A 28nm 27.5TOPS/W Approximate-Computing-Based Transformer Processor with Asymptotic Sparsity Speculating and Out-of-Order Computing.
International Solid-State Circuits Conference(2022)
摘要
Recently, Transformer-based models have achieved tremendous success in many AI fields, from NLP to CV, using the attention mechanism [1]–[3]. This mechanism captures the global correlations of input by indicating every two tokens' relevance with attention scores and uses normalized scores, defined as attention probabilities, to weight all input tokens to obtain output tokens with a global receptive field. A Transformer model consists of multiple blocks, named multi-head, working with the attention mechanism. Figure 29.2.1 details the computation of an attention block with query (Q), key (K), and value-matrix (V), computed by tokens and weight matrices. First, Q is multiplied by KT to generate the attention score matrix. The scores in each row, represented as
$\mathrm{X}_{\mathrm i}$
, indicate a token's relevance with all others. Second, the row-wise softmax with inputs of
$\mathrm{X}_{\mathrm{i}}-\mathrm{X}_{\max}$
normalizes attention scores to probabilities (P), expanding the large scores and reducing the small scores exponentially. Finally, probabilities are quantized and then multiplied by V to produce the output. Each output token is a weighted sum of all input tokens, where the strongly related tokens have large weight values. Global attention-based models achieve 20.4% higher accuracy than LSTM for NLP and 15.1% higher accuracy than ResNet-152 for classification.
更多查看译文
关键词
out-of-order computing,transformer-based models,tremendous success,AI fields,NLP,attention mechanism,attention scores,normalized scores,attention probabilities,input tokens,output token,global receptive field,multiple blocks,named multihead,attention block,weight matrices,attention score matrix,weighted sum,strongly related tokens,weight values,global attention-based models,asymptotic sparsity speculation,approximate-computing-based transformer processor,size 28.0 nm
AI 理解论文
溯源树
样例
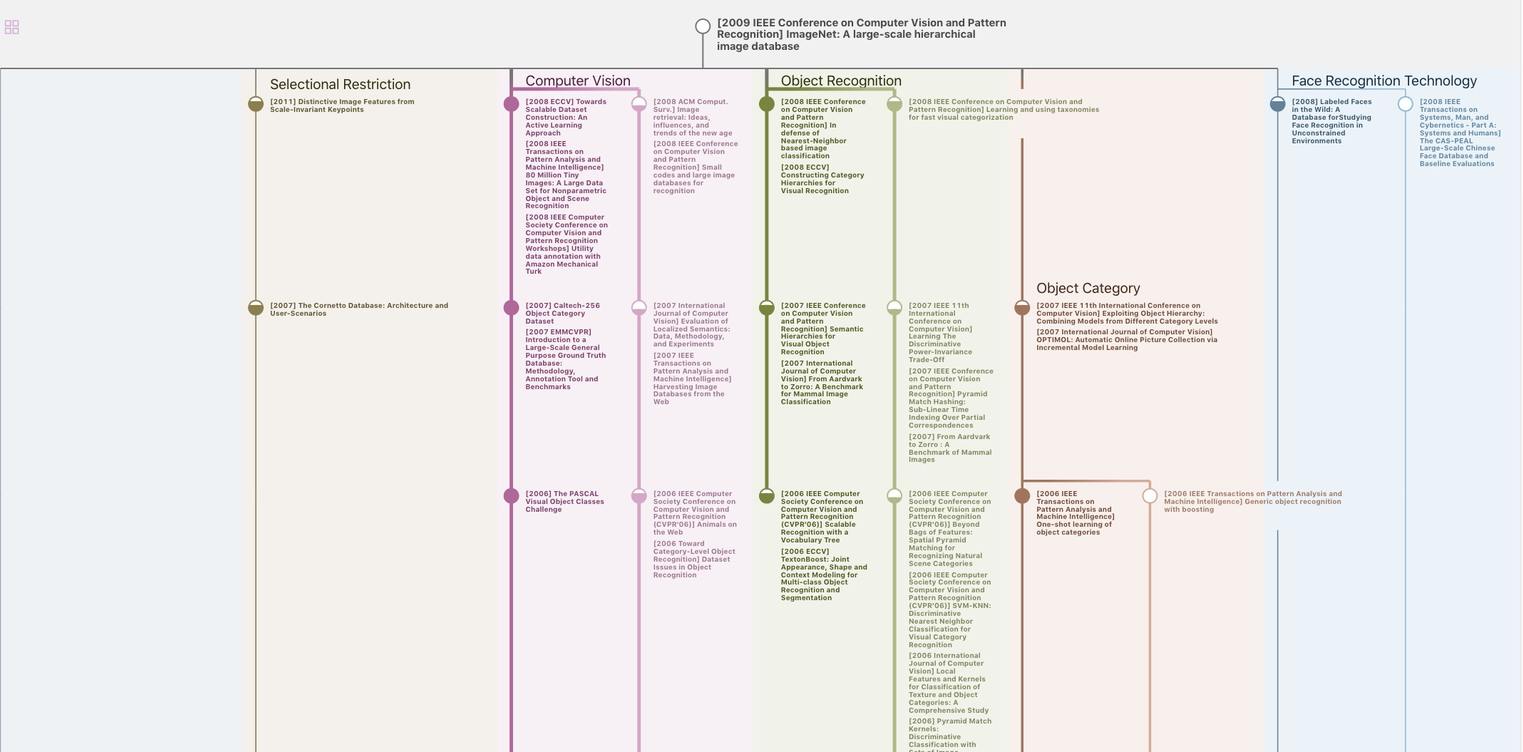
生成溯源树,研究论文发展脉络
Chat Paper
正在生成论文摘要