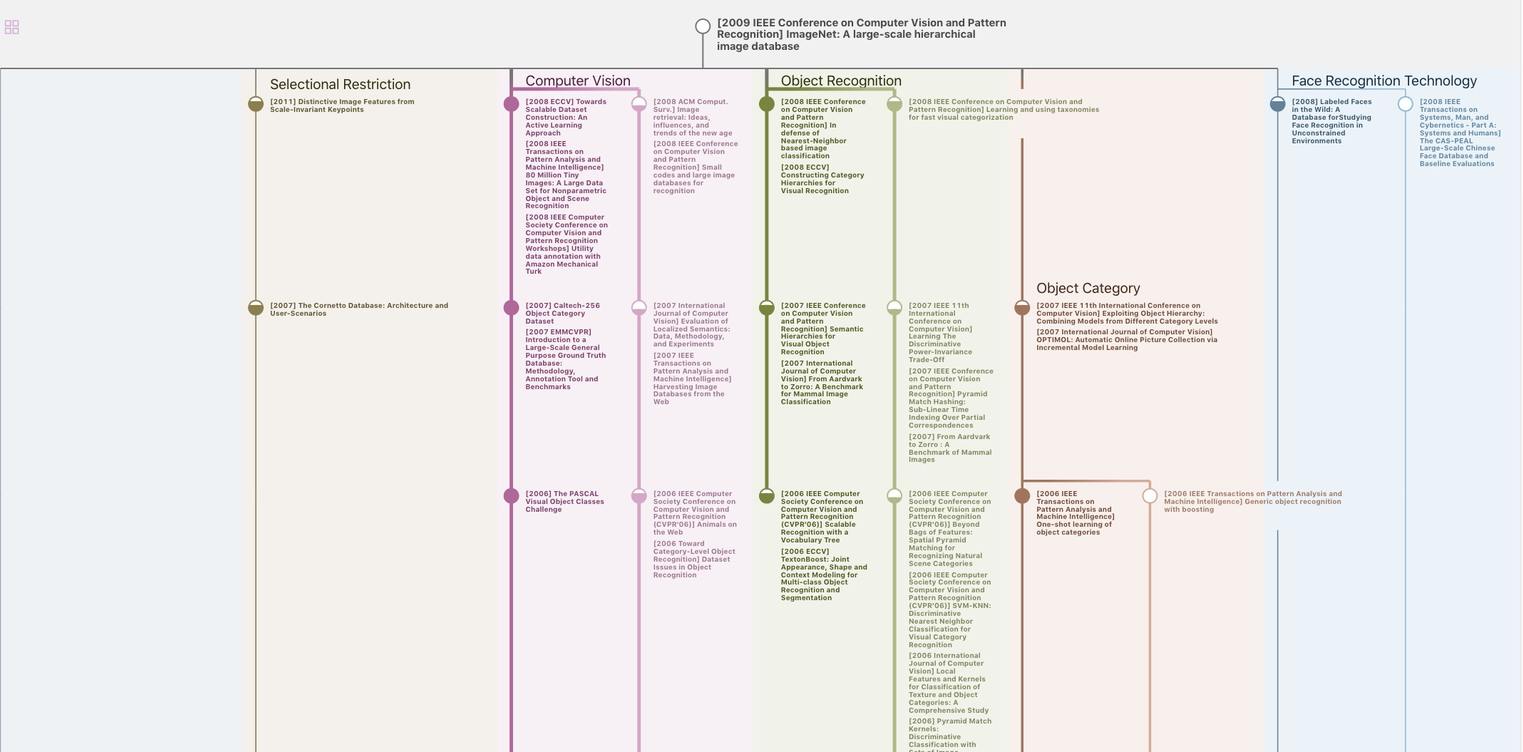
Generate MRT to find the research sequence of this paper
Chat Paper
Summary is being generated by the instructions you defined